Automatic recognition of defects behind railway tunnel linings in GPR images using transfer learning
MEASUREMENT(2024)
摘要
Ground-penetrating radar (GPR) is widely used for non-destructive detection of hidden defects behind railway tunnel linings, including non-compactness, air- and water-filled voids, fractures, and insufficient lining thickness. However, manual interpreting a substantial volume of GPR B-scan images is time-consuming, and the results heavily depend on the professional knowledge of the practitioner and the available prior information. This paper presents an automatic recognition algorithm for detecting hidden defects, as well as estimating lining thickness in GPR B-scan images of railway tunnels using a You Only Look Once for Panoptic driving perception network (YOLOP) model. The process mainly contains three steps. Firstly, a real GPR dataset of underground objects is employed for transfer learning to enhance the robustness of the prediction model. Secondly, a dataset containing 2,245 real GPR B-scan images of railway tunnel linings is created. Thirdly, the established dataset is used to retrain the YOLOP model and assess the prediction accuracy for identifying hidden defects and lining thickness in GPR images. Field experiment results demonstrate that the trained hidden defects recognition algorithm achieves an average accuracy of 80.6%, with a detection time of only 0.02 s for a GPR image sizing of 1024 x 512. Therefore, it is concluded that the proposed algorithm is efficient and reliable for automatic recognition of hidden defects behind railway tunnel linings.
更多查看译文
关键词
Non-destructive testing (NDT),Ground penetrating radar (GPR),Railway tunnel,Deep learning,Hidden defects
AI 理解论文
溯源树
样例
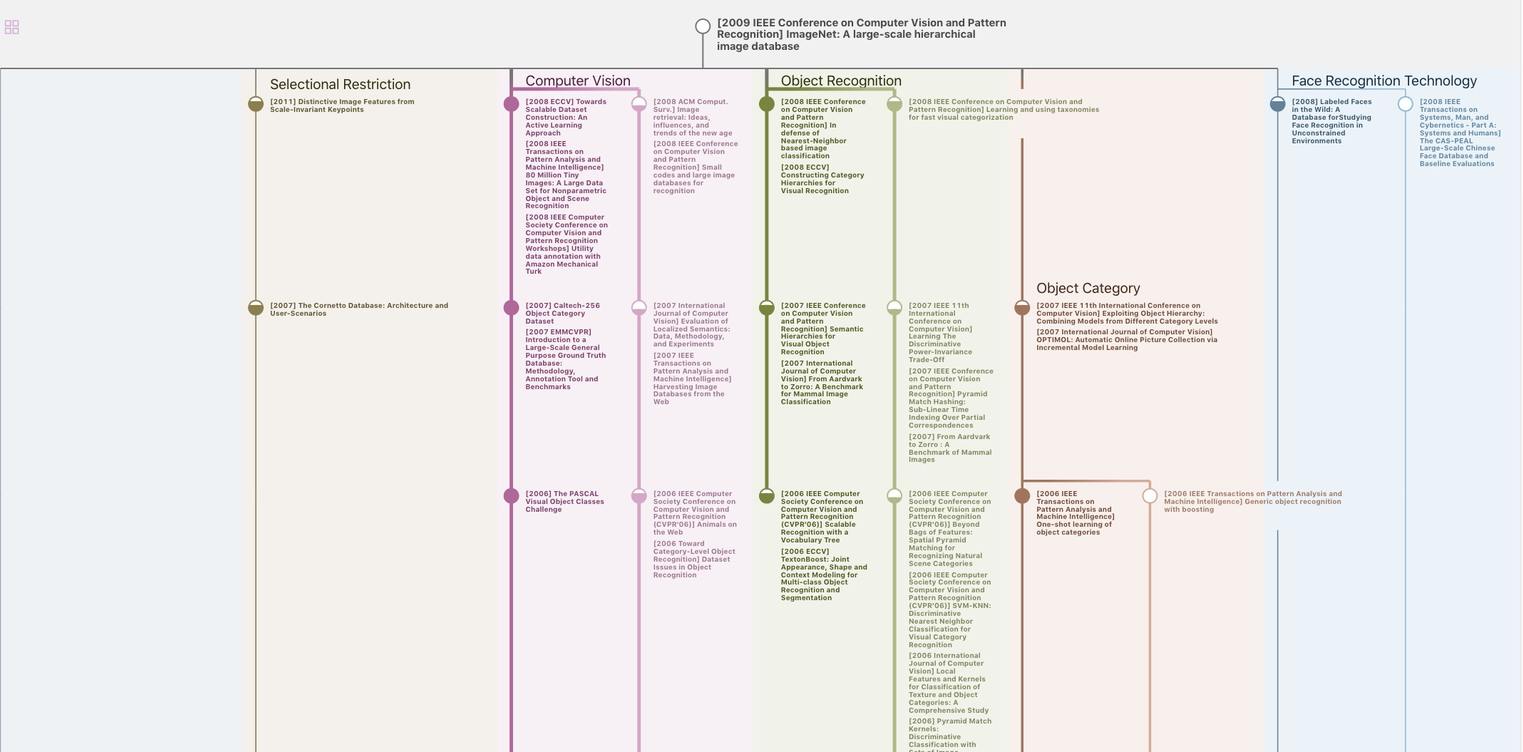
生成溯源树,研究论文发展脉络
Chat Paper
正在生成论文摘要