An advanced multi-objective collaborative scheduling strategy for large scale EV charging and discharging connected to the predictable wind power grid
ENERGY(2024)
摘要
With the large-scale EV connected into the wind power grid, the intermittent, fluctuating and stability bring rigorous challenges for power quality and dispatch, thus wind curtailment becomes more serious. This paper proposes an advanced multi-objective collaborative scheduling strategy to improve the accuracy of the predictable wind grid and ensure the stable operation of the grid by optimizing wind power and EV synergistically. Firstly, a hybrid power prediction method is proposed based on density-based spatial clustering applied to noise (DBSCAN) and partial least squares regression (PLSR) combined with long and short-term memory neural network (LSTM). It establishes a linear component prediction mode to predict the nonlinear wind power efficiently. Secondly, a multi-objective optimization scheduling model is established according to the predicted wind power, considering the vehicle-to-grid (V2G) characteristics of EV, grid load fluctuations, wind curtailment capacity, EV charging costs, and grid losses. The global control aims to achieve overall energy balance and optimize the charging and discharging of EV in a time dimension under constrained conditions. Additionally, based on the total number of EV charging and discharging determined by the global level control, a spatial scheduling optimization is conducted for EV and wind power by the distribution network control, with the goal of minimizing network losses in the distribution network system. Finally, through simulation and comparison of four scenarios, the results demonstrate that the proposed method achieves synergistic optimization of wind power and EV. This paper provides an efficient solution for the optimized scheduling of large-scale EV connected to the predictable wind power grid.
更多查看译文
关键词
Large-scale EV,Hybrid power prediction,Multi-objective optimization scheduling,Constrained conditions
AI 理解论文
溯源树
样例
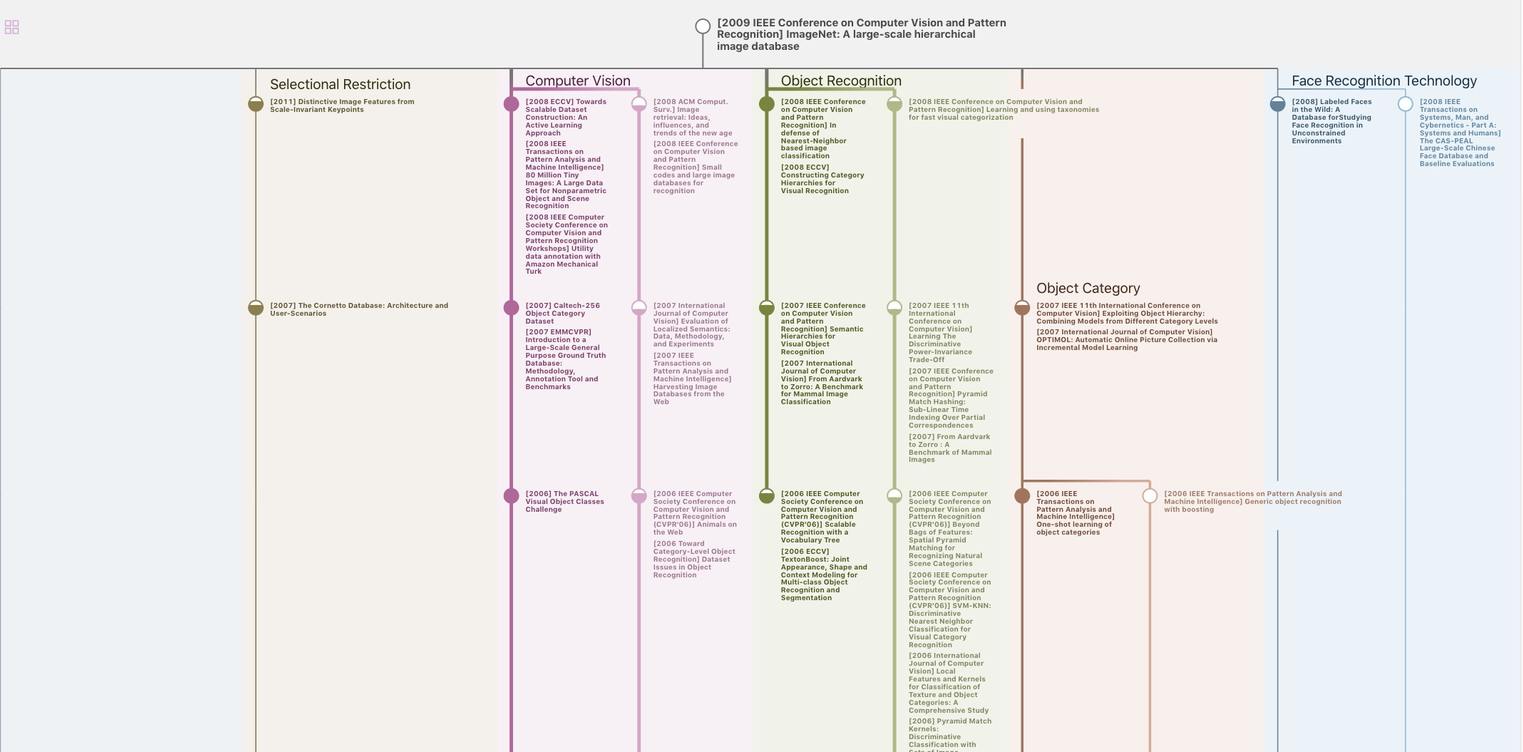
生成溯源树,研究论文发展脉络
Chat Paper
正在生成论文摘要