A communication-less islanding detection scheme for hybrid distributed generation systems using recurrent neural network
INTERNATIONAL JOURNAL OF ELECTRICAL POWER & ENERGY SYSTEMS(2024)
摘要
The proposed scheme in this research paper is a communication-less islanding detection system based on recurrent neural network (RNN) for hybrid distributed generator (DG) systems that include both synchronous and inverter-based DG devices. The scheme consists of three stages: time-domain feature extraction (FE) from the three-phase voltage signal at the point of common coupling (PCC), feature selection using the wrapper method, and detection using a long short-term memory (LSTM) RNN algorithm. To assess the performance of the proposed scheme, a comprehensive set of islanding and grid-connected scenarios was created using the IEC 61850-7420 test system in MATLAB/Simulink. After feature extraction in MATLAB, the feature selection and LSTM model training/testing steps were conducted in Python. Thirteen features were extracted from the voltage signal, and the most significant features were selected for detection using the wrapper method. The detection process encompassed various events, such as line faults, load switching, and capacitor switching, occurring in both islanded and grid-connected modes. The proposed scheme was compared against existing techniques, artificial neural networks, and support vector machines under the same conditions. The proposed scheme demonstrated excellent accuracy (99.68 %), selectivity (99.44 %), and sensitivity (100 %) under normal conditions with all features. It also performed well in noisy environments, with accuracies of 98.94 % and 97.04 % with 40 dB and 30 dB noise, respectively. This research highlights the importance of quality and quantity of data for training intelligent RNN-based IDSs and demonstrates the effectiveness of the proposed scheme in detecting islanding in hybrid DG systems.
更多查看译文
关键词
Islanding detection,Hybrid power systems,Recurrent neural network,Long short-term memory,Distributed generation,Feature selection
AI 理解论文
溯源树
样例
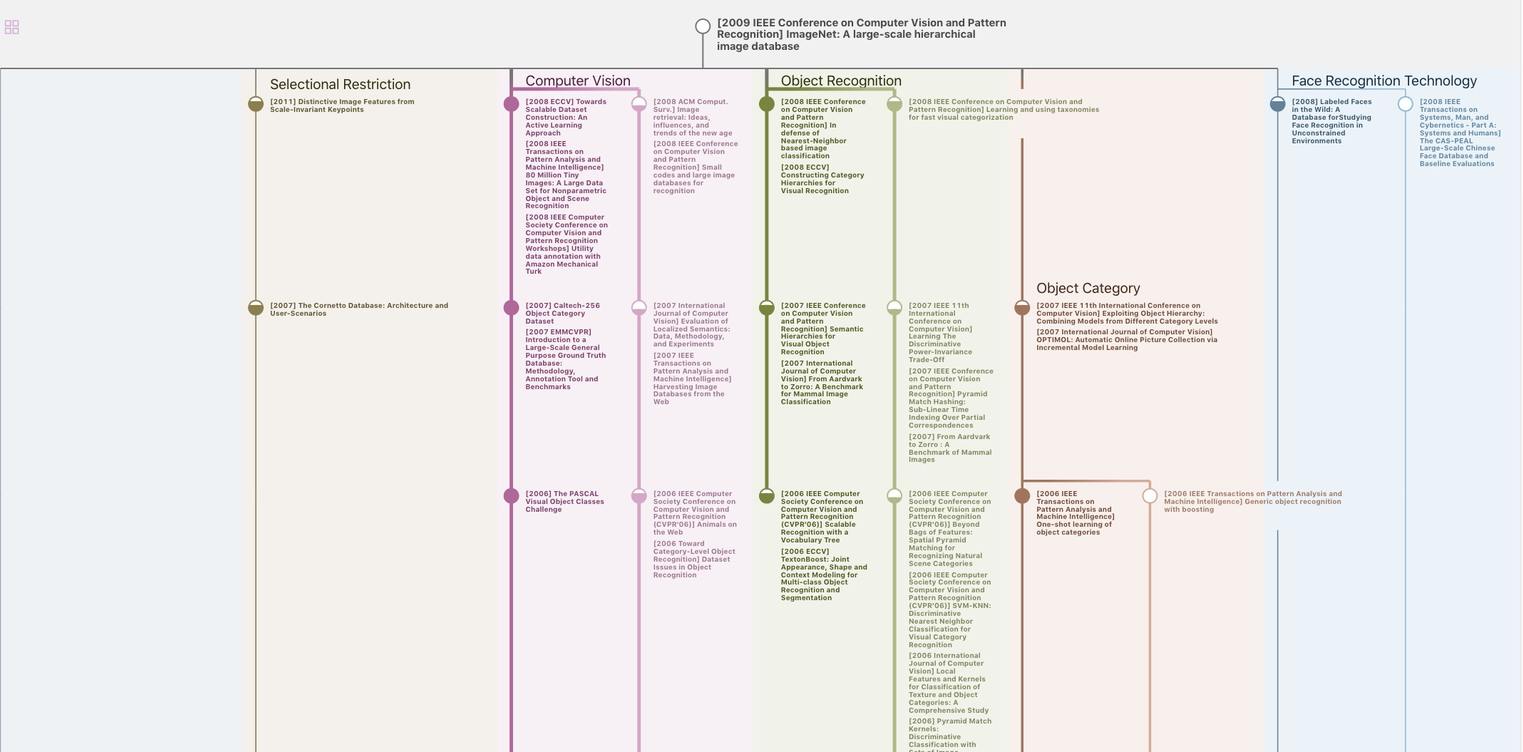
生成溯源树,研究论文发展脉络
Chat Paper
正在生成论文摘要