DQ-HGAN: A heterogeneous graph attention network based deep Q-learning for emotional support conversation generation
KNOWLEDGE-BASED SYSTEMS(2024)
摘要
Emotional Support Conversation (ESC) is widely applied in the fields of intelligent companionship, particularly in psychological health support and customer service. However, there are two significant challenges that require be addressed: dynamically modeling the user's state, encompassing the individual's intention and emotion, and effectively integrating these factors to select the most suitable support strategy. In this paper, a Heterogeneous Graph Attention Network Based Deep Q-learning for Emotional Support Conversation Generation (DQ-HGAN) is proposed to solve these problems. To capture the interaction relationships between user intention, emotion, and historical dialogues more effectively, a heterogeneous graph attention network is constructed based on an intention lexicon and emotion classifier. And a DQN-based algorithm is adopted to direct the response generation, which can learn the optimal response strategy more intelligently than traditional rule-based or heuristic methods. Extensive experiments on the real-world dataset ESConv show that DQ-HGAN outperforms competitive baselines significantly in both automatic and human evaluations. Our codes are available at https://github. com/wumingyao/DQ-HGAN.git.
更多查看译文
关键词
Emotional support conversation (ESC),Heterogeneous graph attention network,Deep Q-learning,User state tracking,Strategy planning
AI 理解论文
溯源树
样例
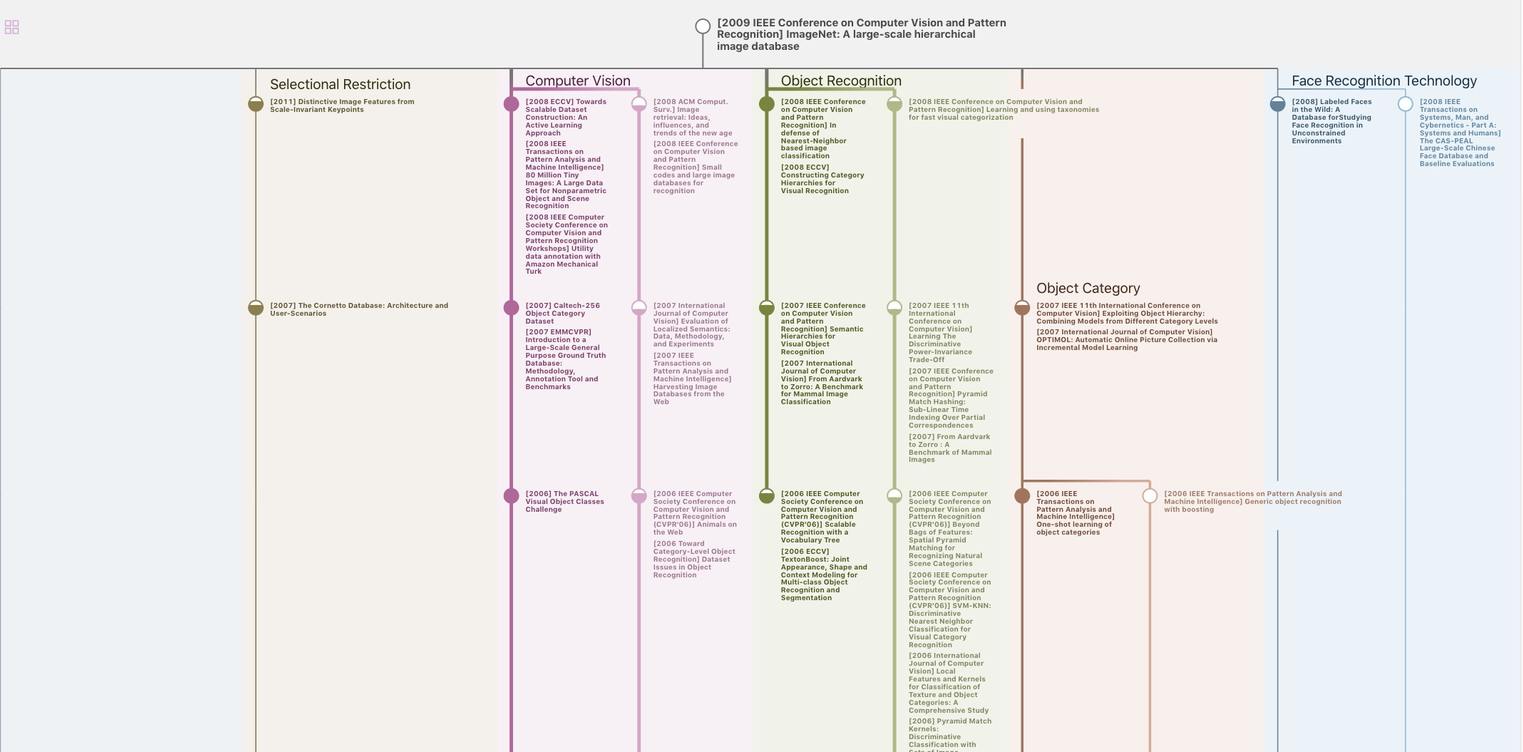
生成溯源树,研究论文发展脉络
Chat Paper
正在生成论文摘要