A deep reinforcement learning based algorithm for a distributed precast concrete production scheduling
INTERNATIONAL JOURNAL OF PRODUCTION ECONOMICS(2024)
摘要
The environmental-friendly production demands higher manufacturing efficiency and lower energy cost; therefore, time-of-use electricity price constraint and distributed production have attracted more attention. For concrete precast architecture construction, the tardiness penalty and warehouse cost cannot be ignored, which should be optimized for lower cost. In this study, the concrete precast process is investigated as the group scheduling of a distributed flexible job shop problem. Each concrete precast is managed in a group, the setup time between different groups is considered. Two objectives, total weighted earliness and tardiness and total time-of-use electricity cost are minimized, simultaneously. To solve the integrated problem, three coordinated double deep Q-networks (DQN) are applied, which are organized as a learn-to-improve reinforcement learning approach. For distributed scheduling problem, operators in a single factory or in multiple factories differs in solution improvement; so selection DQN is designed to decide the type of the operators according to scheduling circumstances. Other two DQNs, i.e., local DQN and global DQN, are to select the optimization operators in one factory or in multiple factories, respectively. Furthermore, two solution refinement strategies are designed to decrease the objectives after reinforcement learning component. Numerical experiment and statistical analysis suggest that the proposed deep reinforcement learning based algorithm has superiority in solving the considered problem.
更多查看译文
关键词
Reinforcement learning,Precast concrete production,Distributed flexible job shop scheduling problem,Group scheduling,Time-of-use electricity price
AI 理解论文
溯源树
样例
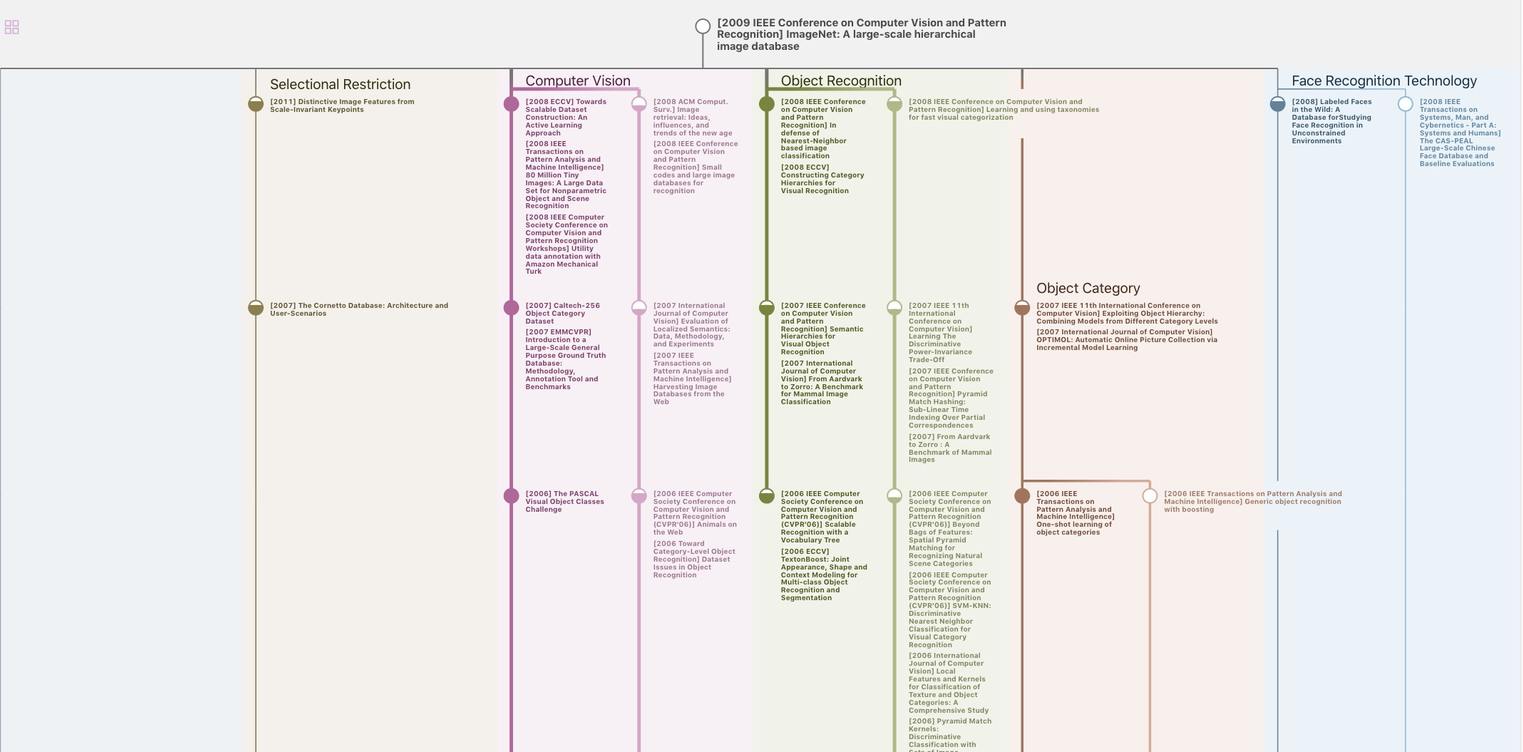
生成溯源树,研究论文发展脉络
Chat Paper
正在生成论文摘要