A comparative Study of Data-driven Prognostic Approaches under Training Data Deficiency
IFAC PAPERSONLINE(2023)
摘要
In this study, a remaining useful life (RUL) prognostic performance is explored for the three algorithms under the insufficient training datasets: data augmentation prognostics (DAPROG) developed by the authors, and trajectory similarity-based prediction (TSBP) and multilayer perceptron (MLP) based neural network, which is one of the popular approaches in the literature. The Commercial Modular AeroPropulsion System Simulation (CMAPSS) datasets are taken to assess each algorithm. Only a fraction is chosen randomly from the training dataset to simulate the data deficiency and evaluate the prognostic performance. As a result, the DAPROG is found to be the most superior in terms of the prediction error when the data deficiency is severe. The prediction variance for the chosen training dataset is also small, indicating more uniform performance.
更多查看译文
关键词
Prognostics and Health Management,Remaining Useful Life,Trajectory Similarity Based Prediction,Data Augmentation Prognostics,Multilayer Perceptron,Health index,Dynamic Time,Warping
AI 理解论文
溯源树
样例
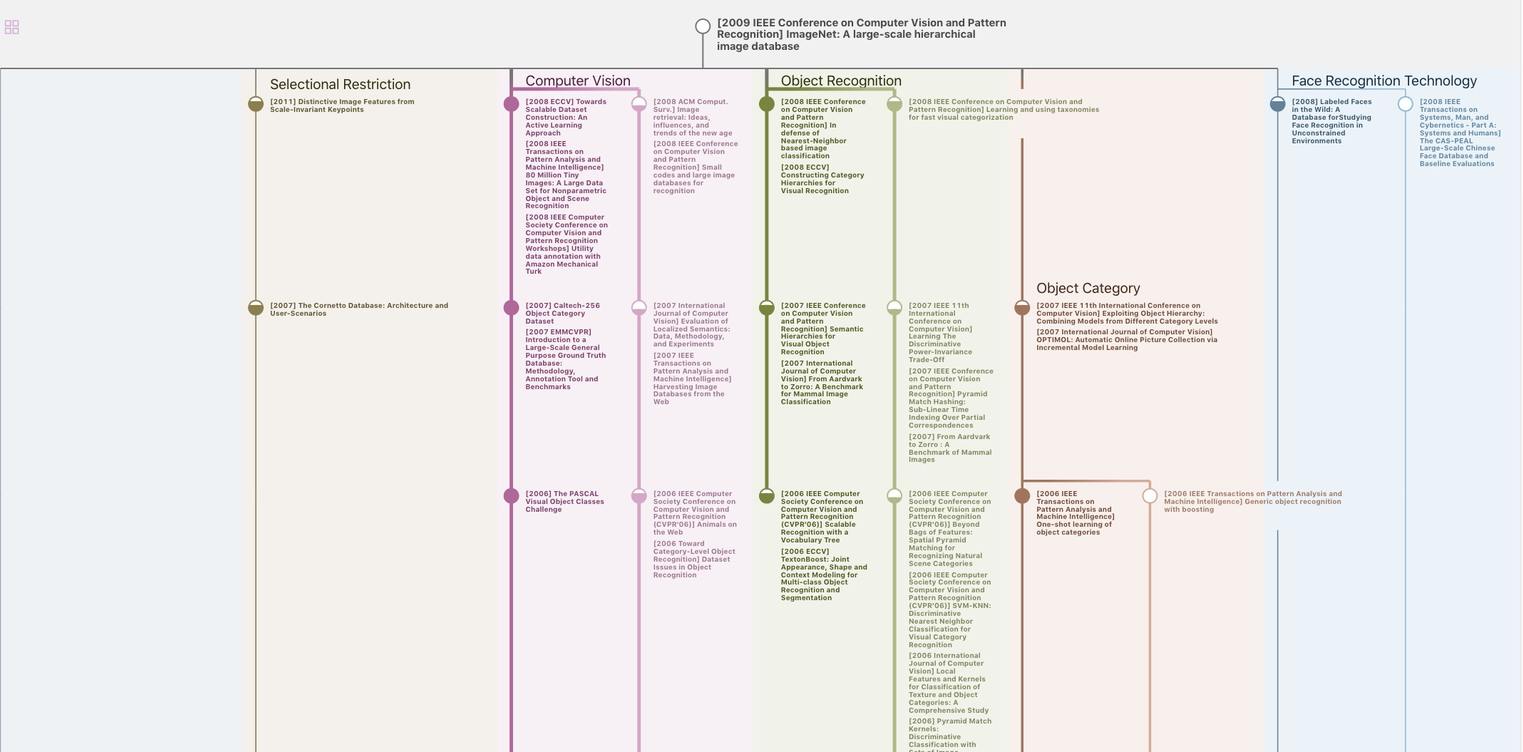
生成溯源树,研究论文发展脉络
Chat Paper
正在生成论文摘要