Uncertainty-aware fatigue-life prediction of additively manufactured Hastelloy X superalloy using a physics-informed probabilistic neural network
RELIABILITY ENGINEERING & SYSTEM SAFETY(2024)
摘要
Microstructural inhomogeneity in additively manufactured (AM) components leads to uncertainty in their fatigue performance. While purely data-driven methods can only provide deterministic outcomes and lack physical interpretability. Furthermore, considering the dispersion of fatigue life, a probabilistic neural network framework integrating physical information, namely a physics-informed probabilistic neural network (PIPNN), is proposed for predicting the fatigue life of AM parts. The framework describes the dispersion of fatigue life in the parametric form of probability statistics. It incorporates physical laws and models to constrain neurons and loss function, enabling the network to learn deeper physical laws that align with the fatigue process, thus enhancing the interpretability and prediction reliability of the model. Fatigue experiments were performed on Hastelloy X superalloy specimens fabricated using laser powder bed fusion, serving as the basis for validating and comparing the PIPNN model with a probabilistic neural network. The results indicate that PIPNN adeptly captures the heteroskedasticity of fatigue life and exhibits superior prediction accuracy and more reliable prediction performance in fatigue-life prediction. PIPNN offers a physically consistent method for fatigue-life prediction considering probabilistic statistics.
更多查看译文
关键词
Fatigue-life prediction,Probabilistic neural network,Physical information,Additive manufacturing,Superalloy
AI 理解论文
溯源树
样例
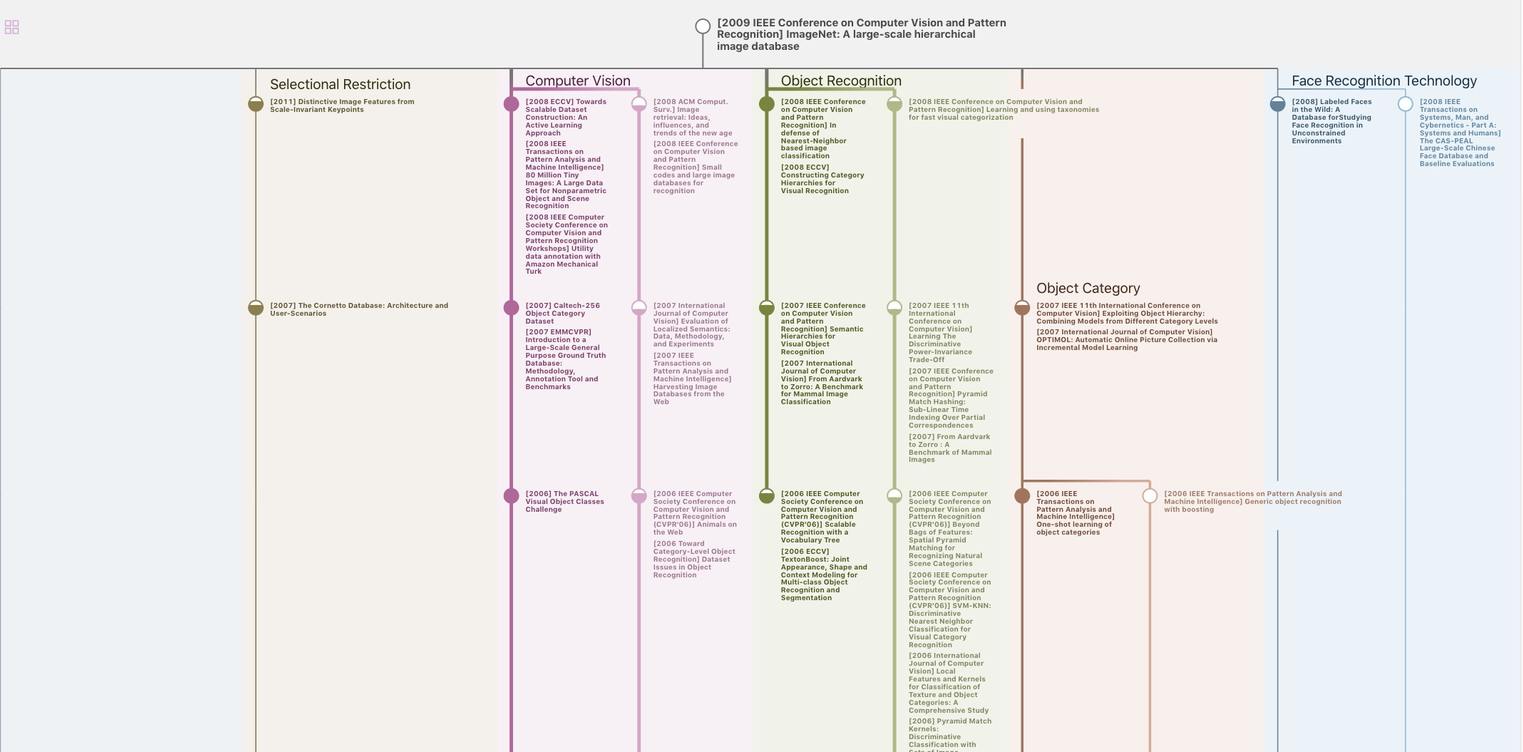
生成溯源树,研究论文发展脉络
Chat Paper
正在生成论文摘要