CrossFuse: A novel cross attention mechanism based infrared and visible image fusion approach
INFORMATION FUSION(2024)
摘要
Multimodal visual information fusion aims to integrate the multi-sensor data into a single image which contains more complementary information and less redundant features. However the complementary information is hard to extract, especially for infrared and visible images which contain big similarity gap between these two modalities. The common cross attention modules only consider the correlation, on the contrary, image fusion tasks need focus on complementarity (uncorrelation). Hence, in this paper, a novel cross attention mechanism (CAM) is proposed to enhance the complementary information. Furthermore, a two-stage training strategy based fusion scheme is presented to generate the fused images. For the first stage, two auto-encoder networks with same architecture are trained for each modality. Then, with the fixed encoders, the CAM and a decoder are trained in the second stage. With the trained CAM, features extracted from two modalities are integrated into one fused feature in which the complementary information is enhanced and the redundant features are reduced. Finally, the fused image can be generated by the trained decoder. The experimental results illustrate that our proposed fusion method obtains the SOTA fusion performance compared with the existing fusion networks. The codes of our fusion method will be available soon.
更多查看译文
关键词
Image fusion,Transformer,Cross attention,Infrared image,Visible image
AI 理解论文
溯源树
样例
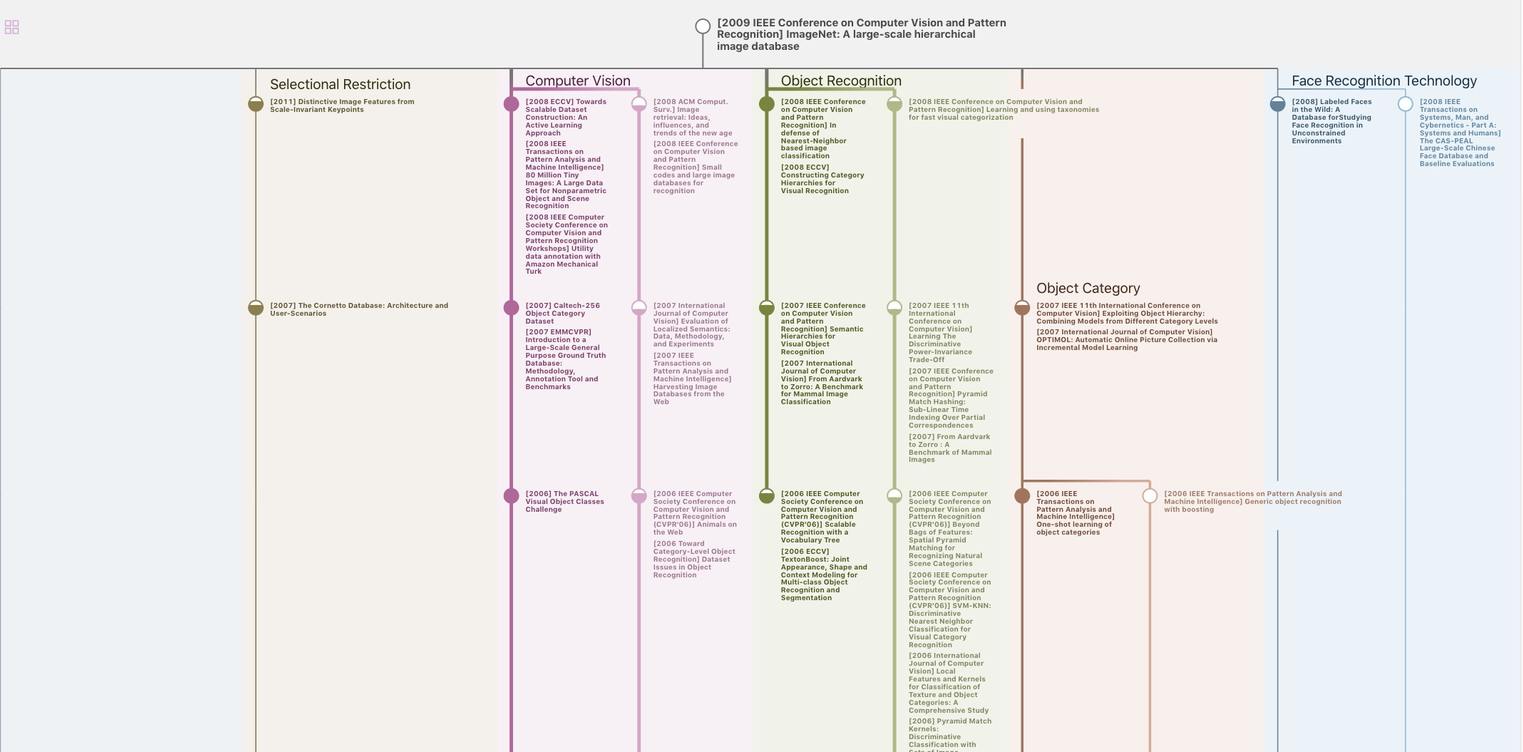
生成溯源树,研究论文发展脉络
Chat Paper
正在生成论文摘要