A data-based neural controller training method with tunable stability margin using multi-objective optimization
IFAC PAPERSONLINE(2023)
摘要
This paper presents a method to design neural network controllers based on imitation learning and with tunable stability guarantees through multi-objective optimization. Stability margins are derived from analyzing state-space neural networks based on the representation of nonlinear activation functions by linear parameter varying models. The controller training is formulated as a multi-objective problem whose solutions yield a set of the best trade-offs in terms of minimal imitation error and maximal stability margins. The proposed approach is illustrated in the synthesis by imitation of an aircraft neural autopilot using a flight simulator.
更多查看译文
关键词
Imitation learning,State-space neural networks,Multi-objective optimization
AI 理解论文
溯源树
样例
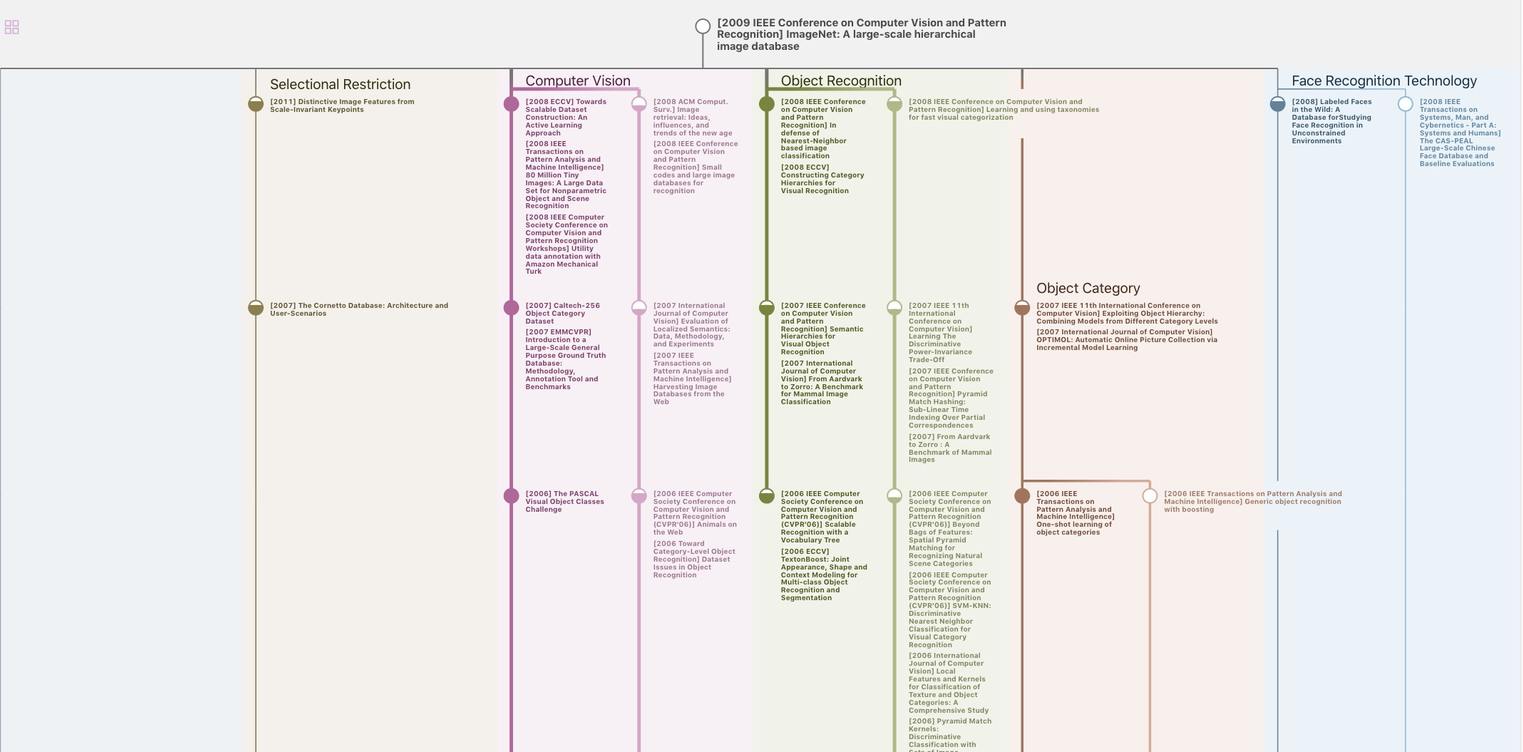
生成溯源树,研究论文发展脉络
Chat Paper
正在生成论文摘要