A Real-Time Gaussian Process-Based Stochastic Controller for Periodic Disturbances
IFAC PAPERSONLINE(2023)
摘要
Using a non-parametric Gaussian process regression (GPR) framework, we present in this paper a practical learning technique to model, filter, and predict periodic disturbances. Augmenting a known system model with the GP model of the disturbance results in a structure referred to as latent force model (LFM), which is also a GP with an appropriate covariance function. By representing the LFM as a stochastic state-space model using spectral factorization, we apply a full Bayesian inference through Kalman filter and smoother, and design a linear quadratic regulator (LQR) to achieve an optimal closed-loop performance. On a real-time application, LJ MS15 DC motor under induced periodic disturbances, the proposed approach is implemented successfully. Real-time experiments demonstrate the efficiency and practicality of the proposed LFM-based LQR compared to the classical LQR approach. Copyright (c) 2023 The Authors.
更多查看译文
关键词
Gaussian process regression,Stochastic optimal control,Kalman filtering,LQR
AI 理解论文
溯源树
样例
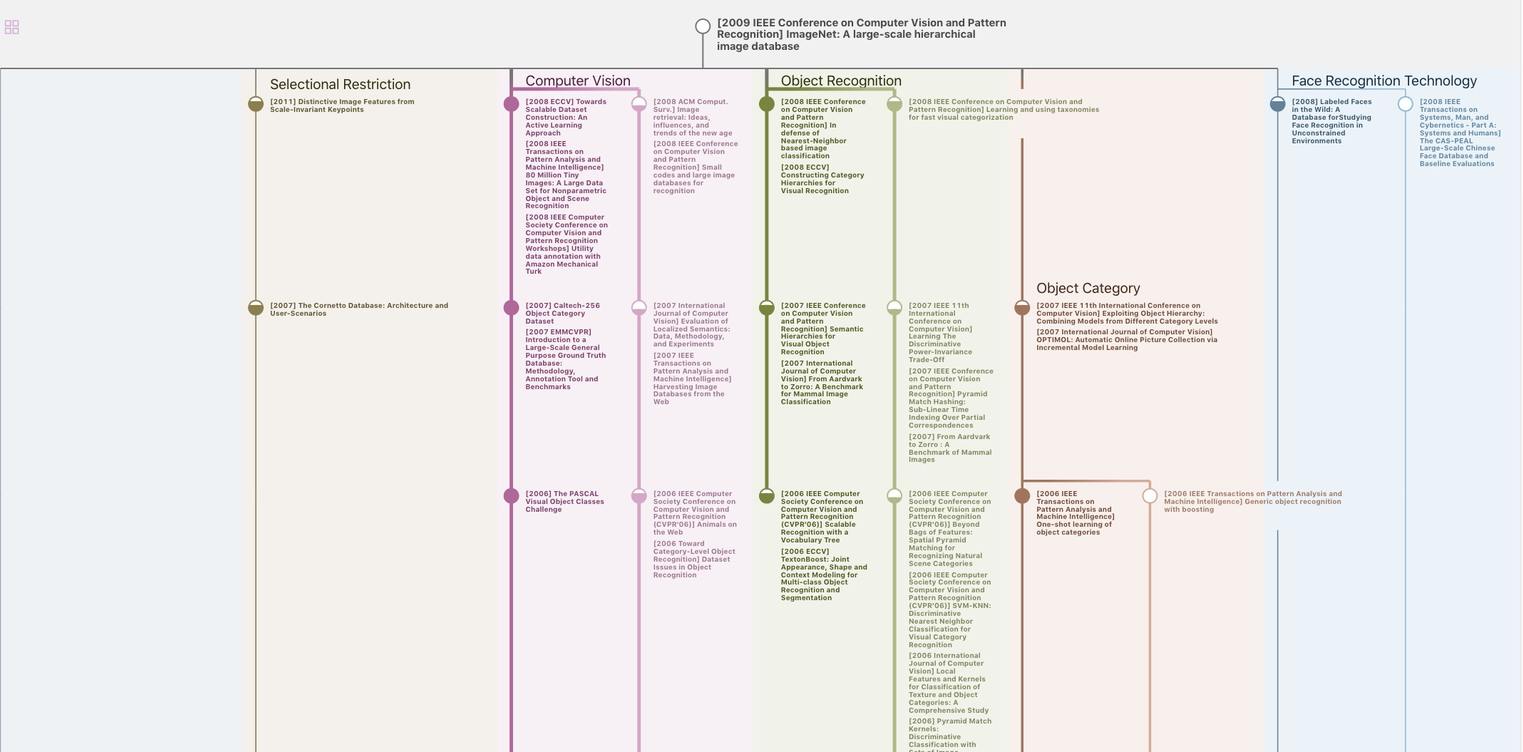
生成溯源树,研究论文发展脉络
Chat Paper
正在生成论文摘要