Data-driven prediction and optimization of axial compressive strength for FRP-reinforced CFST columns using synthetic data augmentation
ENGINEERING STRUCTURES(2024)
摘要
Fiber-reinforced polymer (FRP) sheets can be used as additional confinement to improve the load-bearing capacity and durability of concrete-filled steel tubular (CFST) columns. This study presents a data-driven approach aimed at accurately predicting the strength and optimizing the design of FRP-CFST columns under axial compressive loading. To overcome the limited database size (only 287 samples), a synthetic data augmentation technique was developed with recourse to the tabular generative adversarial networks (TGAN). Four machine learning (ML) models were rigorously trained using two distinct schemes "Trained with synthetic - Tested with real" (TSTR) and "Trained with real - Tested with real" (TRTR) to seek out the best strength prediction model. Then the winner model was integrated with the metaheuristic optimization algorithm-nondominated sorting genetic algorithm II (NSGA-II), to optimize both the axial strength and material cost of FRP-CFST columns. This fusion enabled a dual-objective optimization via generating the Pareto front, providing designers with a spectrum of optimal design solutions. It turned out that the categorical gradient boosting model trained with the TSTR mode (CATB-Syn) showed superior performance than the other ML models. Its accuracy also surpassed that of existing design equations. The combination of CATB-Syn with NSGA-II provided a reliable Pareto front, and the interpretability of CATB-Syn was also verified. Thus, the proposed framework opens a new avenue for the efficient design of such double-jacketed columns.
更多查看译文
关键词
CFST columns,FRP,Axial strength,Data augmentation,Machine learning,Optimization design
AI 理解论文
溯源树
样例
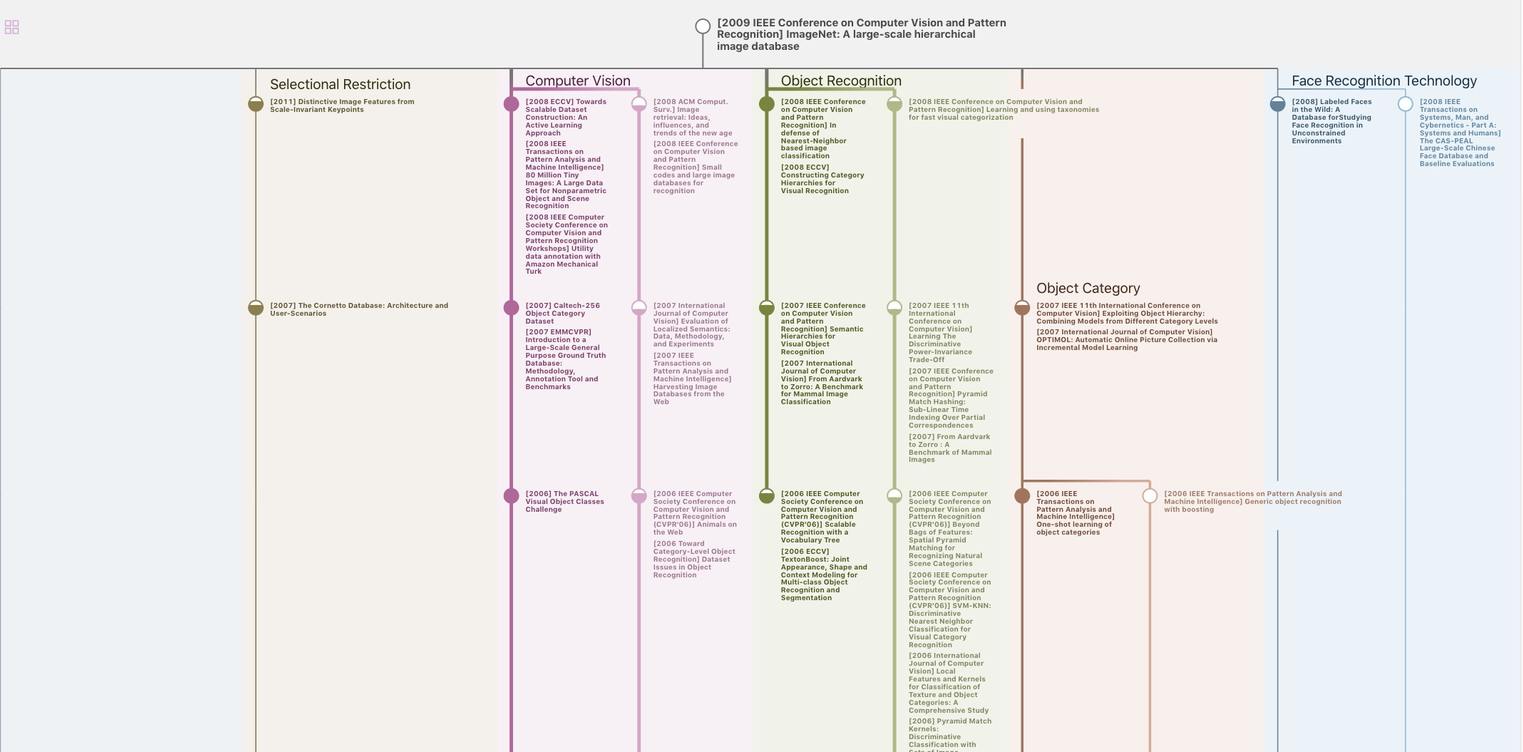
生成溯源树,研究论文发展脉络
Chat Paper
正在生成论文摘要