ChatGPT-based biological and psychological data imputation
Meta-Radiology(2023)
摘要
Missing data are a common problem for large cohort or longitudinal research and have been handled through data imputation. Based on simplified models such as linear or nonlinear interpolations, current imputation methods may not be accurate for real-life data such as biological and behavioral data. The purpose of this work was to explore the capability of ChatGPT, a powerful Large Language Model (LLM) developed by OpenAI, for biological and psychological data imputation. We tested the feasibility using data from the Human Connectome Project. Performance was evaluated by comparing the imputed data against known ground truth (GT) and measured with metrics like Pearson correlation coefficient (r), relative accuracy (MP), and mean absolute error (MAE). Comparative analyses with traditional imputation techniques are also conducted to demonstrate the superior efficacy of the ChatGPT as a data imputer. In summary, through customized data-to-text prompting engineering, ChatGPT can successfully capture intricate patterns and dependencies within biological data, resulting in precise imputations. Fine-tuning ChatGPT with domain-specific biological vocabulary with human in-loop as an interpreter enhances the accuracy and relevance of the imputations.
更多查看译文
关键词
data,biological,chatgpt-based
AI 理解论文
溯源树
样例
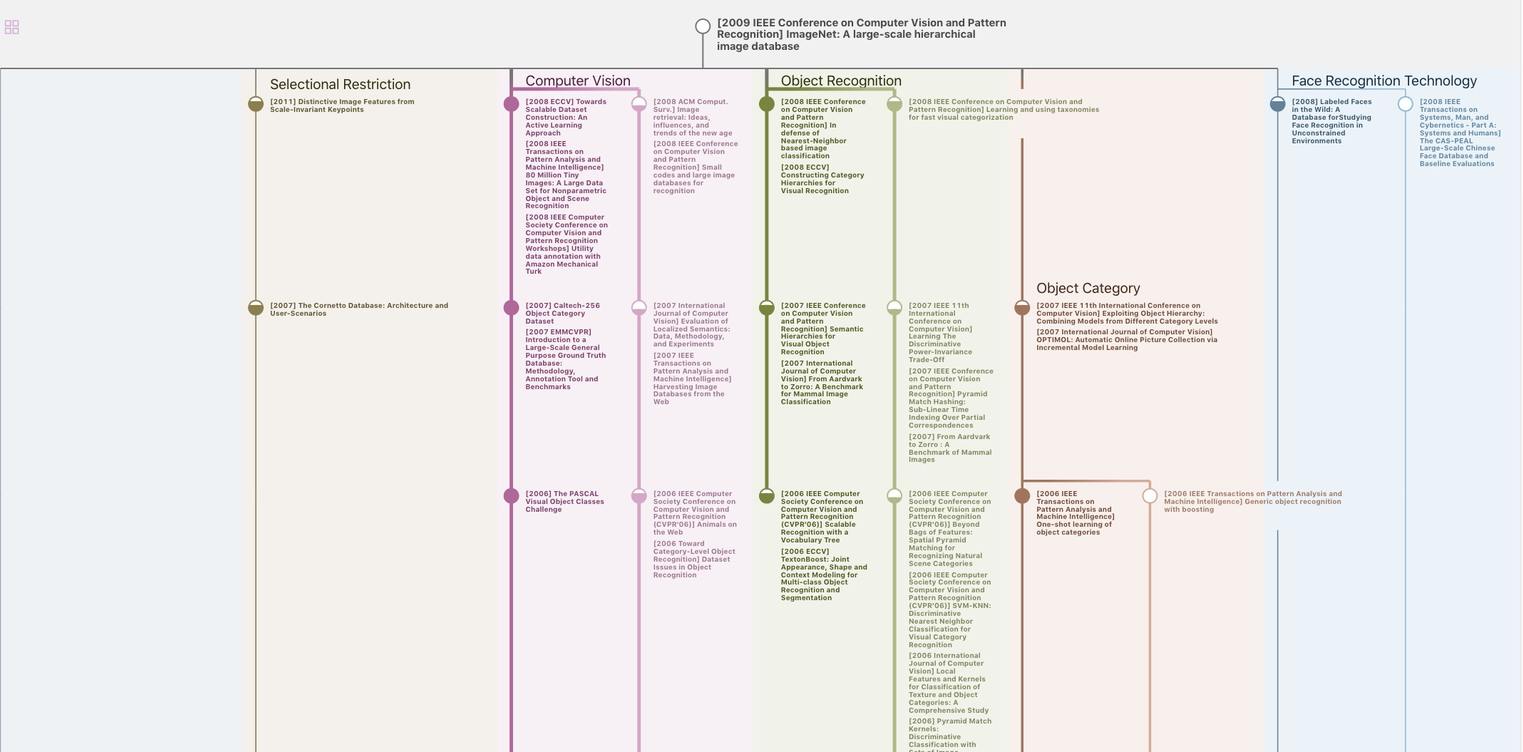
生成溯源树,研究论文发展脉络
Chat Paper
正在生成论文摘要