Implicit IoT authentication using on-phone ANN models and breathing data
INTERNET OF THINGS(2023)
摘要
The expansionist nature of the Internet of Things (IoT) with smart sensing has integrated smart technology into the fabric of daily life. From digital health check-ups and personal property access to financial transactions are conveniently performed with the help of smart technologies, such as smart wearables. Therefore, the security of these devices and the entire IoT-connected cyber-physical space accessible through smart wearables has become a burning question. While the current knowledge-based security approaches (e.g., pattern locks, passwords, or PINs) cause a recall burden to the user, conventional one-time biometric-based methods are not adaptable to the tiny wearables. Therefore, this work presents an implicit IoT authentication utilizing breathing patterns to verify a user through continuous microphone sensing. The core piece of this work is the Breathing data-driven TensorFlow Lite framework-supported on-phone authentication application (i.e., BTL Auth app), which consists of an audio processing pipeline (to perform filtering and feature computation) and an artificial neural network model to verify the target user. From our detailed analysis of sequential and non-sequential neural networks trained with static and dynamic audio features, we can achieve an average accuracy of up to .93 when authenticating a user from breathing patterns. Finally, the BTL Auth app needs around 64 KB of memory and 5 s to verify the user.
更多查看译文
关键词
Artificial neural networks,Breathing patterns,Continuous authentication,Implicit authentication,Microphone sensing,Audio analytics
AI 理解论文
溯源树
样例
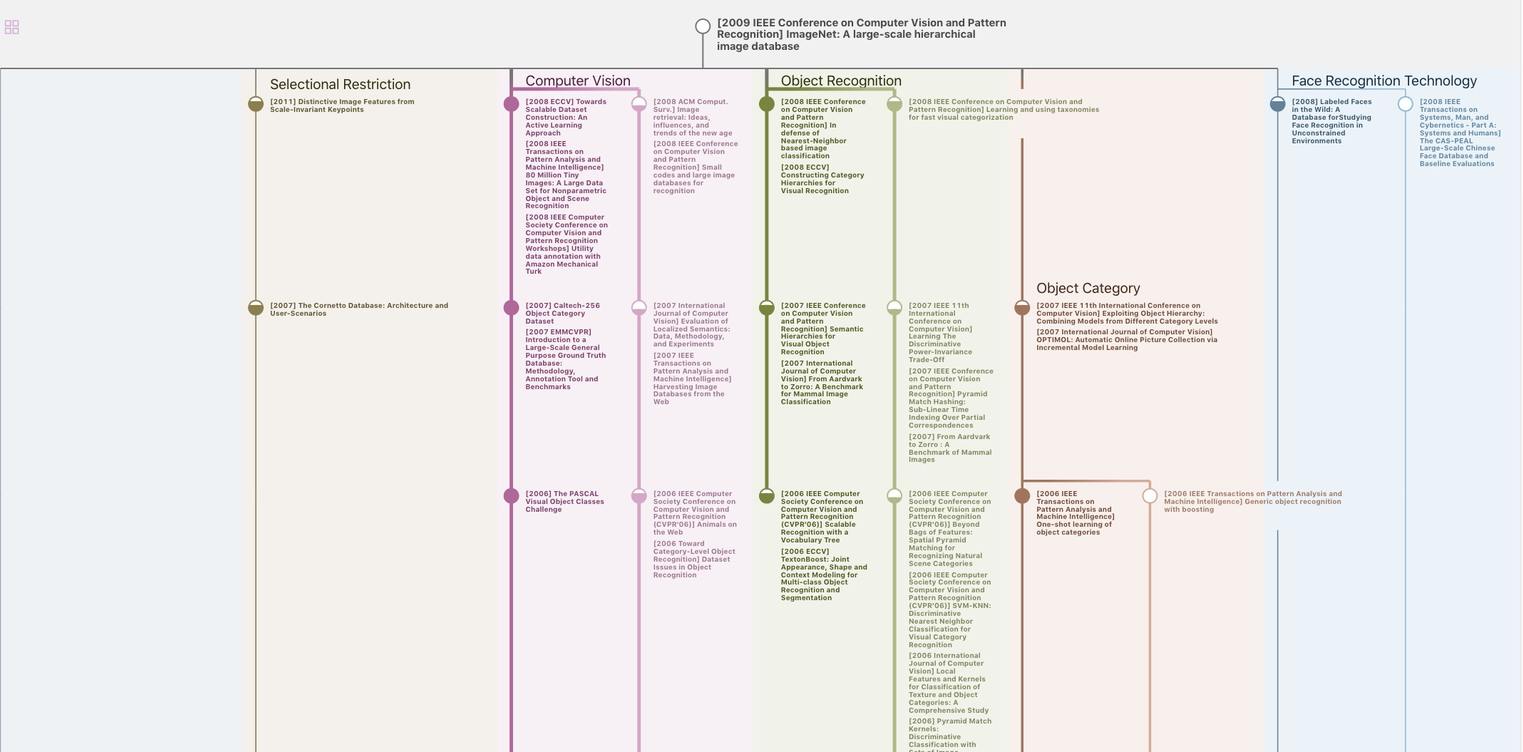
生成溯源树,研究论文发展脉络
Chat Paper
正在生成论文摘要