Deep Learning based monocular fill level detection for an eKanban system
IFAC PAPERSONLINE(2023)
摘要
Timely material replenishment is a key requirement for realizing lean manufacturing. As the monitoring of inventory levels and triggering replenishment is still often carried out manually by human workers, the automation of this process offers great potential for optimization. Existing automation solutions have either a high cost or high integration effort. Therefore, by using modern advancements in deep learning-based image processing, we propose low-cost sensor modules for automated fill level detection in load carriers. An inexpensive RGB camera represents the main sensor for the measurement to keep costs low. A convolutional neural network model is used to determine the fill levels. In contrast to usual monocular depth estimation approaches, our method has just the determination of the fill level as a goal to achieve high precision. The algorithm was trained and tested on the base of two datasets and achieved an average deviation of 7% to the true fill level value, verifying the viability of this approach.
更多查看译文
关键词
deep learning,inventory monitoring,fill level detection,machine learning,convolutional neural networks. image processing,transfer learning,material replenishment
AI 理解论文
溯源树
样例
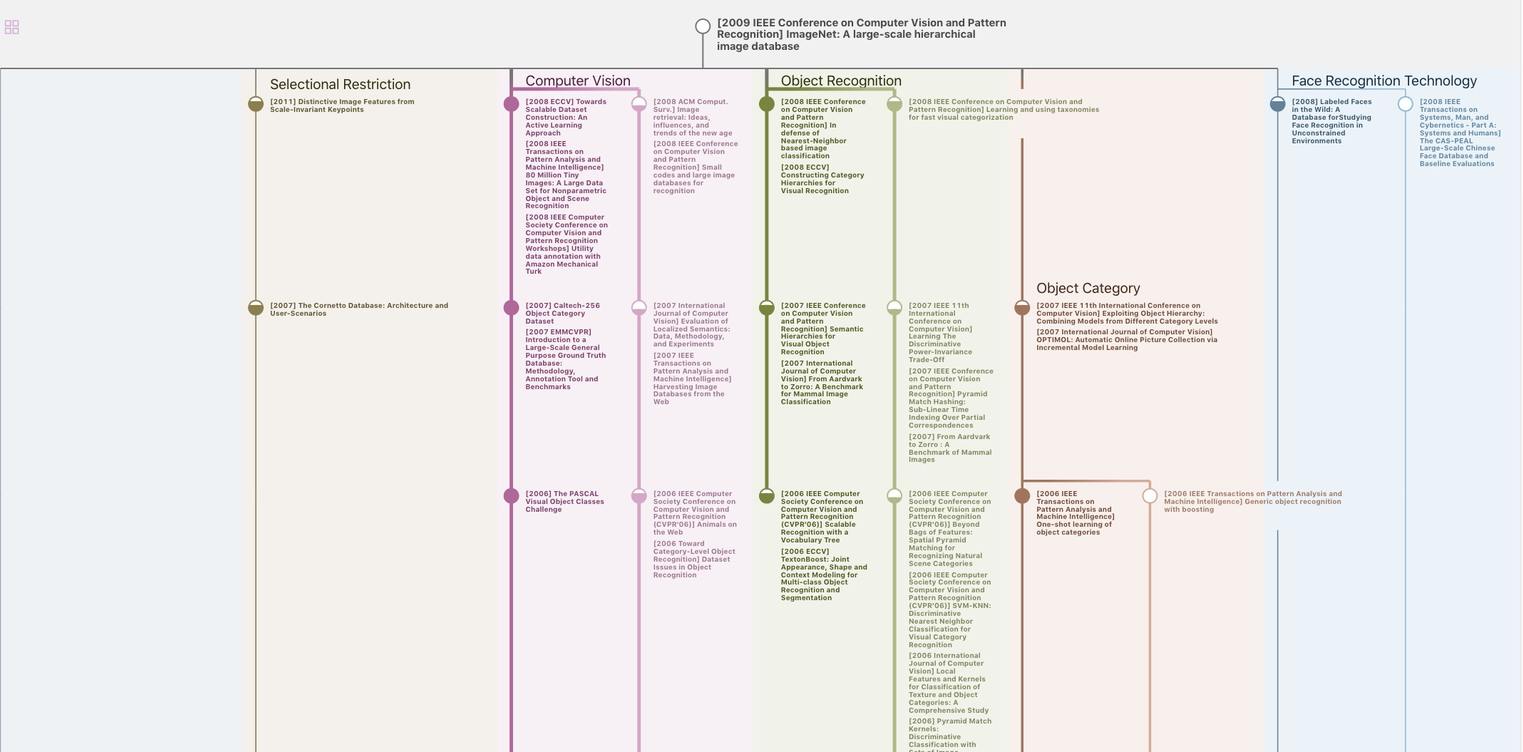
生成溯源树,研究论文发展脉络
Chat Paper
正在生成论文摘要