Contrasting augmented features for domain adaptation with limited target domain data
PATTERN RECOGNITION(2024)
摘要
Domain adaptation aims to alleviate distribution gaps between source and target domains. However, when the available target domain data are scarce for training, learning generalizable representations for domain adaptation is challenging. We propose a novel approach, dubbed Contrasting Augmented Features (CAF), to tackle the challenge of insufficient target domain data for domain adaptation, by generating and contrasting augmented features. We introduce a semantic feature generator to generate augmented features by replacing the instance-level feature statistics of one domain with another domain. With the augmented features, we further design the reweighted instance contrastive loss and category contrastive loss to improve feature discrimination and align feature distributions of source and target domains. CAF can be applied to few-shot domain adaptation and unsupervised domain adaptation with limited unlabeled target domain data. Despite its simplicity, extensive experiments show promising results for both applications. In addition, experiments demonstrate that CAF is more robust to the number of target domain data and also effective in vanilla unsupervised domain adaptation setting with full target domain data.
更多查看译文
关键词
Domain adaptation,Limited target domain data,Contrasting augmented features
AI 理解论文
溯源树
样例
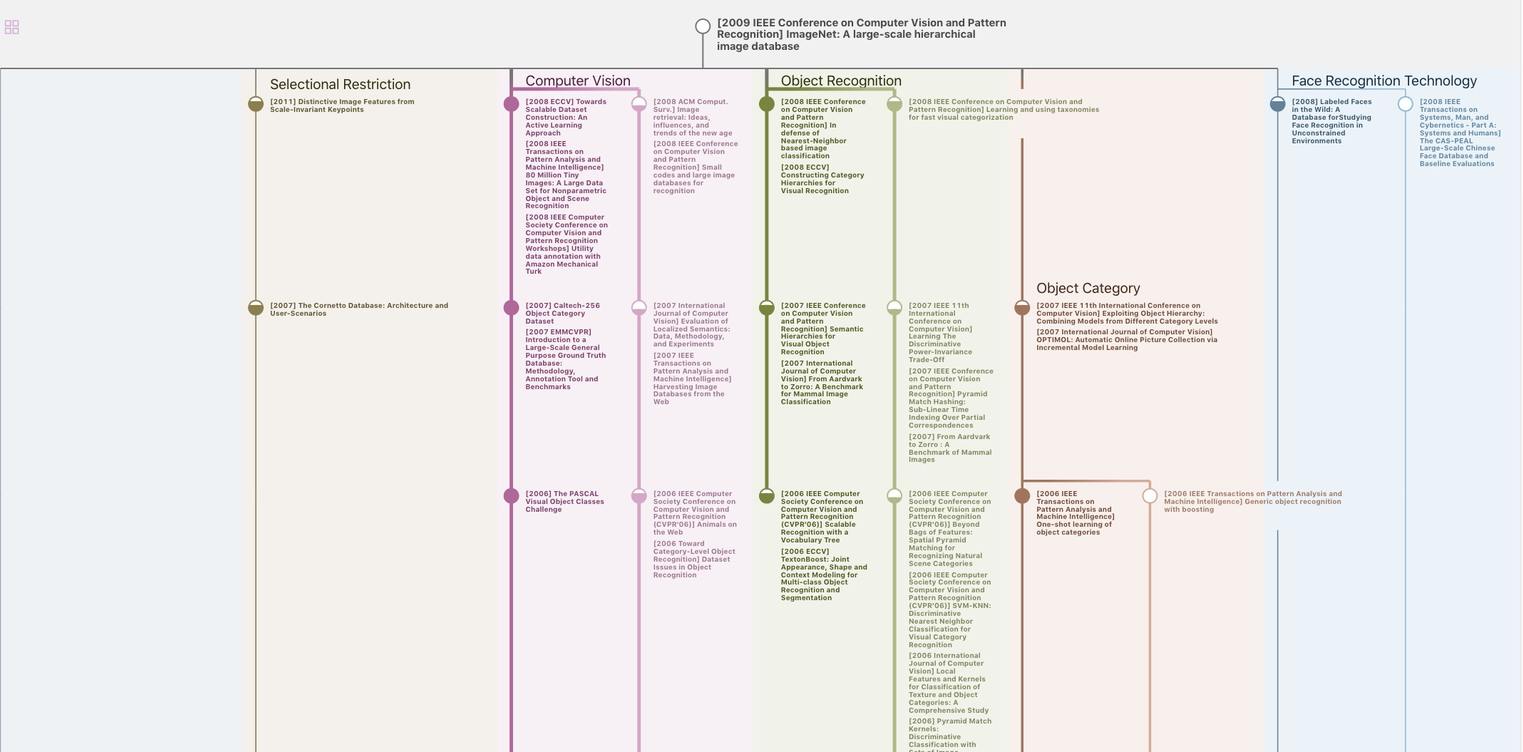
生成溯源树,研究论文发展脉络
Chat Paper
正在生成论文摘要