Self-supervised learning minimax entropy domain adaptation for the underwater target recognition
APPLIED ACOUSTICS(2024)
摘要
With wide research of intelligent methods, studies on underwater target recognition have been making rapid progress. However, various marine conditions may cause data distribution mismatch between the collected signal sets, reducing model recognition performance. To mitigate the negative impact of data divergence, this paper uses the domain adaptation methods in target recognition and proposes an improved domain adaptation frame, self-supervised learning minimax entropy. Firstly, based on the minimax entropy method (MME), the prediction consistency is utilized to determine pseudo-labels, and the loss weight is introduced to deal with the misaligned target domain data. Then, a self-supervised learning mechanism is designed to ensure consistency of prediction results during training. Three different features, including the constant-Q transform (CQT), Mel spectrum, and Mel-frequency cepstral coefficient (MFCC), are used to verify the performance of domain adaptation methods. The experimental results show that applying domain adaptations can effectively improve the recognition performance of the models under most experimental conditions, and the improved frame has higher average recognition accuracy than other domain adaptation methods in the experiments.
更多查看译文
关键词
Underwater target recognition,Deep learning,Domain adaptation,Self-supervised learning mechanism
AI 理解论文
溯源树
样例
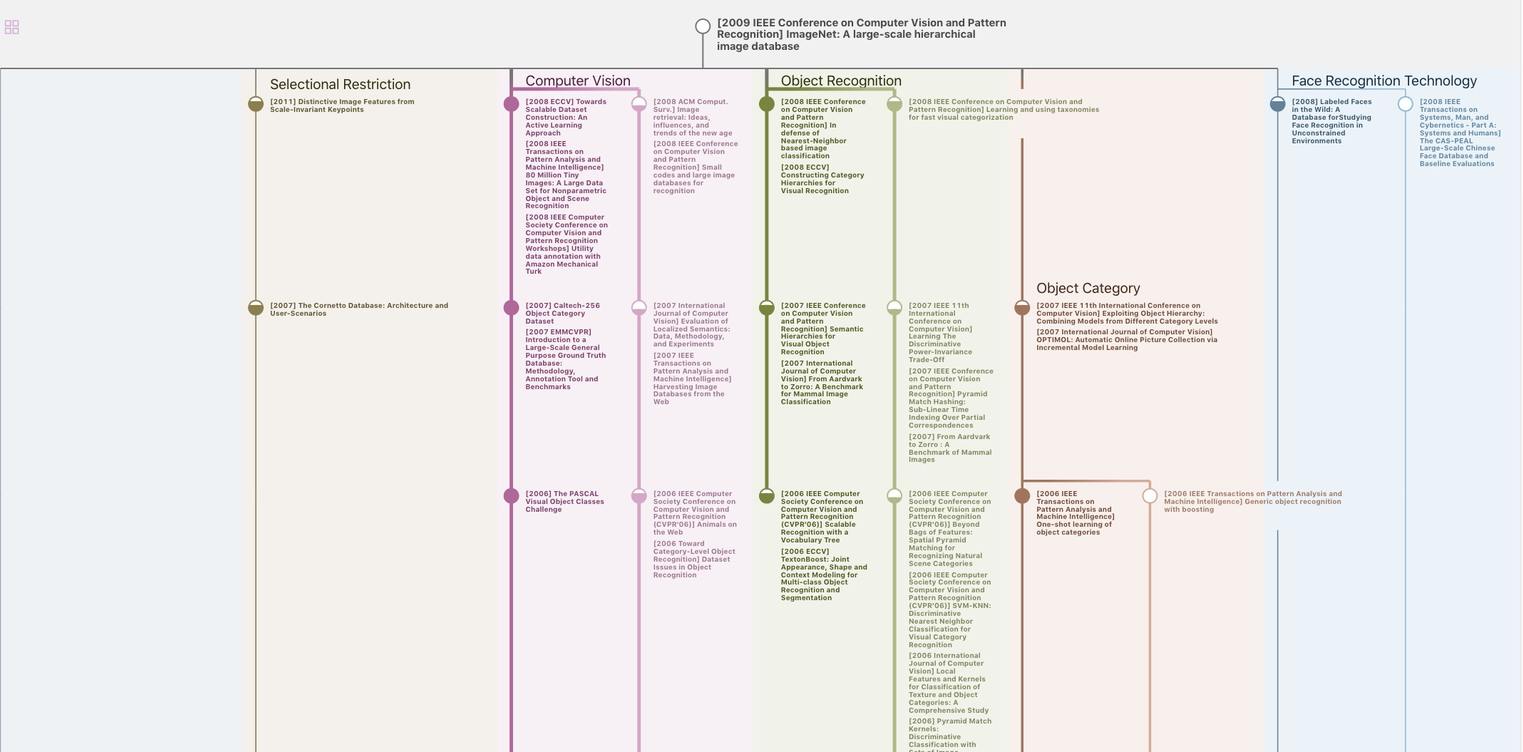
生成溯源树,研究论文发展脉络
Chat Paper
正在生成论文摘要