Self-supervised rotation-equivariant spherical vector network for learning canonical 3D point cloud orientation
ENGINEERING APPLICATIONS OF ARTIFICIAL INTELLIGENCE(2024)
摘要
The perception of orientation in augmented reality, robot grasping, and 3D scene understanding is commonly addressed through the utilization of hand-crafted geometric features. However, machines can also learn the inherent orientation of 3D point clouds through experience, similar to humans. In this paper, we propose a self-supervised spherical vector network that is rotation-equivariant. Specifically, we use density-aware adaptive sampling to construct spherical signal samples to handle distorted point distributions in spherical space. Spherical convolutional vector layers and spherical routing layers are proposed to extract the rotation-equivariant vectors that represent the existence probability of the entity and orientations. Our method learns rotational representations from 3D point clouds through a self-supervised training process. We also provide theoretical proof that our proposed spherical vector networks are rotation-equivariant. Experiments on a variety of public datasets directly and indirectly demonstrate the effectiveness of the proposed method for canonical orientation estimation, even on unknown classes.
更多查看译文
关键词
3D point clouds,Self-supervised,Vector networks,Rotation-equivariant,Spherical CNNs
AI 理解论文
溯源树
样例
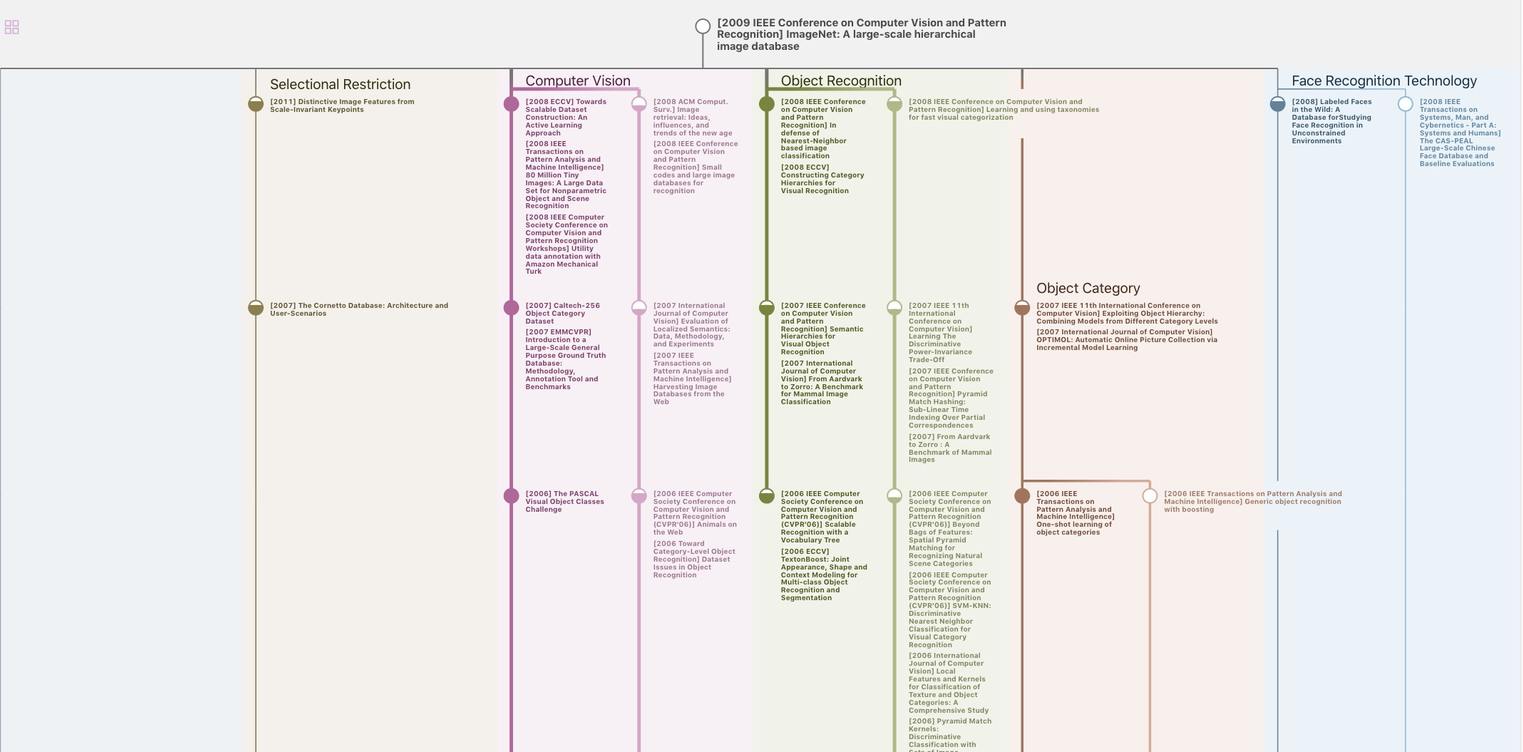
生成溯源树,研究论文发展脉络
Chat Paper
正在生成论文摘要