When explainability turns into a threat- using xAI to fool a fake news detection method
COMPUTERS & SECURITY(2024)
摘要
The inclusion of Explainability of Artificial Intelligence (xAI) has become a mandatory requirement for designing and implementing reliable, interpretable and ethical AI solutions in numerous domains. xAI is now the subject of extensive research, from both the technical and social science perspectives. It is being received enthusiastically by legislative bodies and regular users of machine-learning-boosted applications alike. However, opening the black box of AI comes at a cost. This paper presents the results of the first study proving that xAI can enable successful adversarial attacks in the domain of fake news detection and lead to a decrease in AI security. We postulate the novel concept that xAI and security should strike a balance, especially in critical applications, such as fake news detection. An attack scheme against fake news detection methods is presented that employs an explainable solution. The described experiment demonstrates that the well-established SHAP explainer can be used to reshape the structure of the original message in such a way that the value of the model's prediction could be arbitrarily forced, whilst the meaning of the message stays the same. The paper presents various examples for which the SHAP values are used to point the adversary to the words and phrases that have to be changed to flip the label on the model prediction. To the best of the authors' knowledge, it has been the first research work to experimentally demonstrate the sinister side of xAI. As the generation and spreading of fake news has become a tool of modern warfare and a grave threat to democracy, the potential impact of explainable AI should be addressed as soon as possible.
更多查看译文
关键词
Explainable AI,Adversarial attacks,NLP,Fake news,BERT
AI 理解论文
溯源树
样例
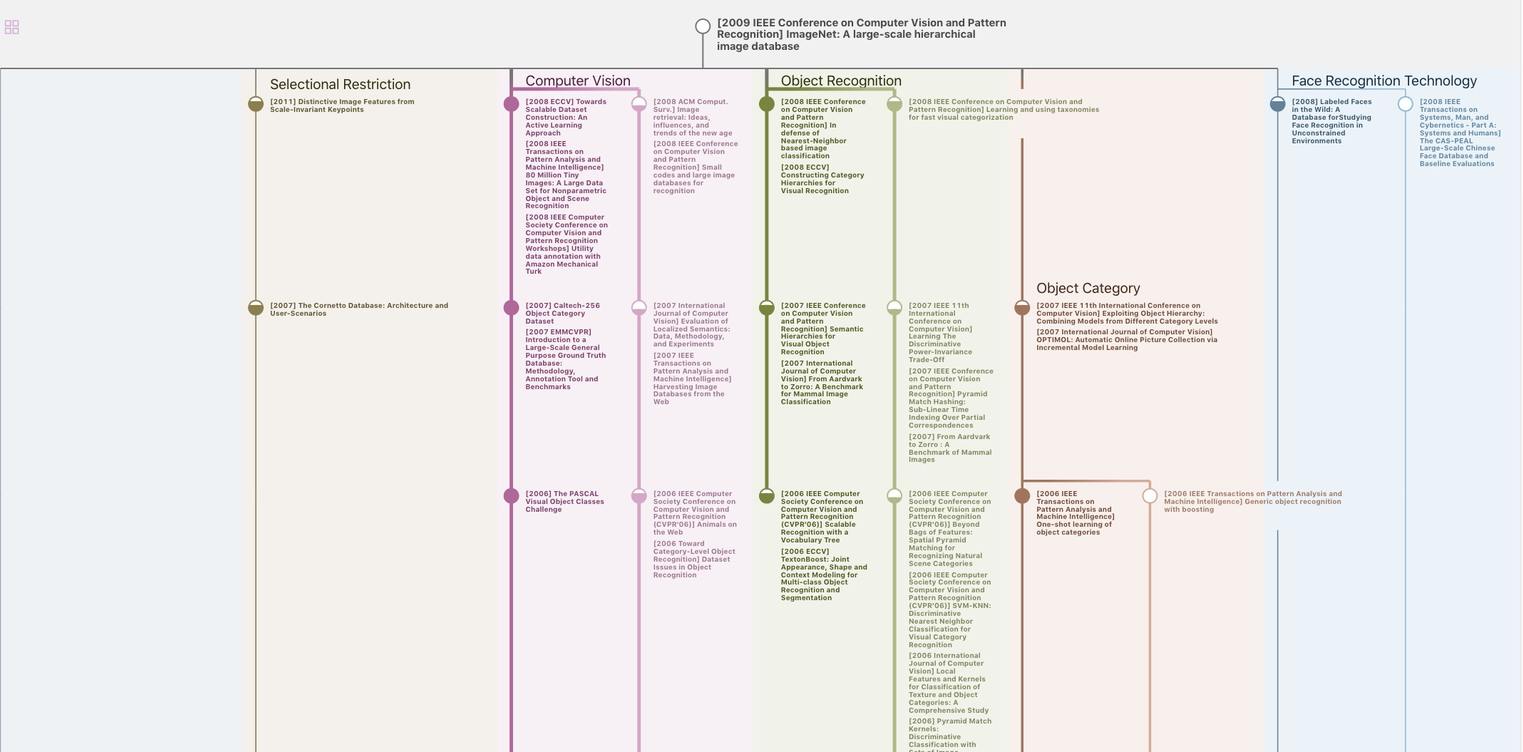
生成溯源树,研究论文发展脉络
Chat Paper
正在生成论文摘要