Surpassing early stopping: A novel correlation-based stopping criterion for neural networks
NEUROCOMPUTING(2024)
摘要
During the training of neural networks, selecting the right stopping criterion is crucial to prevent overfitting and conserve computing power. While the early stopping and the maximum number of epochs stopping methods are simple to implement, they have limitations in identifying the point during training where the training and the validation loss start to diverge. To overcome these limitations, we propose a general correlation-based stopping criterion called the Correlation-Driven Stopping Criterion (CDSC). The CDSC stops the training process when the rolling Pearson correlation of the loss metrics between the training and validation datasets decreases below a pre-defined threshold. To show the effectiveness of the newly proposed Correlation-Driven Stopping Criterion, its effectiveness was compared with the effectiveness of the early stopping and the maximum number of epochs stopping methods across multiple common machine learning problems and neural network models. Our study shows that the proposed Correlation-Driven Stopping Criterion can enhance the out-of-sample performance of all tested neural network models while conserving computing power.
更多查看译文
关键词
Neural network,Stopping criterion,Early stopping,Correlation-Driven Stopping Criterion,Regularization,Pearson correlation
AI 理解论文
溯源树
样例
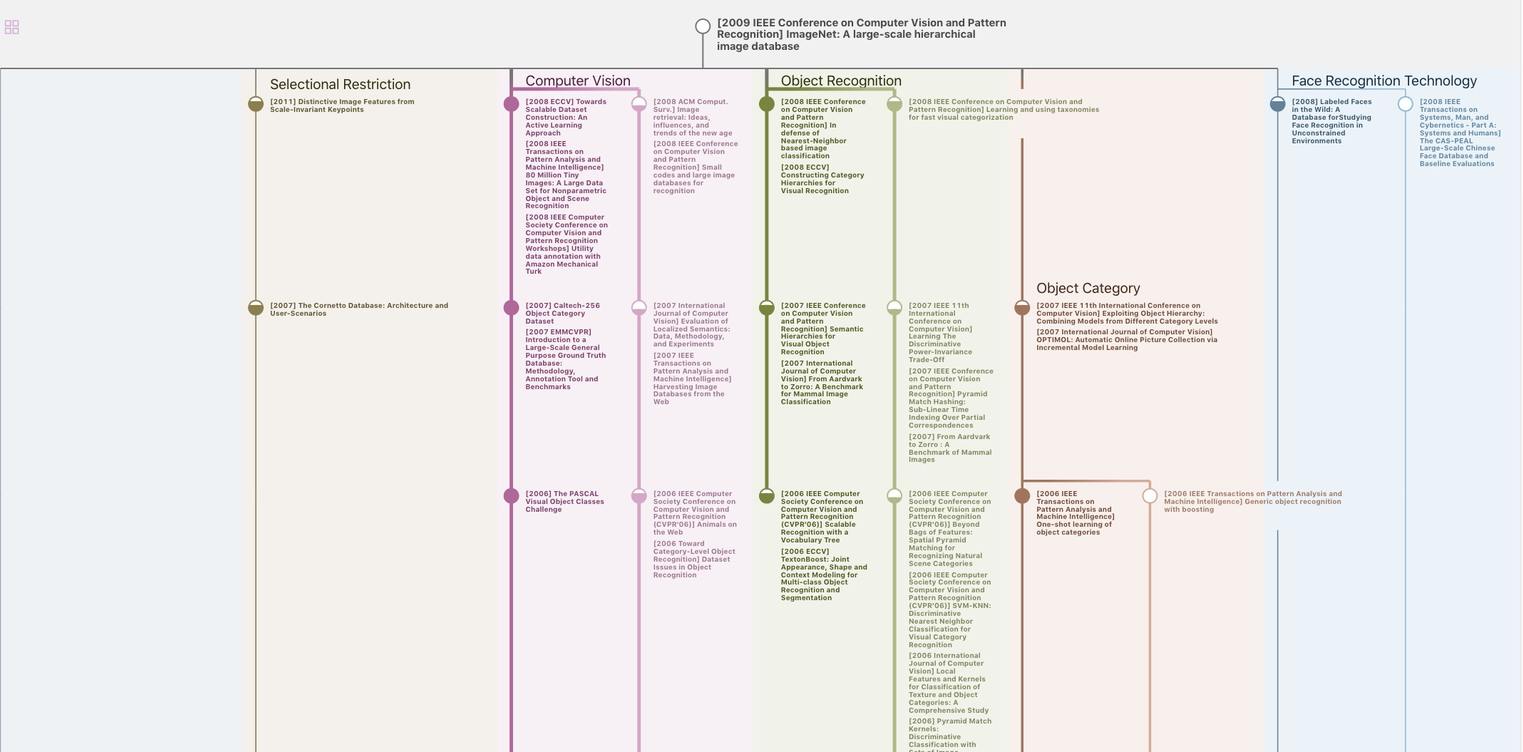
生成溯源树,研究论文发展脉络
Chat Paper
正在生成论文摘要