Wine quality assessment for Shiraz vertical vintages based on digital technologies and machine learning modeling.
FOOD BIOSCIENCE(2023)
摘要
Assessment of wine quality traits can be costly, time-consuming, and usually undertaken with complex chemical analysis and sensory evaluation in specialized laboratories. This study aimed to use novel digital technologies based on near-infrared (NIR) spectroscopy and a low-cost electronic nose (e-nose) integrated with machine learning modeling to assess wine quality traits and provenance in a vertical vintage of Shiraz wines. Results showed highly accurate machine learning (ML) models for the classification of wine vintages for Model 1 (NIR; 98.3%) and Model 2 (e-nose; 99.5%), and prediction of (i) intensity of sensory descriptors (NIR Model 3; R = 0.97), (ii) peak area of volatile aromatic compounds (NIR Model 4; R = 0.96), (iii) physicochemical parameters (NIR Model 5; R = 0.94), (iv) intensity of sensory descriptors (e-nose Model 6; R = 0.97), (v) peak area of volatile aromatic compounds (e-nose Model 7; R = 0.96), and (vi) physicochemical parameters (e-nose Model 8; R = 0.93). Winemakers may use these models to assess vintages and maintain high-quality wines associated with specific vineyards and regions or for a distinctive wine variety. Models could be developed further by including data from different vineyards, regions, wine variety, seasonality, other production, and winemaking techniques.
更多查看译文
关键词
Near-infrared spectroscopy,Electronic nose,Sensory profile,Volatile aromatic compounds,Wine quality traits,Gas chromatography-Mass spectroscopy
AI 理解论文
溯源树
样例
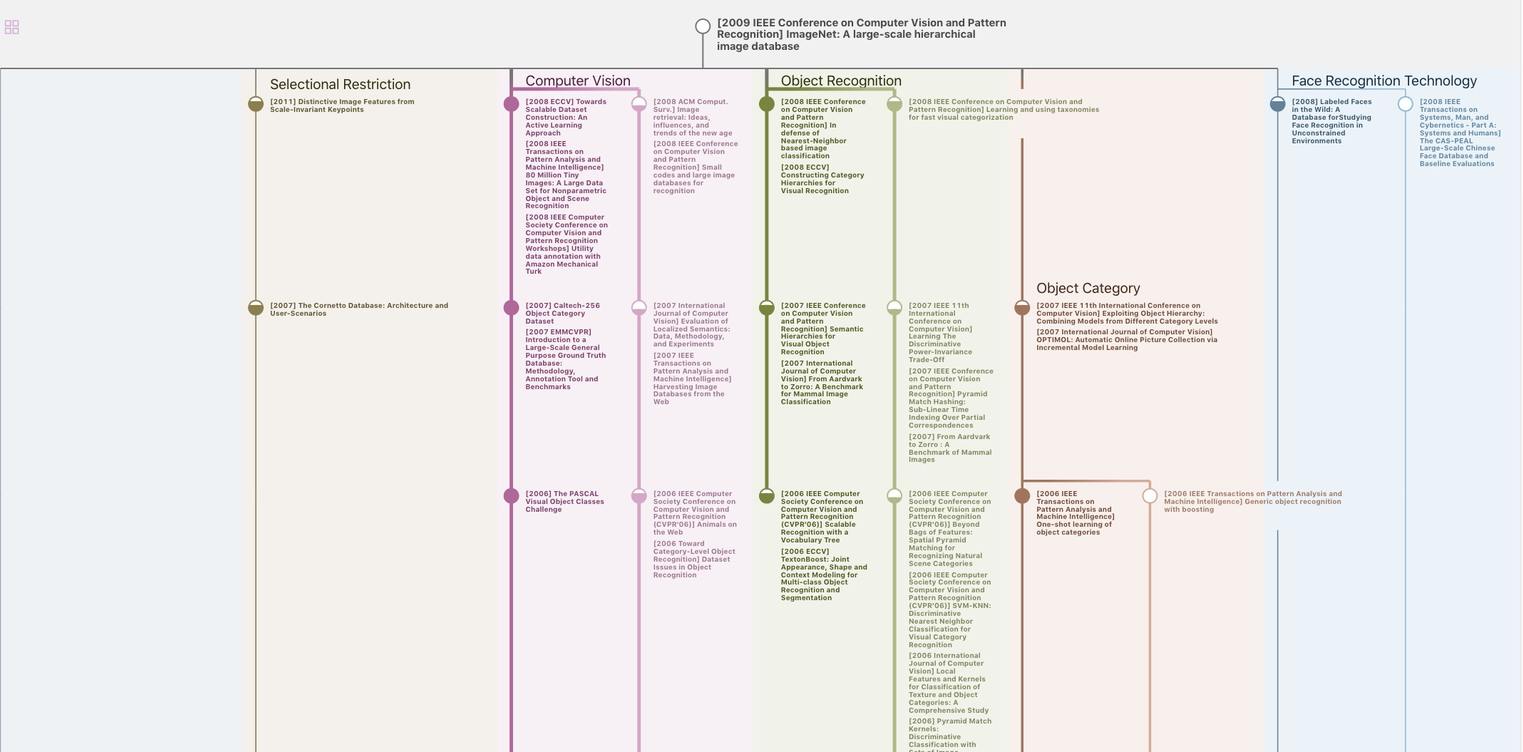
生成溯源树,研究论文发展脉络
Chat Paper
正在生成论文摘要