Deep learning-based motion compensation for automotive SAR imaging
MEASUREMENT(2024)
摘要
In this paper, we propose an unsupervised image -to -image translation (UNIT) network as a deep learningbased motion compensation method to compensate for phase errors that may occur when acquiring data from a synthetic aperture radar (SAR) system. In general, in the SAR system measurement process, a problem arises in obtaining data containing phase errors due to the platform's non -ideal path or unstable pose. Therefore, we propose a deep learning -based motion compensation method and verify the performance of the proposed method through an experiment using an automotive radar. As a result of comparing the performance with existing motion compensation methods based on actual measurement data, the proposed UNIT network showed 8.68% higher peak signal-to-noise ratio (PSNR) and 11.66% higher structural similarity index measure (SSIM) performance than phase gradient autofocus, and 7.98% higher PSNR and 10.82% higher SSIM performance than minimum entropy autofocus.
更多查看译文
关键词
Automotive radar,Motion compensation,Synthetic aperture radar,Unsupervised image-to-image translation
AI 理解论文
溯源树
样例
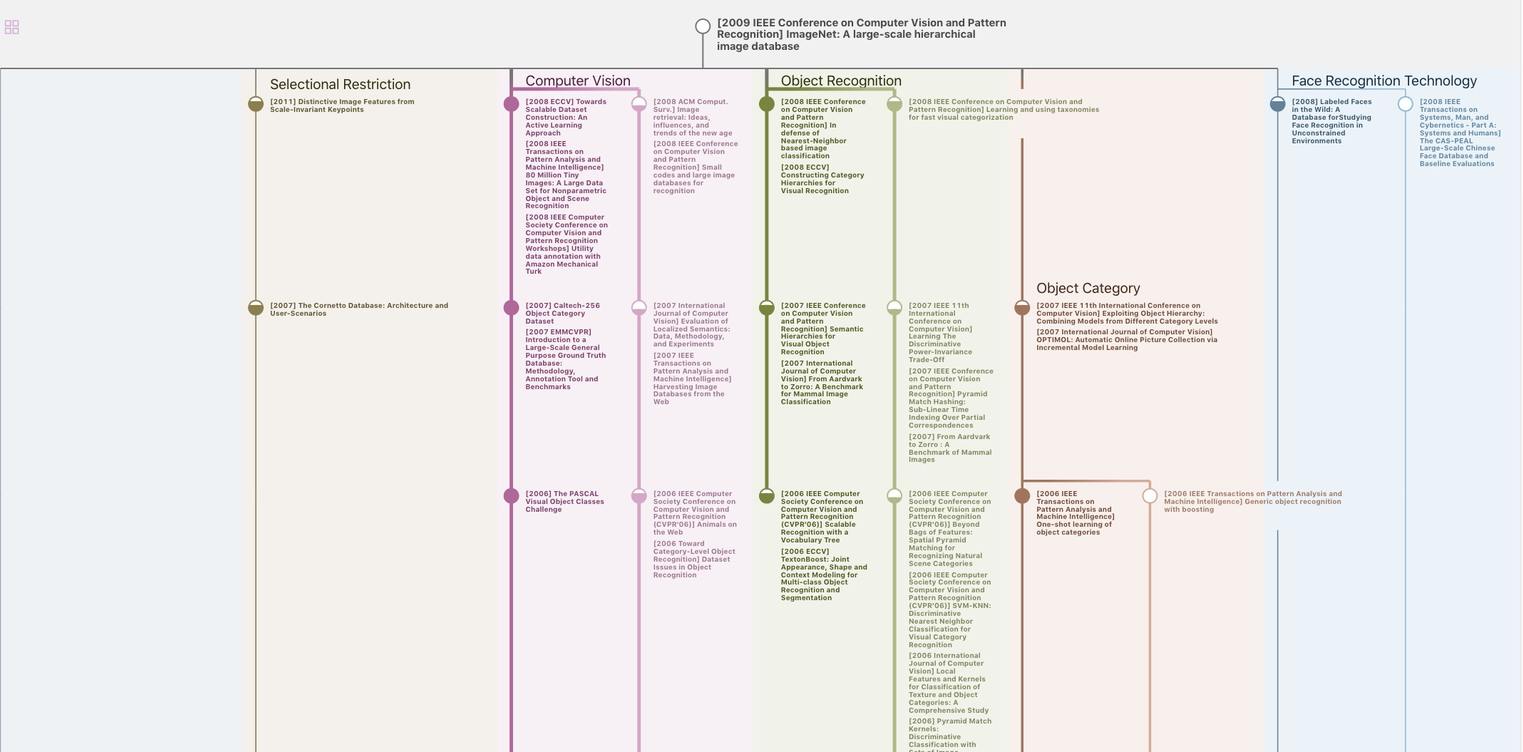
生成溯源树,研究论文发展脉络
Chat Paper
正在生成论文摘要