A constrastive semi-supervised deep learning framework for land cover classification of satellite time series with limited labels
NEUROCOMPUTING(2024)
摘要
In this work, we present a new semi-supervised learning framework to cope with satellite image time series (SITS) classification in a data paucity scenario, considering extreme low levels of supervision. The proposed methodology, referred as S3ITS (Semi-Supervised Satellite Image Time Series classification method), is based on temporal convolutional neural networks and it takes advantage of both labelled and unlabelled information. S3ITS enforces the data to be projected in a discriminative manifold via contrastive learning, in order to produce a data representation where samples belonging to the same category are closer than the ones belonging to different ones. Pseudo-labelling is employed on unlabelled samples to take the most out of the available unlabelled information. Experiments on two study sites described by SITS of Sentinel-2 images highlight the quality of the proposed method with respect to common SITS-based classification methods and recent machine learning approaches especially tailored for the semi-supervised classification of multi-variate time series data.
更多查看译文
关键词
Satellite image time series,Land cover mapping,Semi-supervised learning
AI 理解论文
溯源树
样例
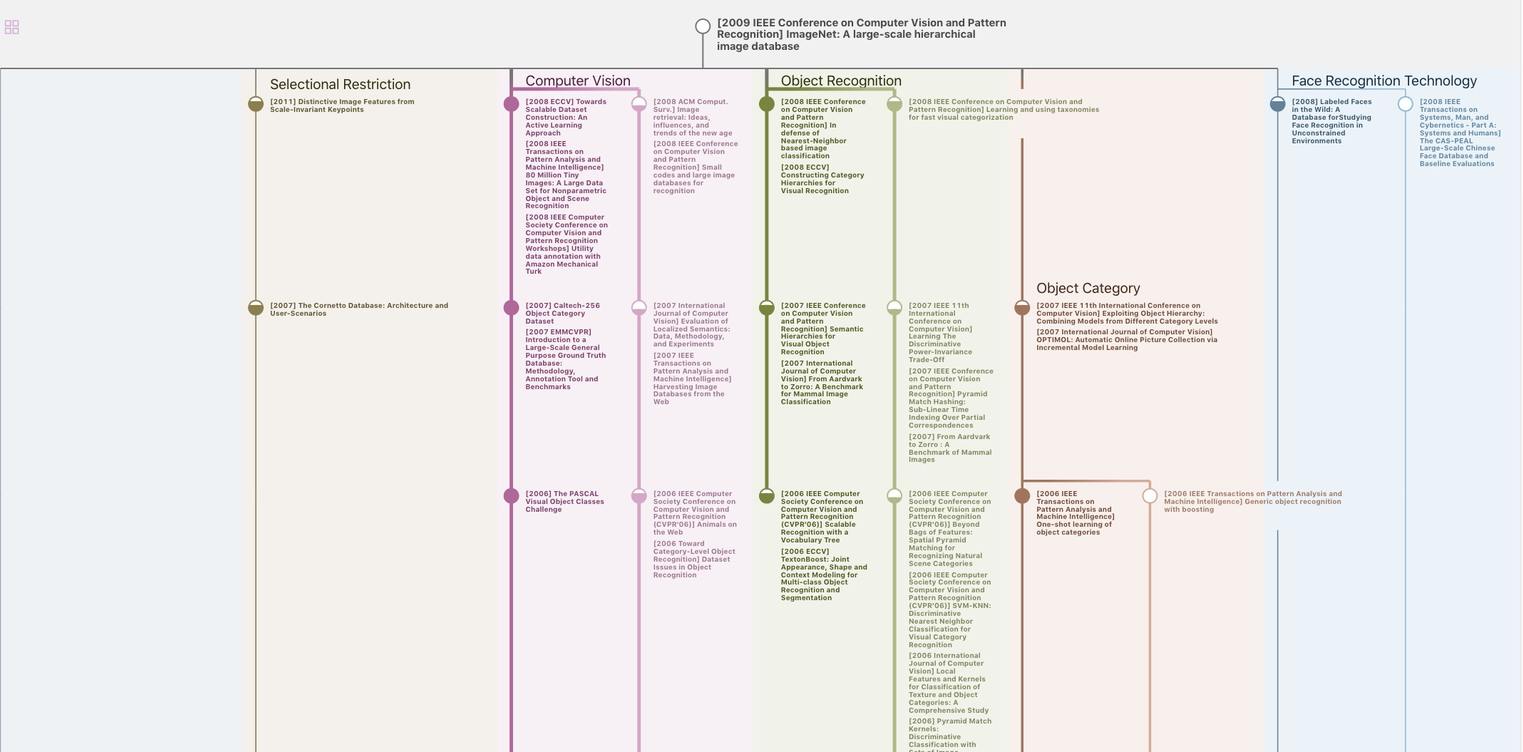
生成溯源树,研究论文发展脉络
Chat Paper
正在生成论文摘要