Novel framework based on ensemble classification and secure feature extraction for COVID-19 critical health prediction
Engineering Applications of Artificial Intelligence(2023)
摘要
The Covid outbreak necessitated the use of an automated method for treating patients with critical symptoms. Increasing use of the Internet of Things (IoT) and smart devices requires access to real-time data over robust and secure network infrastructures. During the lifetime of healthcare applications, the data must be transferred in a controlled manner. In this context, this study proposes, an IoT-based decision support system for the analysis of Covid-related data in healthcare systems. Through in-vehicle monitoring, it is intended to safely send important data and patient updates to attending physicians. The suggested approach uses feature extraction methods to identify patients' crucial states while protecting their private information. To improve feature extraction, two techniques are combined: ensemble classification and cipher substitution for secure Json Web Tokens (JWTs). By incorporating the Secure Hashing Algorithm-2 (SHA-2) methods into the Feature Extraction based Logistic Regression (FELR), security protections are substantially reinforced. The system uses the Message Queuing Telemetry Transport (MQTT) protocol to provide secure communication between devices and access to the extracted characteristics from rescue vehicles. The system analyzes passengers, traffic data, and medical issues, which are continually updated with cloud-based security, by using vehicle-based communication networks. The secure movement of patient data inside the hospital network is ensured by this cloud-based system. By using sophisticated regression analysis and ensemble learning approaches, our suggested methodology has shown significant advantages compared to traditional models such as Logistic Regression (LR) and Mixed Regression (MR). Our technique demonstrates exceptional performance with an accuracy rate of 96%, a recall rate of 91%, and an F-measure of 91%. We also precisely compared the performance of our proposed FELR algorithm with hybrid prediction model using deep learning, the extra tree convolutional neural network-based ensemble model, ensemble-based random forest, ensemble transformation learning, and machine learning with regression. Significantly, FELR algorithm has demonstrated exceptional superiority by consistently surpassing the predictive capabilities of all these algorithms. The system has exceptional performance and exhibits improved efficiency and secure transmission capabilities. The unique model that we propose offers a feasible and effective approach for detecting crucial states in patients within healthcare facilities. The success of our framework is shown via a well-planned approach that includes important processes such as data preprocessing, feature extraction, ensemble model construction, and evaluation. The proposed framework offers a promising avenue for aiding healthcare professionals in making informed decisions regarding the critical health trajectories of COVID-19 patients.
更多查看译文
关键词
ensemble classification,secure feature extraction,feature extraction
AI 理解论文
溯源树
样例
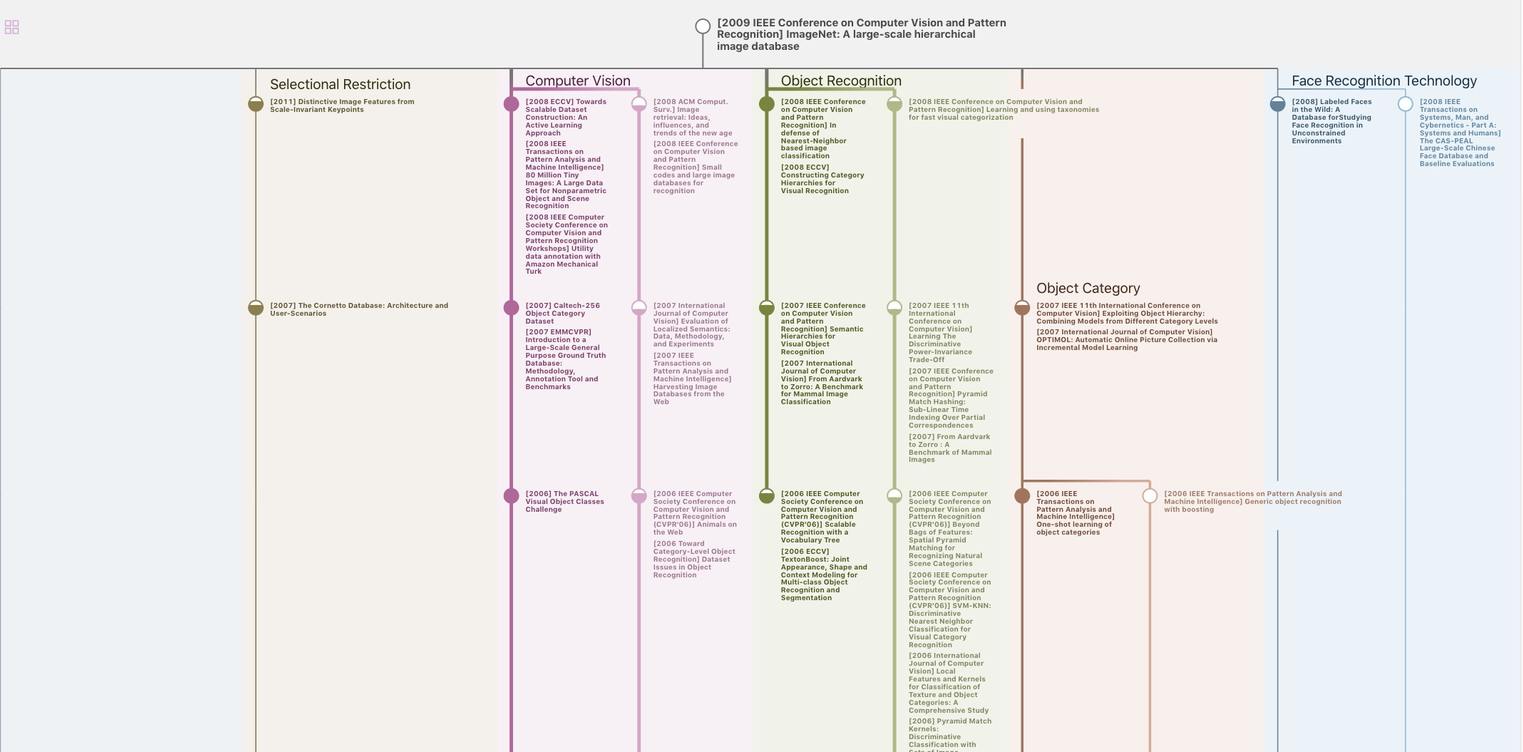
生成溯源树,研究论文发展脉络
Chat Paper
正在生成论文摘要