Outlier detection for partially labeled categorical data based on conditional information entropy
INTERNATIONAL JOURNAL OF APPROXIMATE REASONING(2024)
摘要
Labeling a large amount of data is exceptionally costly and practically infeasible, and thus available data may have missing labels. In this article, we investigate outlier detection for partially labeled categorical data based on conditional information entropy. Firstly, the equivalence class in a partially labeled categorical decision information system (p-CDIS) is introduced, so that the missing labels can be predicted by use of conditional probability. Then, conditional information entropy in a p-CDIS is calculated, which provides a more comprehensive measure of uncertainty. Additionally, the relative information entropy and relative cardinality in a p-CDIS are proposed. Next, the degree of outlierness and the weight function are presented to find outlier factors. Finally, an outlier detection method in a p-CDIS based on conditional information entropy is proposed, and a corresponding conditional information entropy algorithm (CEOF) is designed. To evaluate the stability of the CEOF algorithm, experiments are performed on ten UCI Machine Learning Repository datasets. Compared with five other algorithms, the proposed method is shown to have good effectiveness and adaptability for categorical data.
更多查看译文
关键词
Partially labeled categorical data,Partially labeled categorical decision,information system,Outlier detection,Conditional information entropy
AI 理解论文
溯源树
样例
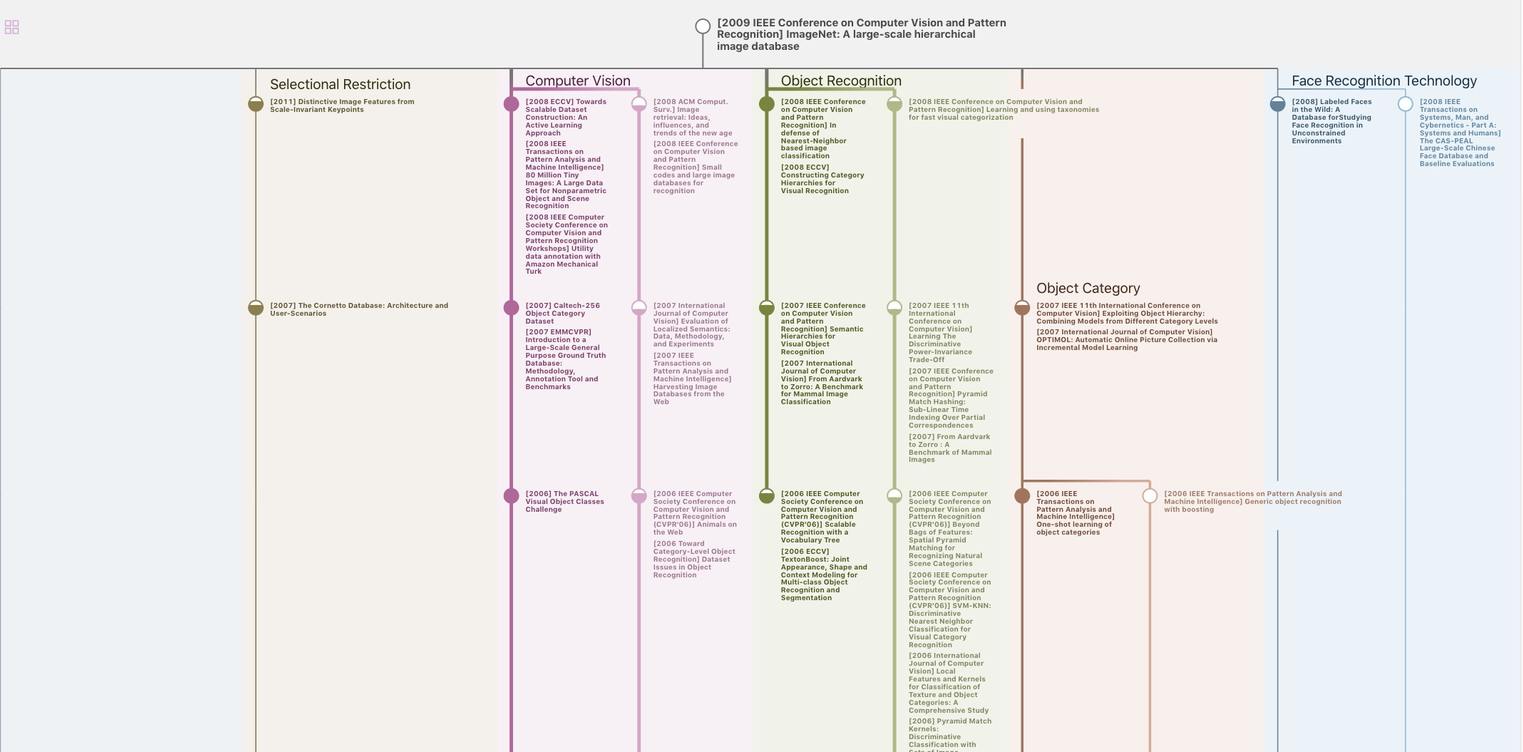
生成溯源树,研究论文发展脉络
Chat Paper
正在生成论文摘要