Model Identification with Incomplete Input Data in Type 1 Diabetes*
IFAC-PapersOnLine(2023)
摘要
A major challenge in fitting models to glucose metabolism in people with type 1 diabetes is incomplete data as its collection partially relies on self-reporting and does not include all relevant events. We develop a method for identifying optimal input corrections to reestablish a correct input-output relationship in the data while jointly identifying personalized model parameters. The unreported or misreported parts in the data are reconciled by adding sparse corrections via mixed-integer quadratic programming leading to an improved identification of the model parameters. We conduct numerical experiments with incomplete in-silico training data and show that models obtained from our method are able to provide more accurate predictions on test data than models obtained from standard methods. The performance of our methodology is similar to that attained with the standard method when trained on data with complete information.
更多查看译文
关键词
Identification and validation,Quantification of physiological parameters,Grey box modeling,Physiological Model,Type 1 Diabetes
AI 理解论文
溯源树
样例
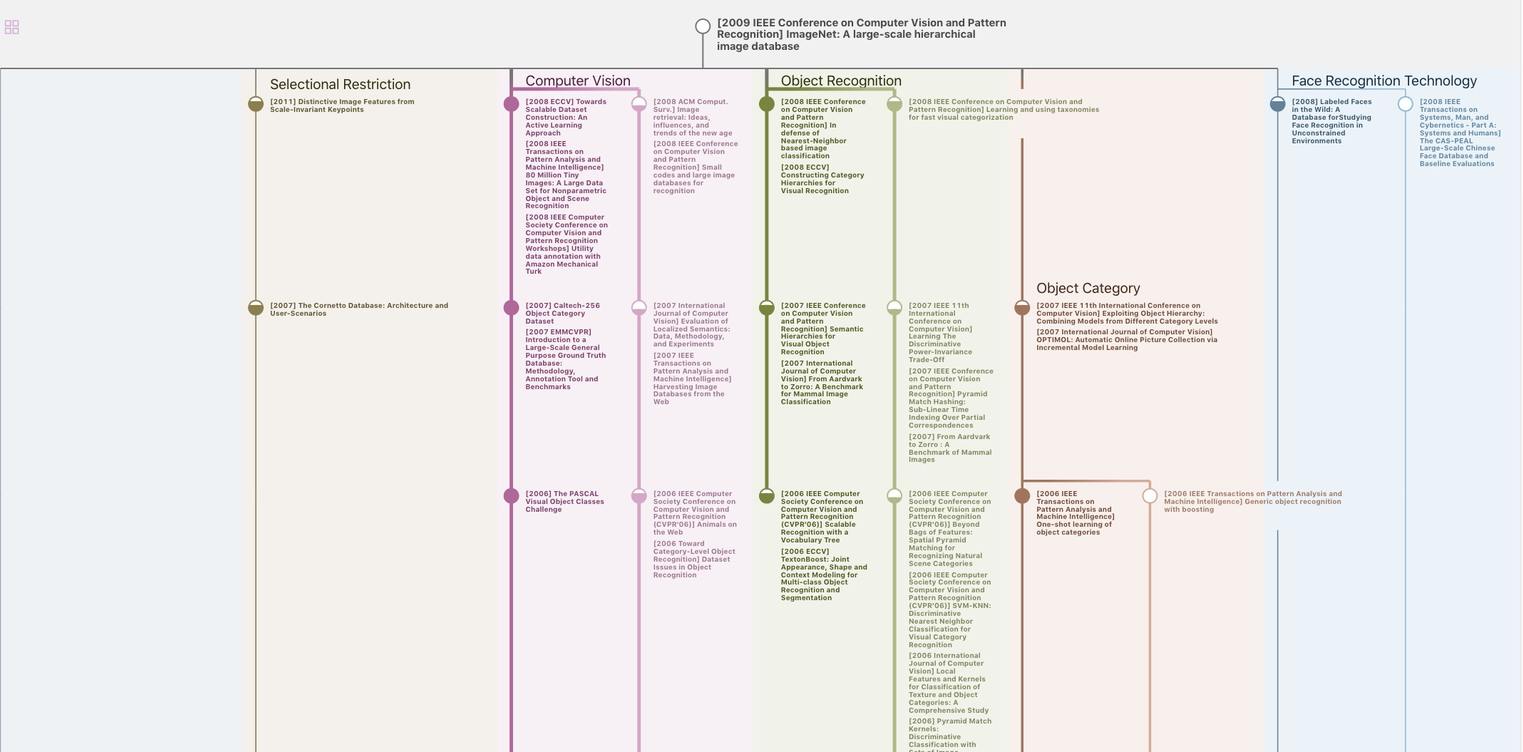
生成溯源树,研究论文发展脉络
Chat Paper
正在生成论文摘要