Supervised kernel principal component analysis for forecasting
FINANCE RESEARCH LETTERS(2023)
摘要
Principal component analysis (PCA) is a versatile tool for dimension reduction with many applications in finance, economics, and machine learning. This paper proposes a new approach to improving the forecasting ability of the Principal Components (PCs) of observed high-dimensional predictors in a factor-augmented forecasting framework. The approach is carried out in a three-step procedure, where we first perform a nonparametric kernel regression of the target variable on each predictor to obtain a new high-dimensional predictor vector formed by stacking all estimated kernel regression functions, we then extract PCs from these new predictors using PCA, and finally, we employ the extracted PCs as predictors to forecast the target variable. A real example on macroeconomic forecasting is analyzed and numerical results show that the proposed kernel PCA can outperform some commonly used forecasting approaches in out-of-sample prediction.
更多查看译文
关键词
Forecasting,Nonparametric regression,Principal component analysis,Dimension reduction
AI 理解论文
溯源树
样例
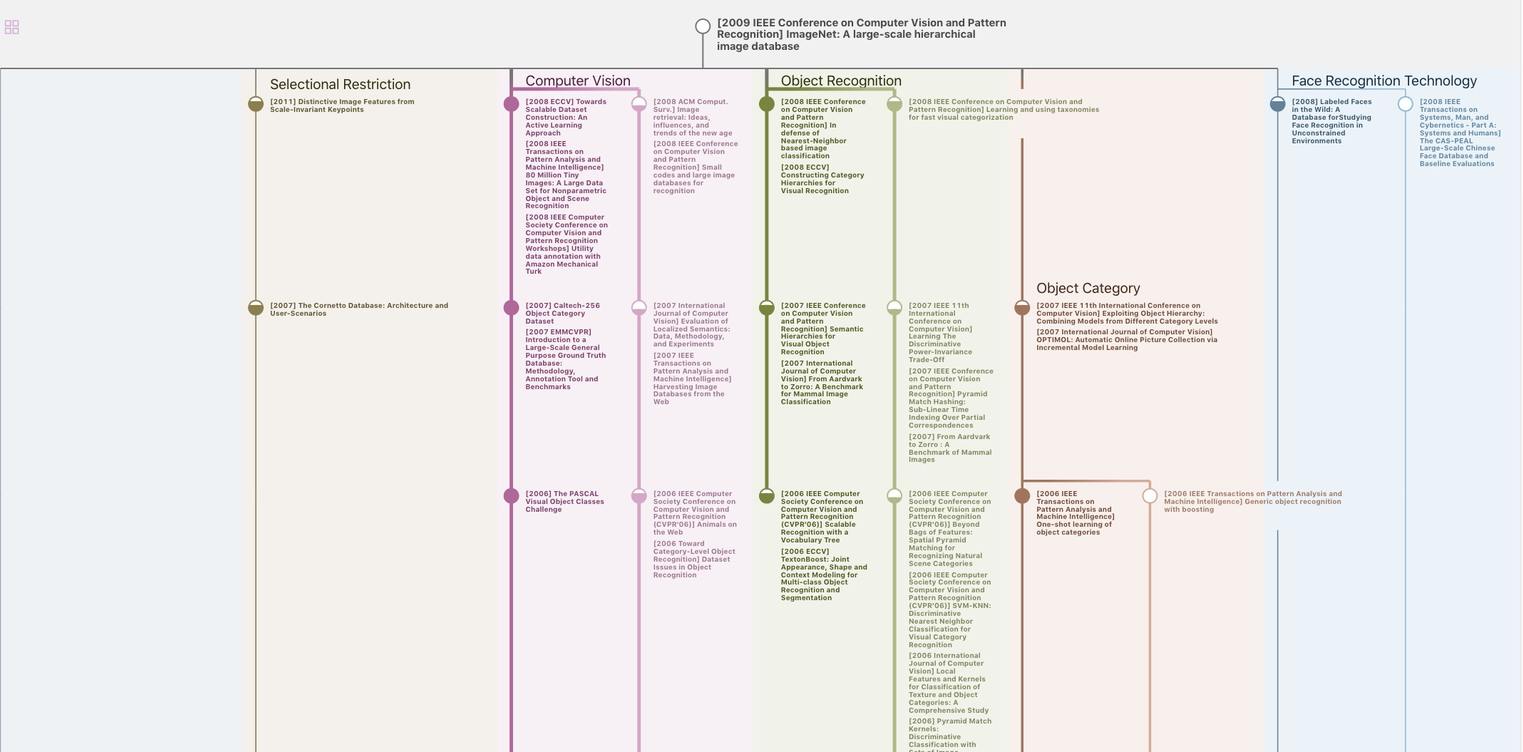
生成溯源树,研究论文发展脉络
Chat Paper
正在生成论文摘要