A High-Speed Method of Segmenting Human Body Motions with Regular Time Interval Sensor Data Based on Gaussian Process Hidden Semi-Markov Model
IFAC-PapersOnLine(2023)
摘要
Real-time action detection and feedback systems are needed to reduce the load on assembly line workers. Segmenting motion based on a Gaussian process hidden semi-Markov model (GP-HSMM) is suitable for detecting workers’ actions because an assembly line involves repeated movements. However, GP-HSMM has bottlenecks in calculating inverse covariance matrices and forward probabilities. To remove those bottlenecks, we first converted block covariance matrices into circulant matrices by combining sensor data at the same time step with a distributional kernel so that we can solve them with an FFT. Secondly, we parallelized forward probabilities by shifting rows of the log-likelihood matrix, which is calculated from all of the combinations between the signal patterns generated from the Gaussian process and observed data, and summing up rows and columns incrementally. In an experiment with 2593 × 11 electromyography and inertial measurement unit time series data, our algorithm was 495 times faster than conventional methods.
更多查看译文
关键词
Motion segmentation,Gaussian process,Hidden semi-Markov model,Electro-myography,Inertial measurement unit,Assembly line,Human operator support,Modeling of human performance
AI 理解论文
溯源树
样例
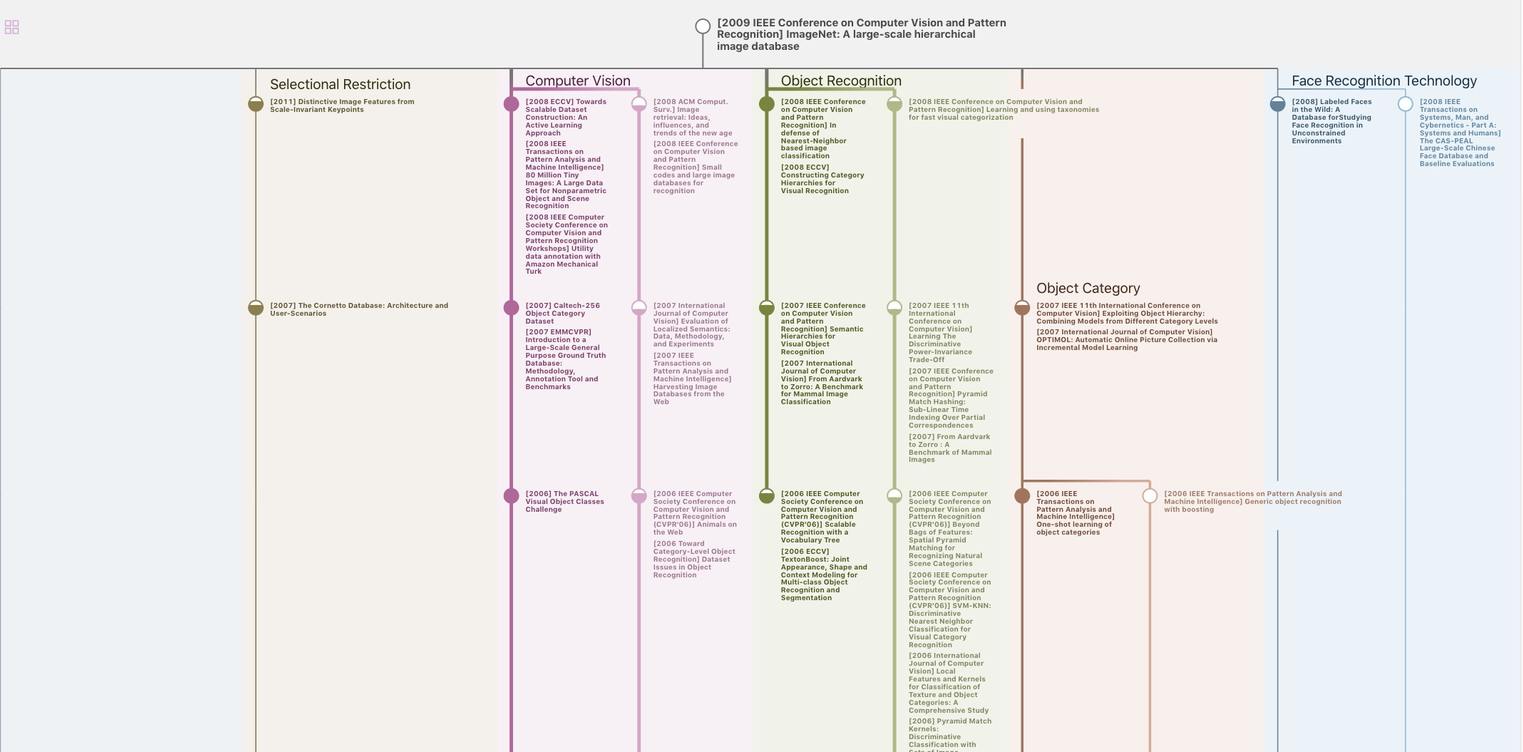
生成溯源树,研究论文发展脉络
Chat Paper
正在生成论文摘要