Gooseneck barnacle optimization algorithm: A novel nature inspired optimization theory and application
MATHEMATICS AND COMPUTERS IN SIMULATION(2024)
摘要
This paper introduces the Gooseneck Barnacle Optimisation Algorithm (GBO) as a novel evolutionary method inspired by the natural mating behaviour of gooseneck barnacles, which involves sperm casting and self-fertilization. GBO is mathematically modelled, considering the hermaphroditic nature of these microorganisms that have thrived since the Jurassic period. In contrast to the previously published Barnacle Mating Optimizer (BMO) algorithm, GBO more accurately captures the unique static and dynamic mating behaviours specific to gooseneck barnacles. The algorithm incorporates essential factors, such as navigational sperm casting properties, food availability, food attractiveness, wind direction, and intertidal zone wave movement during mating, creating two vital optimization stages: exploration and exploitation. Real-world case studies and mathematical test functions serve as qualitative and quantitative benchmarks. The results demonstrate that GBO outperforms well-known algorithms, including the previous BMO, by effectively improving the initial random population for a given problem, converging to the global optimum, and producing significantly better optimization outcomes.
更多查看译文
关键词
Optimization,Evolutionary algorithm,Meta-heuristic,Constrained optimization,Benchmark,Covid-19 confirmed cases
AI 理解论文
溯源树
样例
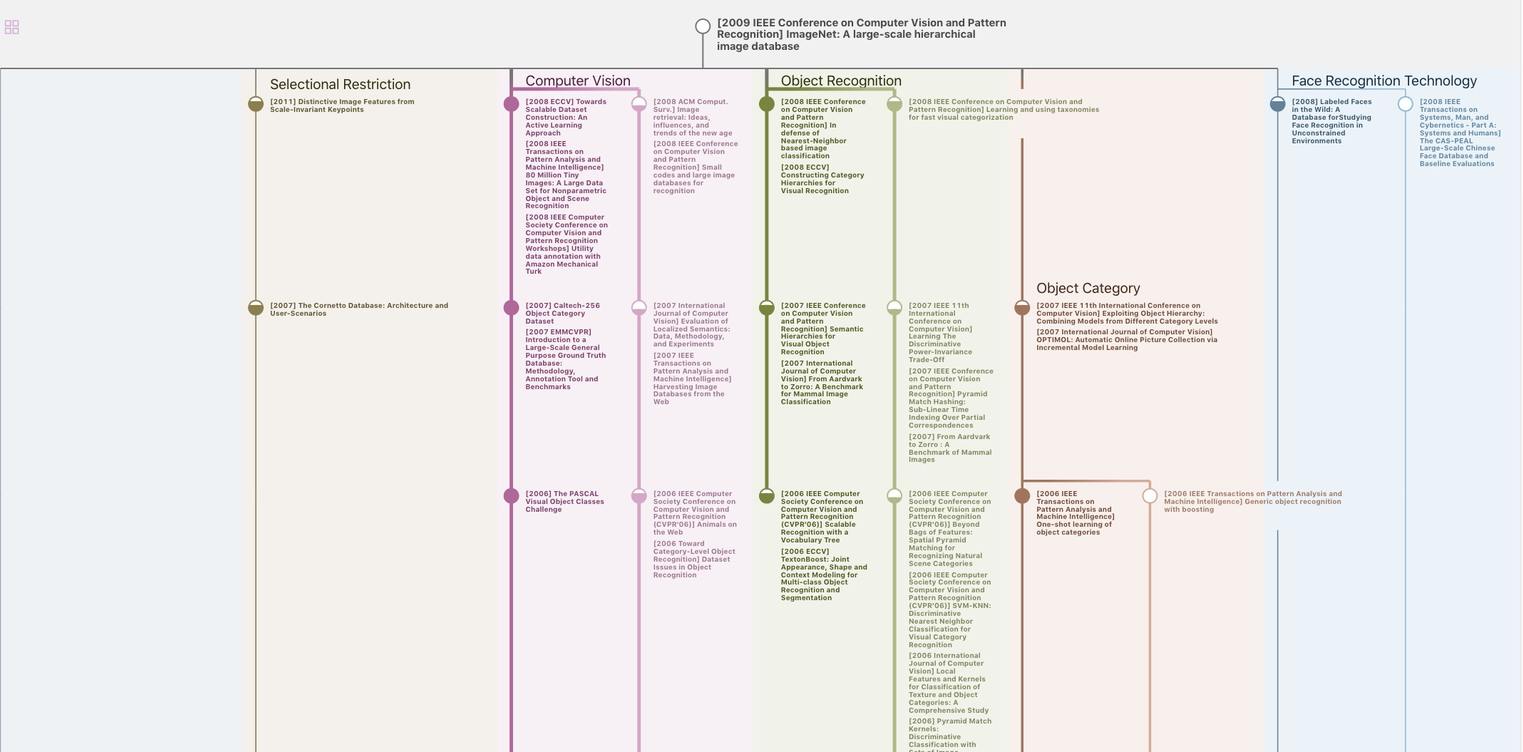
生成溯源树,研究论文发展脉络
Chat Paper
正在生成论文摘要