Integrated Spatio-Temporal Deep Clustering (ISTDC) for cognitive workload assessment
BIOMEDICAL SIGNAL PROCESSING AND CONTROL(2024)
摘要
Traditional high-dimensional electroencephalography (EEG) features (spectral or temporal) may not always attain satisfactory results in cognitive workload estimation. In contrast, deep representation learning (DRL) transforms high-dimensional data into cluster-friendly low-dimensional feature space. Therefore, this paper proposes an Integrated Spatio-Temporal Deep Clustering (ISTDC) model that uses DRL followed by a clustering method to achieve better clustering performance. The proposed model is illustrated using four Algorithms and Variational Bayesian Gaussian Mixture Model (VBGMM) clustering method. Temporal and spatial Variational Auto Encoder (VAE) models (mentioned in Algorithm 2 and Algorithm 3) learn temporal and spatial latent features from sequence-wise EEG signals and scalp topographical maps using the Long short-term memory and Convolutional Neural Network models. The concatenated spatio-temporal latent feature (mentioned in Algorithm 4) is passed to the VBGMM clustering method to efficiently estimate workload levels of n-back task. For the 0-back vs. 2-back task, the proposed model achieves the maximum mean clustering accuracy of 98.0%, and it improves by 11.0% over the state-of-the-art method. The results also indicate that the proposed multimodal approach outperforms temporal and spatial latent feature-based unimodal models in workload assessment.
更多查看译文
关键词
Cognitive workload,Electroencephalography,Deep clustering,Variational bayesian gaussian mixture model
AI 理解论文
溯源树
样例
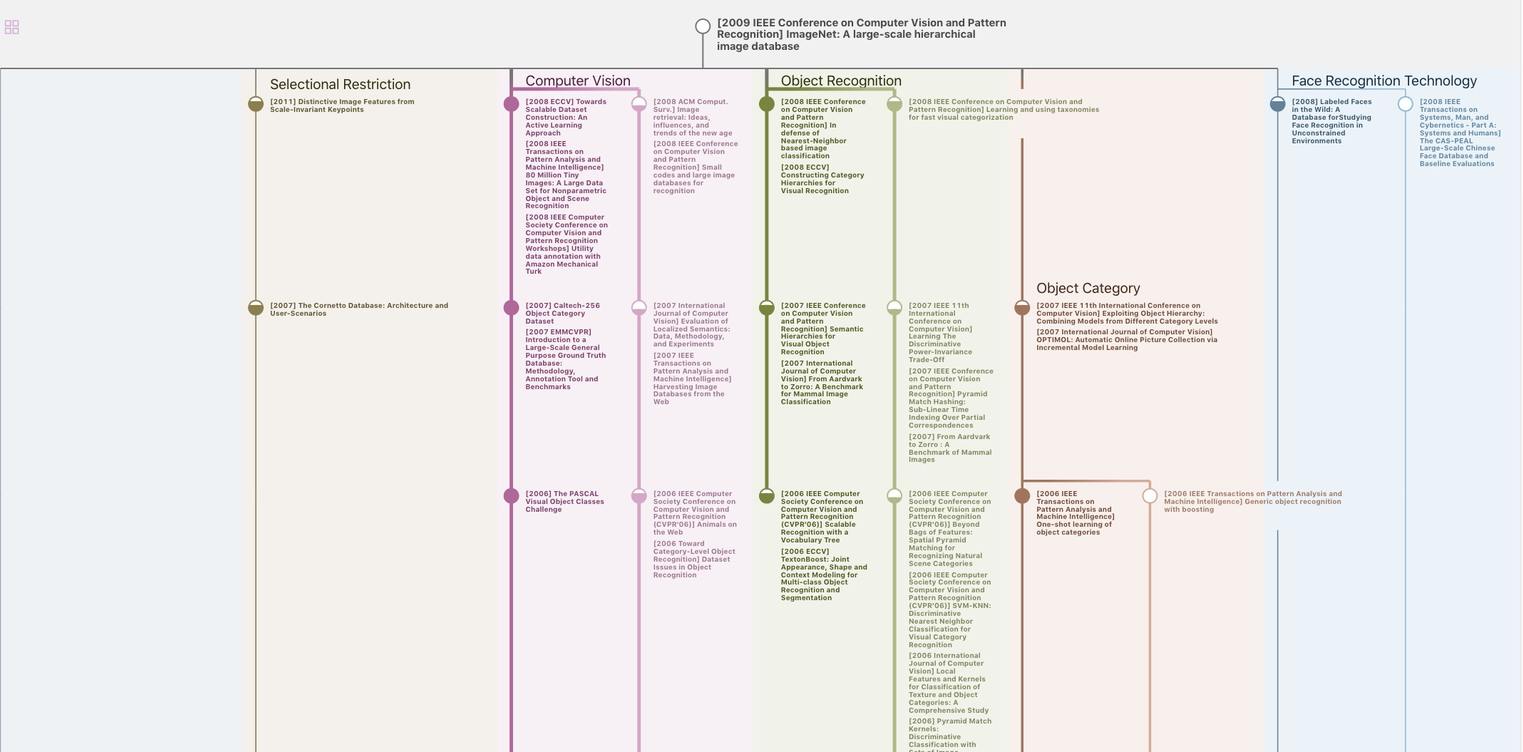
生成溯源树,研究论文发展脉络
Chat Paper
正在生成论文摘要