An efficient segmentation method based on semi-supervised learning for seafloor monitoring in Pujada Bay, Philippines
ECOLOGICAL INFORMATICS(2023)
摘要
The semantic segmentation of marine images makes it easier to describe seafloor scenes and track marine organisms. However, creating human-annotated datasets for image segmentation requires significant time and effort. Therefore, this study proposes a semisupervised learning approach that combines the mean-teacher model and U-Net architecture for segmenting seafloor images obtained from the Philippines. The proposed method performs segmentation for categories, including corals, sea urchins, sea stars, and seagrass. Traditional manual labelling methods are used for coral, sea urchins, and sea stars. For the seagrass category, which is challenging to label manually, we used the K-means clustering algorithm to obtain corresponding labelled datasets based on the characteristics of such images and evaluated the feasibility. Compared with the U-Net-based supervised method, the semi-supervised method used in this study achieved good results and accuracy values, even with fewer labelled images.
更多查看译文
关键词
Coral,Semi-supervised learning,Semantic segmentation,Seafloor images,Marine organism,Deep learning
AI 理解论文
溯源树
样例
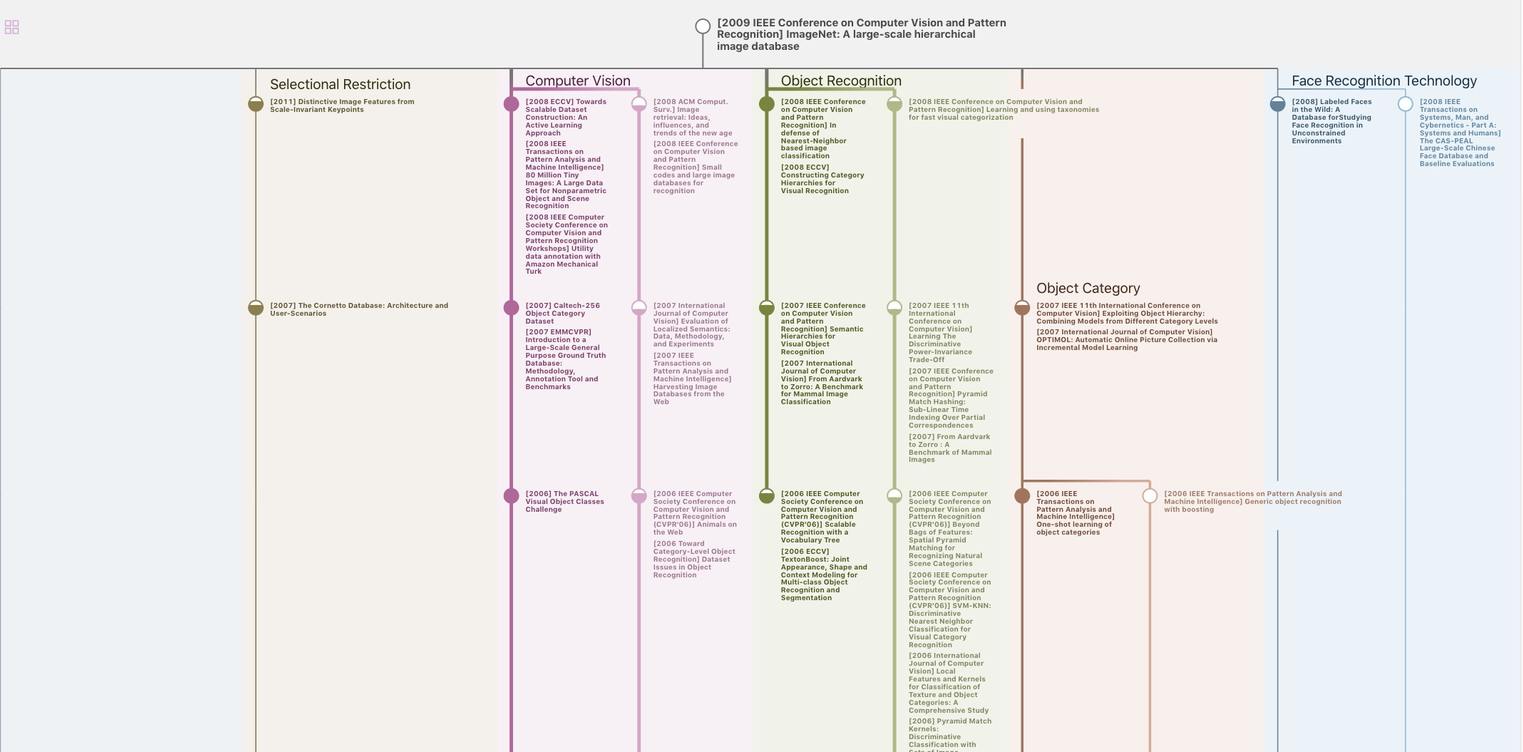
生成溯源树,研究论文发展脉络
Chat Paper
正在生成论文摘要