Control of the Cart-Pole System: Model-based vs. Model-free Learning
IFAC PAPERSONLINE(2023)
摘要
In this paper, we study the control design problem for a cart-pole system without the prior knowledge of its physical parameters. The control task involves both swing-up and balancing. Two control methods are compared: 1) model-free reinforcement learning; 2) systemidentification based control design. The former uses a popular deep deterministic policy gradient (DDPG) algorithm, whereas the latter uses a model-based design together with a system identification method for parameter estimation. The results show that system-identification based control design is far superior than reinforcement learning in terms of training, computation complexity and control performance, but requiring skills for system modelling, parameter estimation and control design. Copyright (c) 2023 The Authors.
更多查看译文
关键词
Reinforcement learning,optimal control,system identification.
AI 理解论文
溯源树
样例
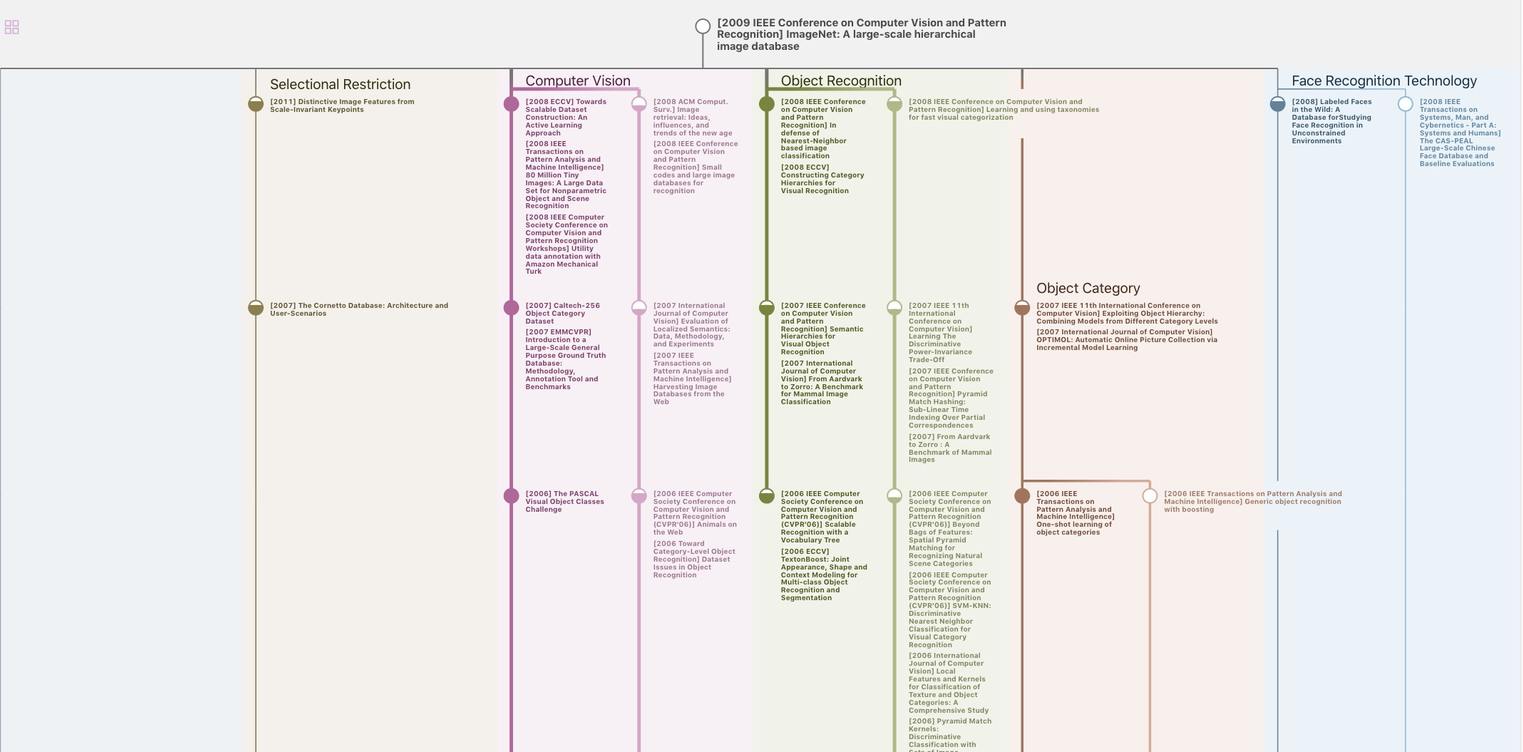
生成溯源树,研究论文发展脉络
Chat Paper
正在生成论文摘要