Cooperative behavior of a heterogeneous robot team for planetary exploration using deep reinforcement learning
ACTA ASTRONAUTICA(2024)
摘要
As we continue exploration of the Lunar and Martian surfaces and push farther into unknown and unstructured environments, employing a team of distributed heterogeneous robots will increase the odds of success by enabling more complex task planning that utilizes the complementary capabilities and synergy of the team members. A requirement to reap these potential benefits is effective cooperation and collaboration between the members of a robot team. Defining a metric for effective cooperation is a difficult task that will depend on the composition of the team, the task to be performed, and the working environment. This paper establishes a method for determining the reward criteria (figures of merit) that can be used for training the robot swarm through reinforcement learning techniques. A hierarchical framework of rewards is used which, at the lowest level, measures the success of an individual robot in performing its task. The success of all robots performing different subtasks is then measured using the Quantified Cooperation Assessment (QCA) metric which was introduced in our previous research of multi-robot collaboration. Finally, the mission-level success and overall reward is determined by weighting each task using its priority within the overall mission context. A common reward for each of the robotic teammates is then applied within the learning process, which emphasizes group performance over that of an individual and encourages cooperative behavior. This cooperation framework is trained in a grid-based environment representing an exploration mission on a planetary surface by a heterogenous team of robots consisting of a landing craft, a traditional rover, and several small agile robots. The robots are trained concurrently, but with individual policies developed for each agent resulting in a decentralized control scheme. Once trained, the control policies were evaluated in several test environments consisting of novel terrain maps and regions of interest. An average success rate of 90 % was seen in the test environments demonstrating the robustness of the trained policies. The robots have been trained to not only complete the mission but also perform it in a cooperative manner as well as show the scalable and resilient behavior.
更多查看译文
关键词
Robot swarm,Heterogeneous robot team,Multi-robot cooperation,Planetary exploration,Reinforcement learning,Team behavior,Decentralized multi-agent control
AI 理解论文
溯源树
样例
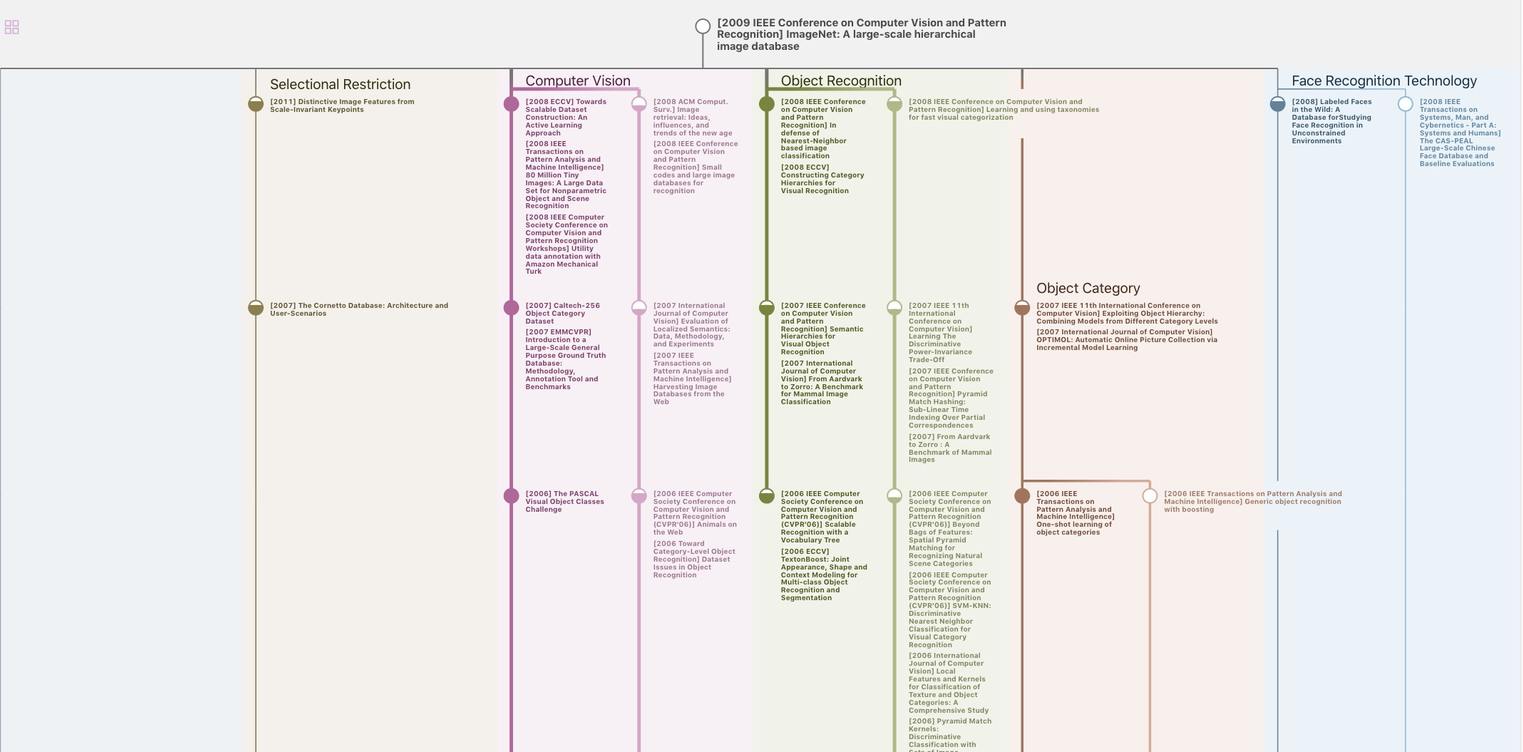
生成溯源树,研究论文发展脉络
Chat Paper
正在生成论文摘要