Acquisition Function Choice in Bayesian Optimization via Partially Observable Markov Decision Process
IFAC PAPERSONLINE(2023)
摘要
Bayesian optimization is a popular technique in optimization of functions which are expensive to evaluate (e.g. in experimental optimization of complex and slow processes). Bayesian optimizers select the points in which the cost function is evaluated via optimizing a surrogate acquisition function. Such acquisition function is usually fixed, based on intuition, from several choices available in the literature. However, for a given problem, it is sometimes difficult to know which is the right one and, perhaps, a strategy combining them along the experiments sequence might provides better results. This paper explores a scenario-based optimization to estimate the best sequence of acquisition function choices in finite-horizon optimization problems under uncertainty, cast as a Partially Observable Markov Decision Process. Improvements over standard Bayesian optimization and a recent rollout strategy are illustrated in simulation.
更多查看译文
关键词
POMDP,Experimental optimization,Dynamic programming,Learning
AI 理解论文
溯源树
样例
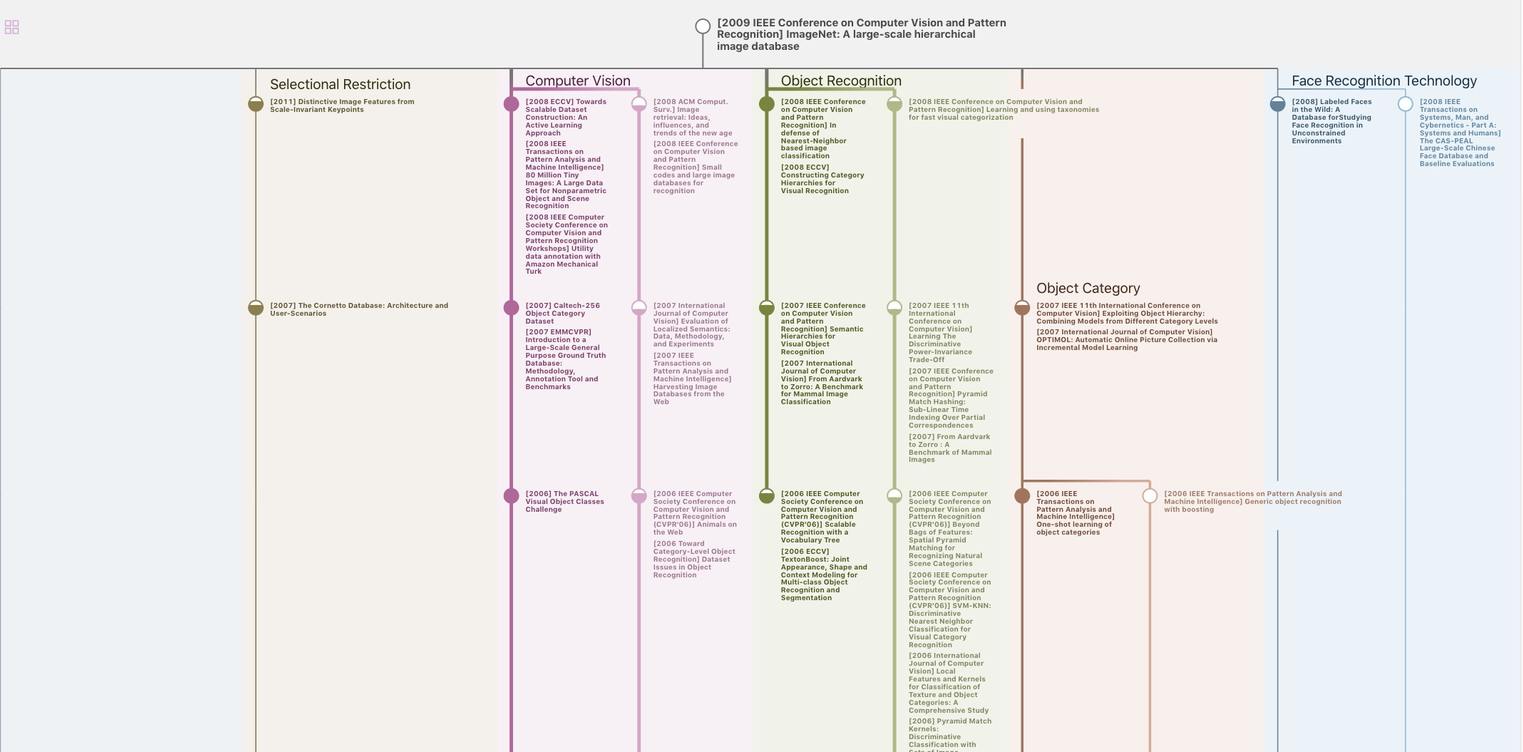
生成溯源树,研究论文发展脉络
Chat Paper
正在生成论文摘要