Scale-pyramid dynamic atrous convolution for pixel-level labeling
EXPERT SYSTEMS WITH APPLICATIONS(2024)
摘要
For achieving better performance, the majority of deep convolutional neural networks have endeavored to increase the model capacity by adding more convolutional layers or increasing the size of the filters. Consequently, the computational cost increases proportionally with the model capacity. This problem can be alleviated by dynamic convolution. In the case of pixel-level labeling, existing pixel-level dynamic convolution methods have a smaller scanning area than ordinary convolution or image-level dynamic convolution and are thus unable to exploit fine contextual information. As a consequence, pixel-level dynamic convolution is more sensitive to large-scale varying objects and confusion categories. In this paper, we propose a scale-pyramid dynamic atrous convolution (SDAConv) and exploit multi-scale pixel-level features in finer granularity, in order to efficiently increase model capacity, exploring contextual information, capture detail information and alleviate large-scale variation problem at the same time. Through kernel engineering (instead of network engineering), SDAConv dynamically arranges atrous filters in the individual convolutional kernels over different semantic areas at dense scales in the spatial dimension. By simply replacing the regular convolution with SDAConv in SOTA architectures, extensive experiments on three public datasets, Cityscapes, PASCAL VOC 2012 and ADE20K benchmarks demonstrate the superior performance of SDAConv on pixel-level labeling tasks.
更多查看译文
关键词
Pixel-level labeling,Deep learning,DCNN,Dynamic convolution,Kernel engineering
AI 理解论文
溯源树
样例
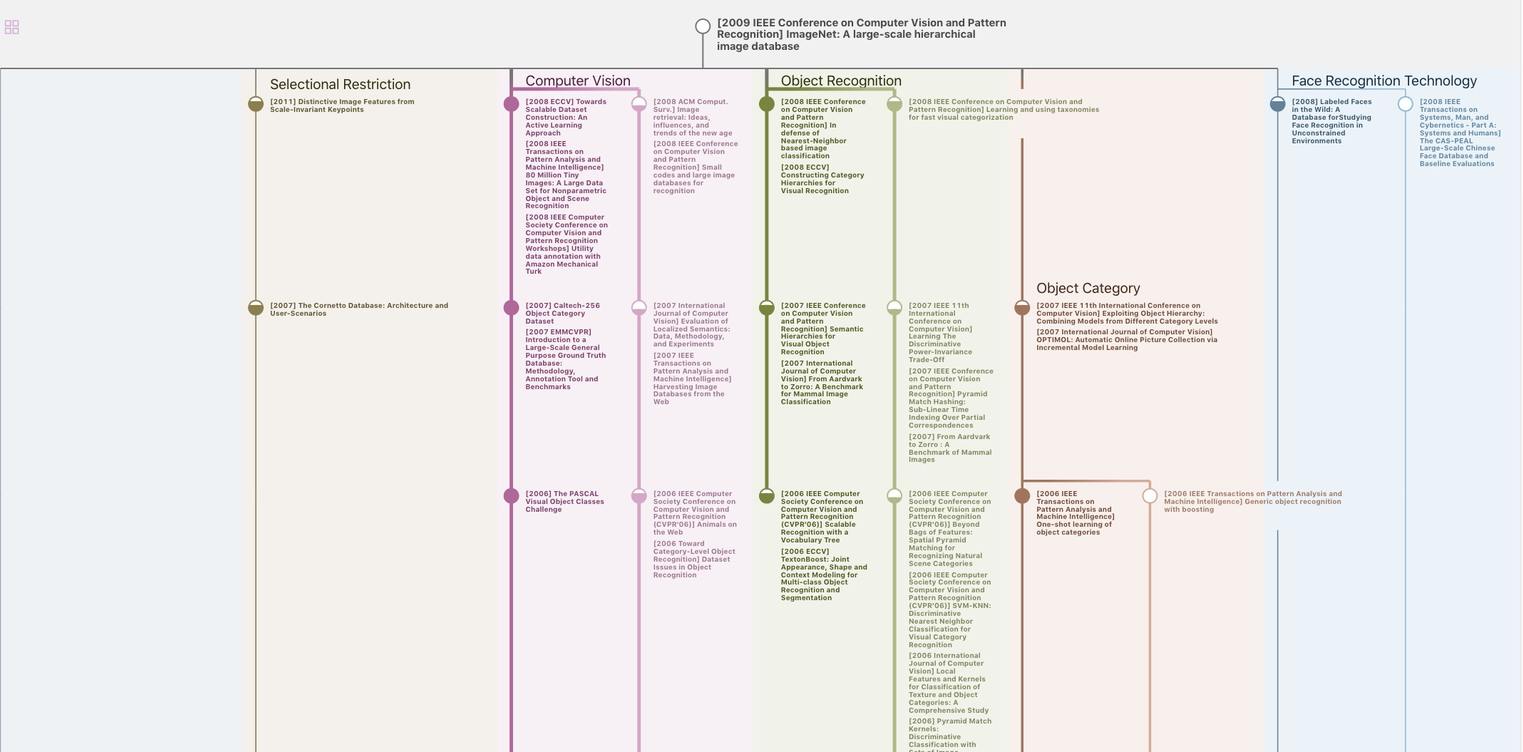
生成溯源树,研究论文发展脉络
Chat Paper
正在生成论文摘要