JoyPose: Jointly learning evolutionary data augmentation and anatomy-aware global-local representation for 3D human pose estimation
PATTERN RECOGNITION(2024)
摘要
Video -based 3D human pose estimation is an important yet challenging task for many human -involved pattern recognition systems. Existing deep learning -based 3D human pose estimation methods are faced with problems of lacking large-scale training data and lacking effective solutions to represent the complicated human body structure. To this end, this paper proposes a jointly learning framework entitled JoyPose that simultaneously leverages both human pose data augmentation and human pose estimation. In particular, JoyPose consists of an evolutionary data augmentation module and an anatomy -aware global-local pose feature representation module for 3D human pose estimation. The evolution for data augmentation is guided by reinforcement learning strategy in a probabilistic way according to pose estimation loss. The anatomy -aware global-local pose feature representation module separately captures global features and local features according to anatomical and kinematic patterns observed from pose estimation errors across different human joints. The performance of the final human pose estimation is leveraged by both data augmentation and anatomy aware global-local feature representation. Extensive experiments on three real -world datasets demonstrate superiority and robustness against state-of-the-art methods.
更多查看译文
关键词
3D human pose estimation,Evolutionary data augmentation,Global-local representation,Anatomy-awareness,Joint optimization
AI 理解论文
溯源树
样例
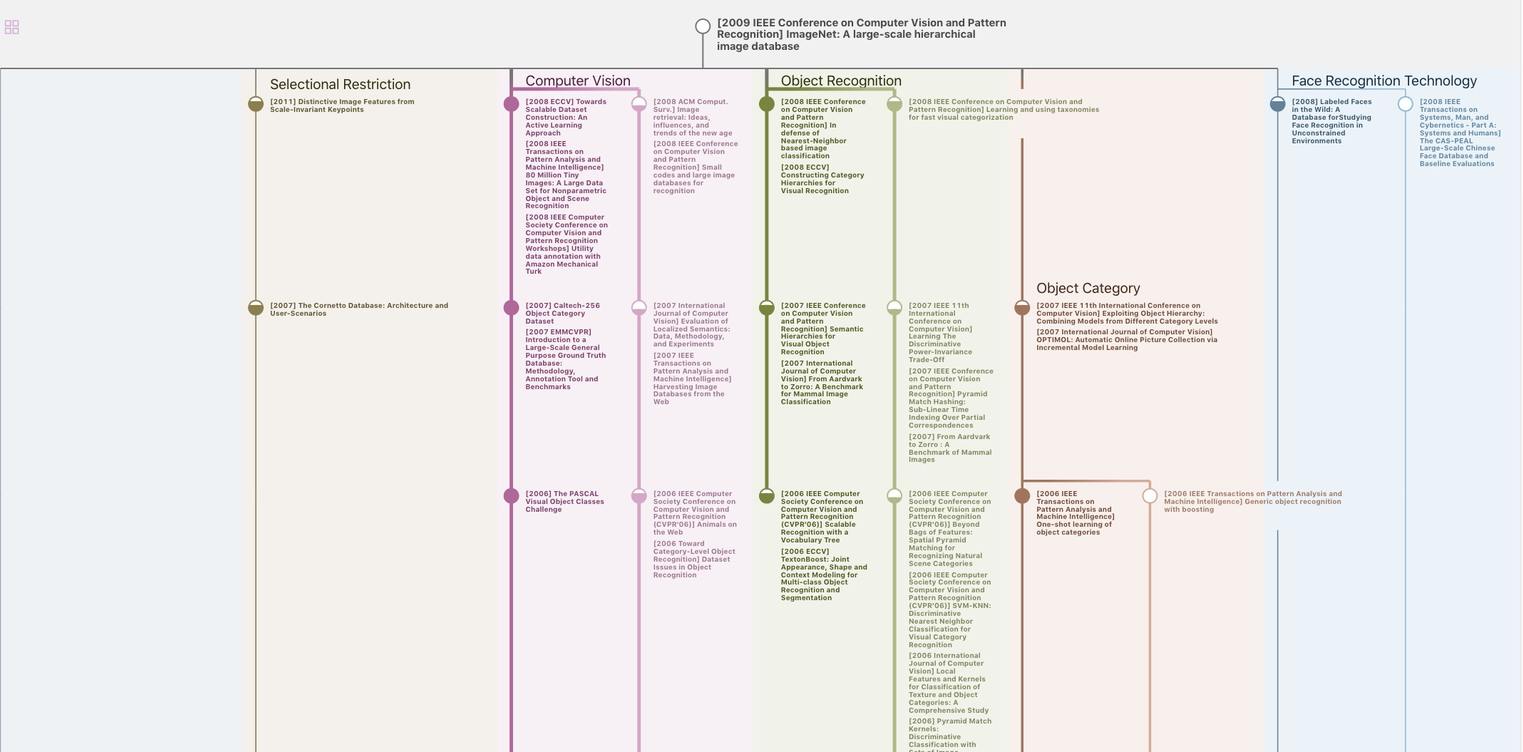
生成溯源树,研究论文发展脉络
Chat Paper
正在生成论文摘要