Dual states based reinforcement learning for fast MR scan and image reconstruction
NEUROCOMPUTING(2024)
摘要
Incomplete phase encoding with few phases is an effective under-sampling manner of fast Magnetic Resonance (MR) scan. The key is how to choose important slice-specific phases. Reinforcement Learning (RL) is powerful for sequential decision-making and therefore is feasible for slice-specific phase selection. Existing RL-based methods employ time-consuming reconstruction-oriented Deep Neural Networks (DNN) to generate/transit states from which phase selection and reward computation are performed. The advantage is that the selected phases and the corresponding partial k-space data match the DNN for image reconstruction. The disadvantage lies in the impossibility of deciding/selecting a phase in as short as several milliseconds required in the timings of a typical pulse sequence. To simultaneously keep the matching advantage and avoid the inefficiency disadvantage, we propose a dual-state-based RL framework. A visible Parameter-Free (PF) state obtained by inverse Fast Fourier transform and a hidden DNN state obtained by applying a time-consuming reconstruction oriented DNN on the visible state are called dual states. Visible states are used as input of phase decision networks and hidden states are used for computing reward to evaluate the decision networks. Because the timeconsuming hidden states are merely involved in training process and only efficient visible states are computed in inference process, the proposed method is very efficient. Moreover, we demonstrate that incorporating the phase-indicator vector (containing sequentially selected phases) as an additional input to the transformer used for reconstructing from undersampled MR images can significantly improve image reconstruction accuracy. Experiments on fastMRI dataset demonstrate effectiveness and efficiency of the proposed method.
更多查看译文
关键词
Fast scan,Phase encoding,Magnetic resonance imaging,Reinforcement learning,Image reconstruction
AI 理解论文
溯源树
样例
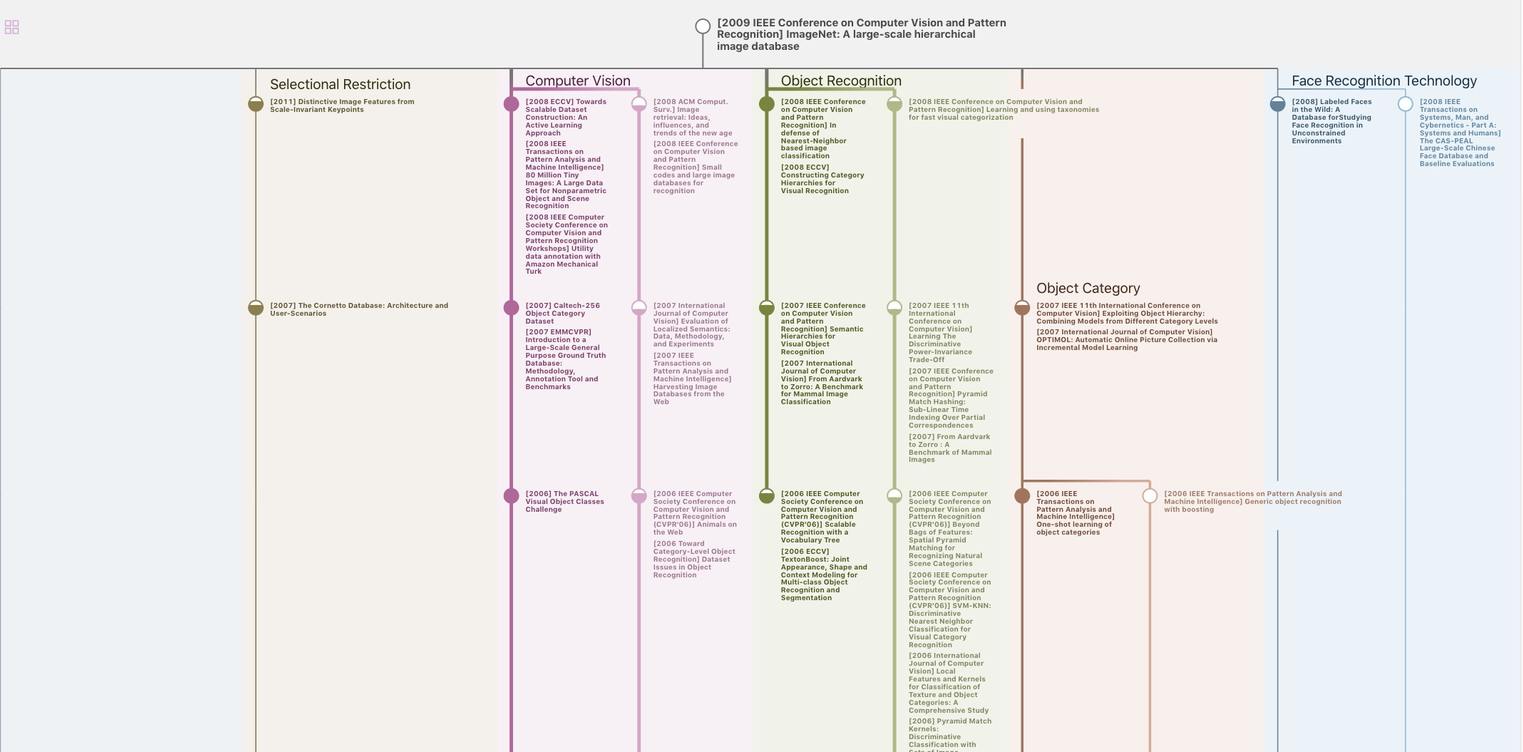
生成溯源树,研究论文发展脉络
Chat Paper
正在生成论文摘要