Collaborative representation based cross-domain semantic transfer for vehicle re-identification
NEUROCOMPUTING(2024)
摘要
Vehicle re-identification (re-ID) has received increasing attention due to its tremendous potential in practical intelligent transportation scenarios. The main difficulties of vehicle re-ID are generally induced by insufficient annotations, varying viewpoints and illuminations, and mismatched visual-semantic cues. Previous studies on vehicle re-ID have generally focused on exploring better visual representations of the target samples while ignoring semantic complementarity from external sources. In this paper, we propose an unsupervised semantic transfer method based on collaborative matrix representation (STCMR) for vehicle re-ID that obtains rich semantic representations by learning knowledge from external annotated data. STCMR contains three components: an asymmetrical collaborative matrix representation term, a low-rank regularized relaxed attribute term, and a dual-graph Laplacian regularization term. In particular, we first build two reliable feature matrices to better transfer external annotated semantics from the source domain to the target re-ID domain. We then propose a robust collaborative matrix representation term to decompose the feature matrices into user-defined, domain-shared, and domain-unique parts in an asymmetrical manner. To tackle samples with incomplete semantics, we design a low-rank regularized attribute relaxed term that fully exploits existing annotations to complete missing entries in a soft constraint manner. Finally, we exploit the local geometric structure consistency in source and target domains by a dual-graph Laplacian regularization term to adaptively estimate the relationships of source domain samples. In addition, we further propose an alternating iterative algorithm to solve the optimization problem. Experimental results conducted on the VehicleID and VeRi-776 datasets validate the effectiveness of our proposed method.
更多查看译文
关键词
Vehicle re-identification,Transfer learning,Matrix representation,Low-rank
AI 理解论文
溯源树
样例
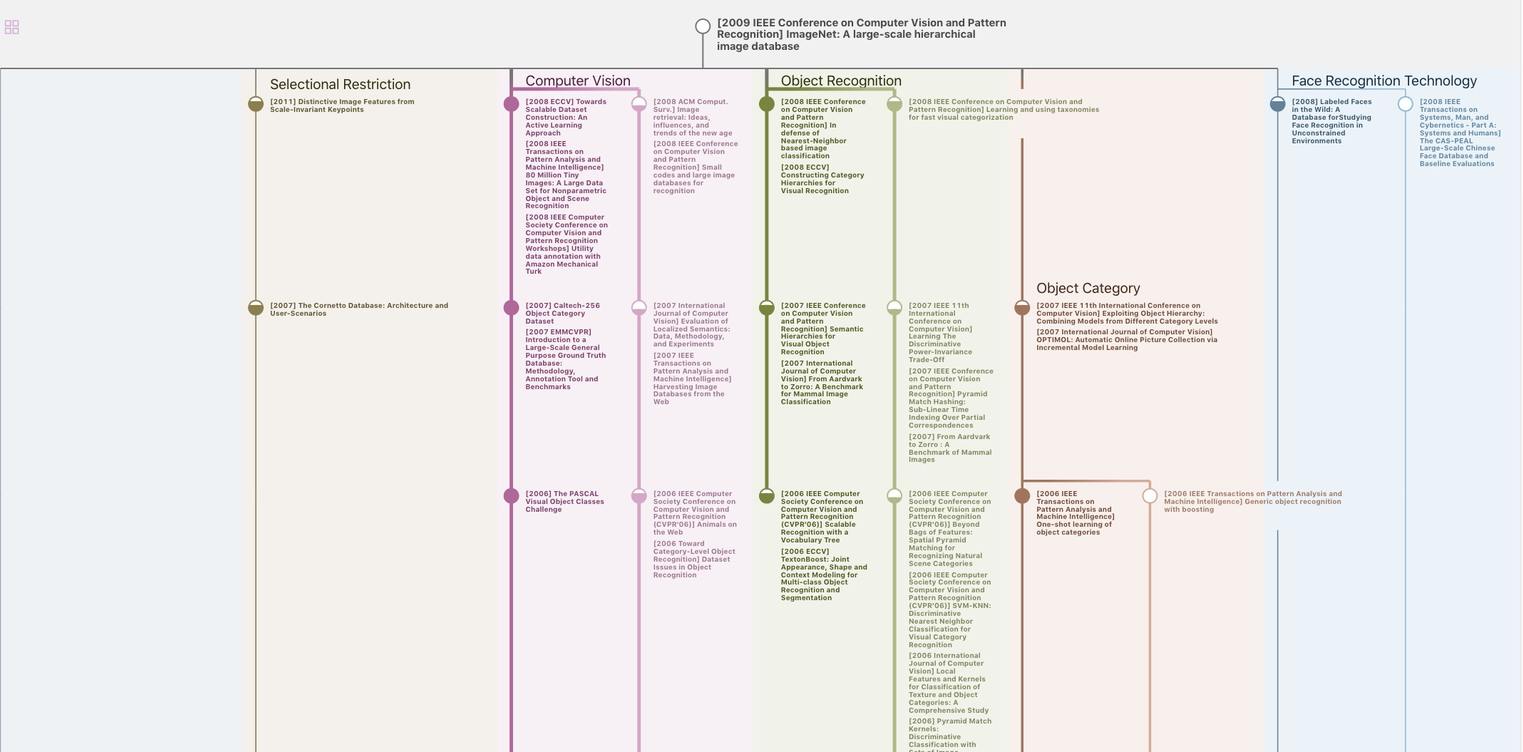
生成溯源树,研究论文发展脉络
Chat Paper
正在生成论文摘要