A differential evolution algorithm for solving mixed-integer nonlinear programming problems
SWARM AND EVOLUTIONARY COMPUTATION(2024)
摘要
Many engineering optimization problems fall into the category of Mixed-Integer Nonlinear Programming (MINLP) problems, which combine nonlinear relations, constraint conditions, and different types of variables, including continuous, integer, and/or discrete variables. Solving MINLP problems can be a challenging exploration process since their landscape can be composed of many discontinuous feasible parts with different sizes. In this scheme, Evolutionary Algorithms (EAs) often fail to generate enough diversity to explore the discontinuous feasible parts. Consequently, EAs are vulnerable to being attracted to larger discontinuous feasible parts, even if they are not promising regions. In this paper, a new proposal based on two fundamental strategies is presented to improve the performance of the differential evolution algorithm when solving MINLP problems. The first strategy considers a set of "good fitness-infeasible solutions" that contribute to exploring promising regions from infeasible contours. It reduces the vulnerability of the solutions to be attracted to larger discontinuous feasible parts with unpromising objective function values. The second is a composite trial vector generation to improve the combinatorial exploration while ensuring a robust convergence capacity toward the final solution. Sixteen well-known MINLP problems are used in several experiments to evaluate the performance of the proposed algorithm, comparing it to state-of-the-art EAs. The results provided by the proposal show a better performance in terms of quality, robustness, and computational cost.
更多查看译文
关键词
Differential evolution (DE),Discrete variables,Engineering optimization problems,Integer restriction handling,Mixed-integer nonlinear programming (MINLP)
AI 理解论文
溯源树
样例
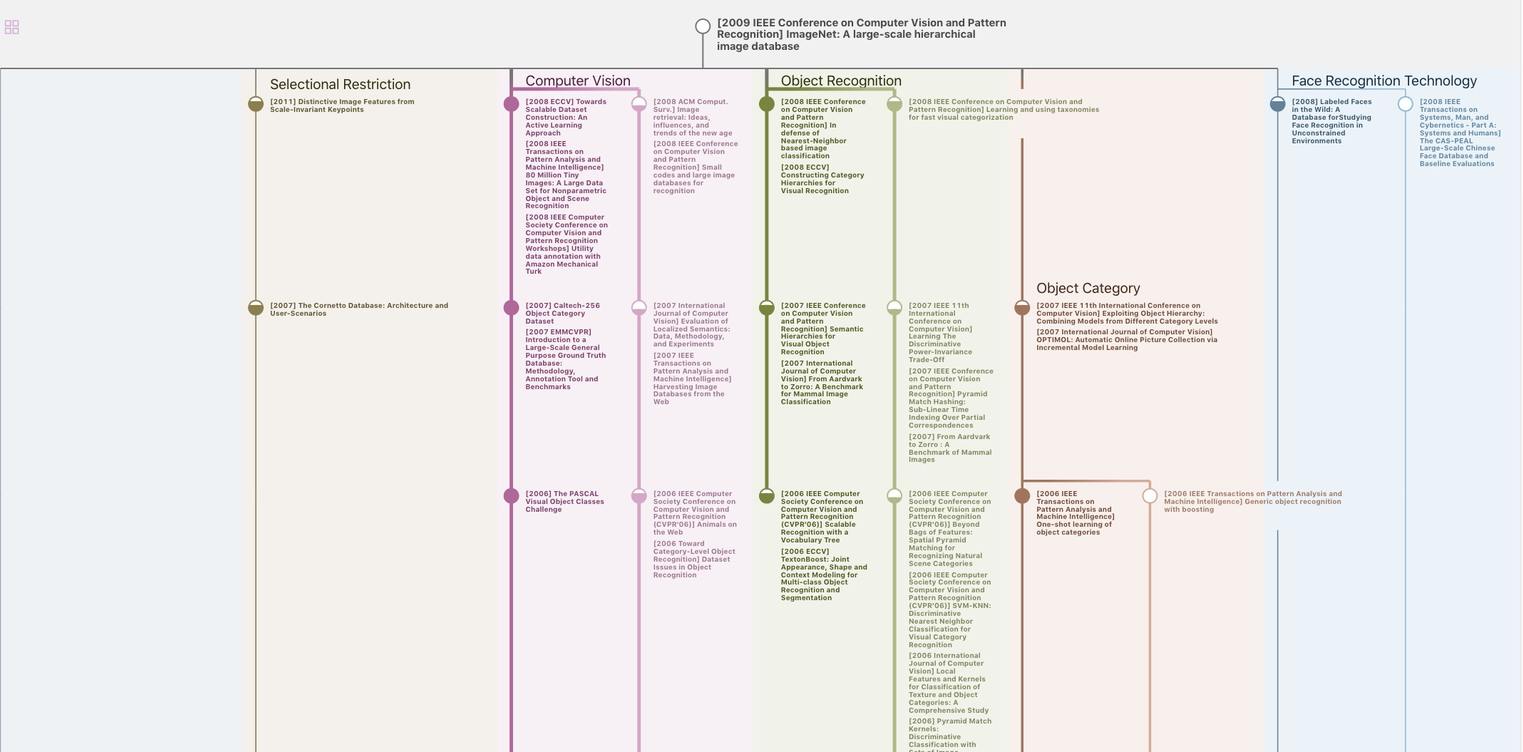
生成溯源树,研究论文发展脉络
Chat Paper
正在生成论文摘要