Exploring iterative dual domain adaptation for neural machine translation
KNOWLEDGE-BASED SYSTEMS(2024)
摘要
Domain adaptation for neural machine translation (NMT) has always been a hot research topic in the community of machine translation. Generally, previous studies focus on the one-pass translation knowledge transfer from single source domain to a target domain, which, however, is unable to fully exploit domain-shared translation knowledge. In this paper, we propose several iterative dual domain adaptation frameworks for NMT, which iteratively perform distillation-based bidirectional translation knowledge transfer (from one domain to another and then vice versa). Specifically, we consider three scenarios of NMT domain adaptation: (1) One-to-one domain adaptation for NMT; (2) Many-to-one domain adaptation for NMT, where the above-mentioned transfer is performed sequentially between the target domain and each source domain in the ascending order of their domain similarities; and (3) Many-to-many domain adaptation for NMT. In this setting, each domain is considered as the target domain, and the translation knowledge of other domains are synchronously exploited to enhance the target-domain NMT model via distillation-based knowledge transfer. Particularly, during this process, impacts of the translation knowledge from other domains mainly depend on their similarities to the target domain. Experimental results and in-depth analyses show that the proposed frameworks significantly outperform commonly-used domain adaptation approaches across several language pairs. We release our code and at Github https://github.com/DeepLearnXMU/JIDDANMT.
更多查看译文
关键词
Machine translation,Domain adaptation,Knowledge distillation
AI 理解论文
溯源树
样例
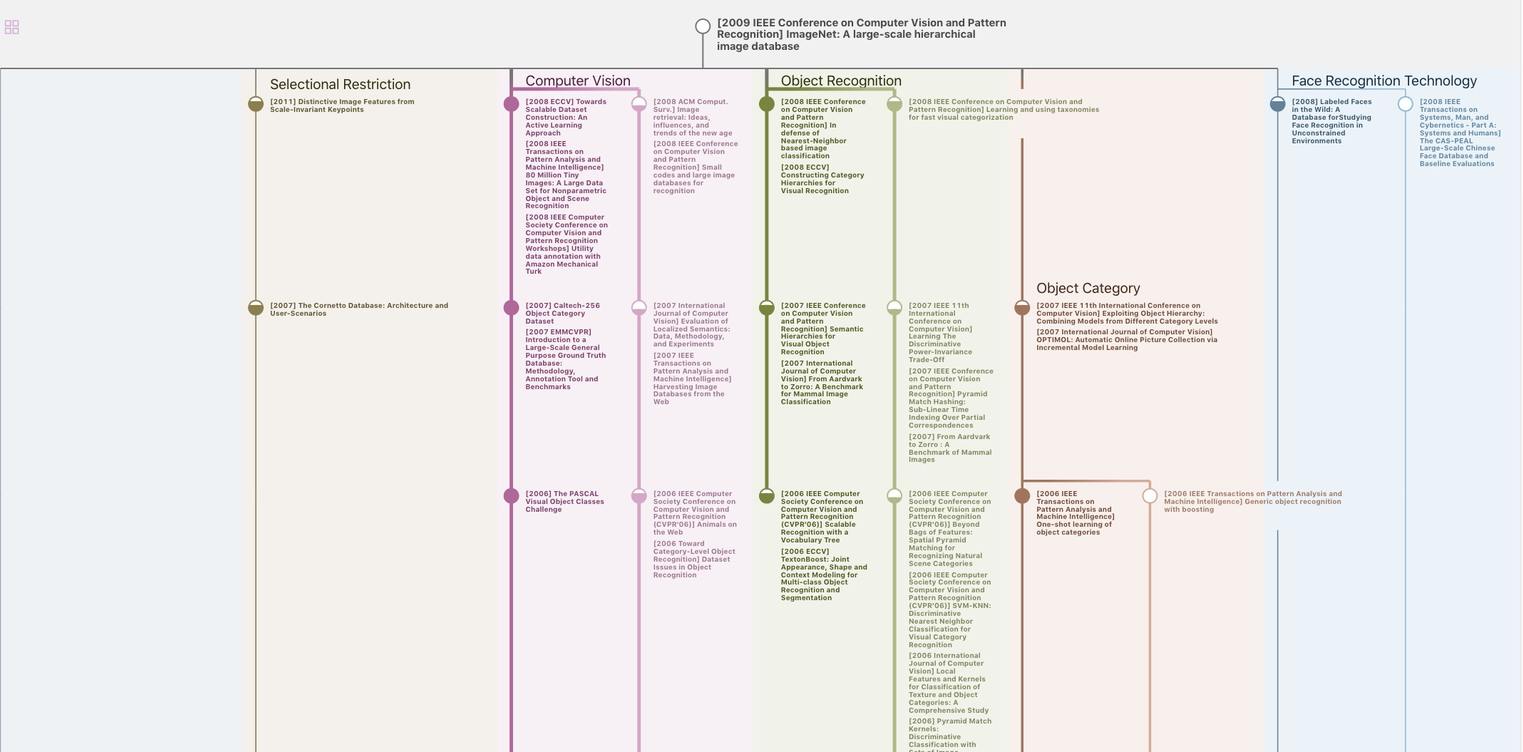
生成溯源树,研究论文发展脉络
Chat Paper
正在生成论文摘要