Language model as an Annotator: Unsupervised context-aware quality phrase generation
KNOWLEDGE-BASED SYSTEMS(2024)
摘要
Phrase mining is a fundamental text mining task that aims to identify quality phrases from context. Nevertheless, the scarcity of extensive gold labels datasets, demanding substantial annotation efforts from experts, renders this task exceptionally challenging. Furthermore, the emerging, infrequent, and domain specific nature of quality phrases present further challenges in dealing with this task. Therefore, in this paper, we propose LMPhrase, a novel unsupervised context-aware quality phrase mining framework built upon large pre-trained language models (LMs). Specifically, we first mine quality phrases as silver labels by employing a parameter-free probing technique called Perturbed Masking on the pre-trained language model BERT (coined as Annotator). In contrast to typical statistic-based or distantly-supervised methods, our silver labels, derived from large pre-trained language models, take into account rich contextual information contained in the LMs. As a result, they bring distinct advantages in preserving informativeness, concordance, and completeness of quality phrases. Secondly, training a discriminative span prediction model heavily relies on massive annotated data and is likely to face the risk of overfitting silver labels. Alternatively, motivated by recent success in formulating language understanding problems such as named entity recognition and sentiment analysis as generation tasks, we formalize phrase tagging task as the sequence generation problem by directly fine-tuning on the Sequence to-Sequence (Seq2Seq) pre-trained language model BART with silver labels (coined as Generator). Finally, we merge the quality phrases from both the Annotator and Generator as the final predictions, considering their complementary nature and distinct characteristics. Extensive experiments show that our LMPhrase consistently outperforms all the existing competitors across two different granularity phrase mining tasks, where each task is tested on two different domain datasets. The promising results show the superiority of our framework with pre-trained language model.
更多查看译文
关键词
Phrase mining,Unsupervised learning,Pre-trained language model,Seq2Seq learning
AI 理解论文
溯源树
样例
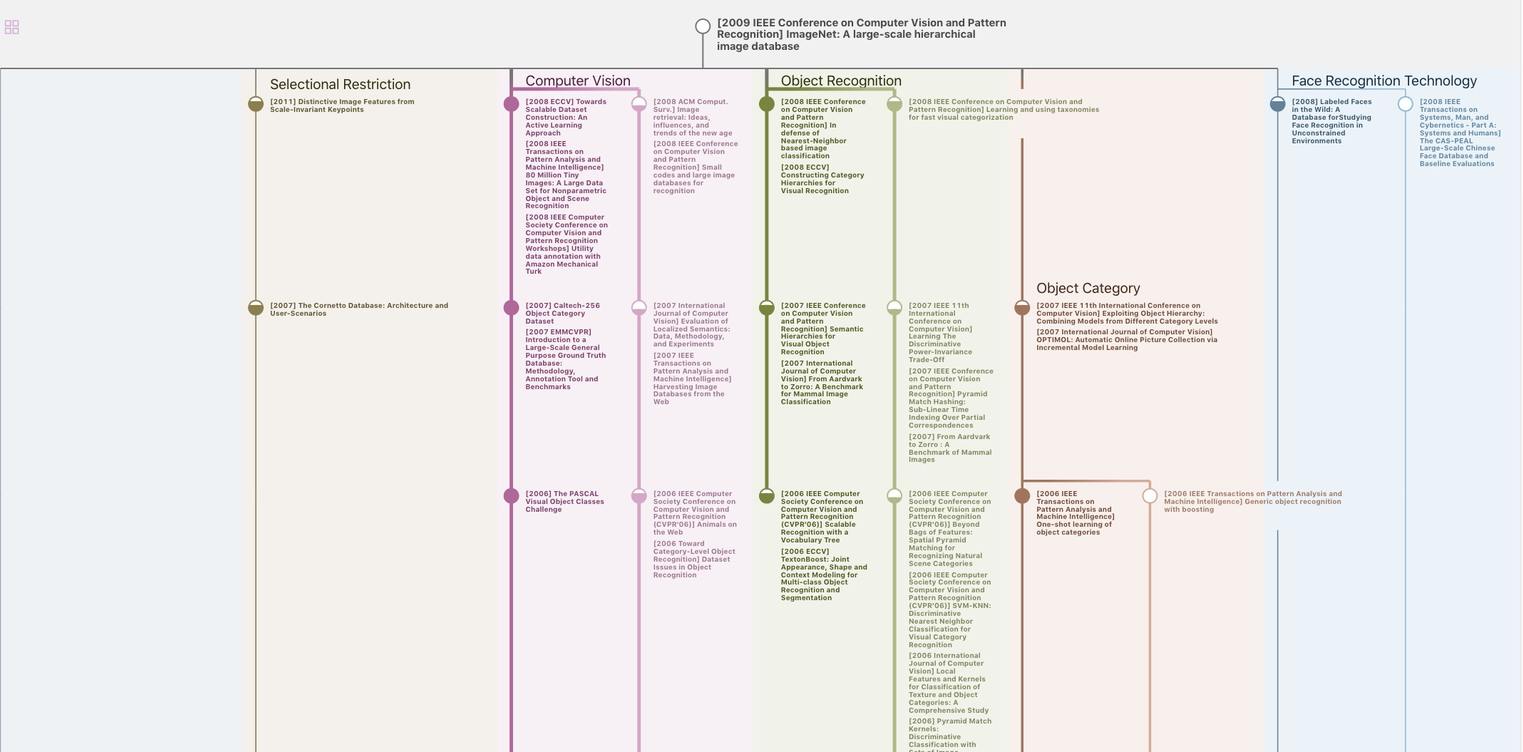
生成溯源树,研究论文发展脉络
Chat Paper
正在生成论文摘要