Unsupervised industrial anomaly detection with diffusion models
JOURNAL OF VISUAL COMMUNICATION AND IMAGE REPRESENTATION(2023)
摘要
Due to the limitations of autoencoders and generative adversarial networks, the performance of reconstruction -based unsupervised image anomaly detection methods are not satisfactory. In this paper, we aim to explore the potential of a more powerful generative model, the diffusion model, in the anomaly detection problem. Specifically, we design a Reconstructed Diffusion Models (RecDMs) based on conditional denoising diffusion implicit models for image reconstruction. To eliminate the stochastic nature of the generation process, our key idea is to use a learnable encoder to extract meaningful semantic representations, which are then used as signal conditions in an iterative denoising process to guide the model in recovering the image, while avoiding falling into an "identical shortcut" to meaningless image reconstruction. To accurately locate anomaly regions, we introduce a discriminative network to obtain the pixel-level anomaly segmentation map based on the reconstructed image. Our experiments demonstrate the effectiveness of the proposed method, achieving a new state-of-the-art image-level AUC score of 98.1% and a pixel-level AUC score of 94.6% on the MVTec AD dataset, among all reconstruction-based methods. We also show the significant potential and promising future of our method on the challenging real-world dataset, the CHL AD dataset.
更多查看译文
关键词
Anomaly detection,Diffusion model,Image reconstruction,Unsupervised learning
AI 理解论文
溯源树
样例
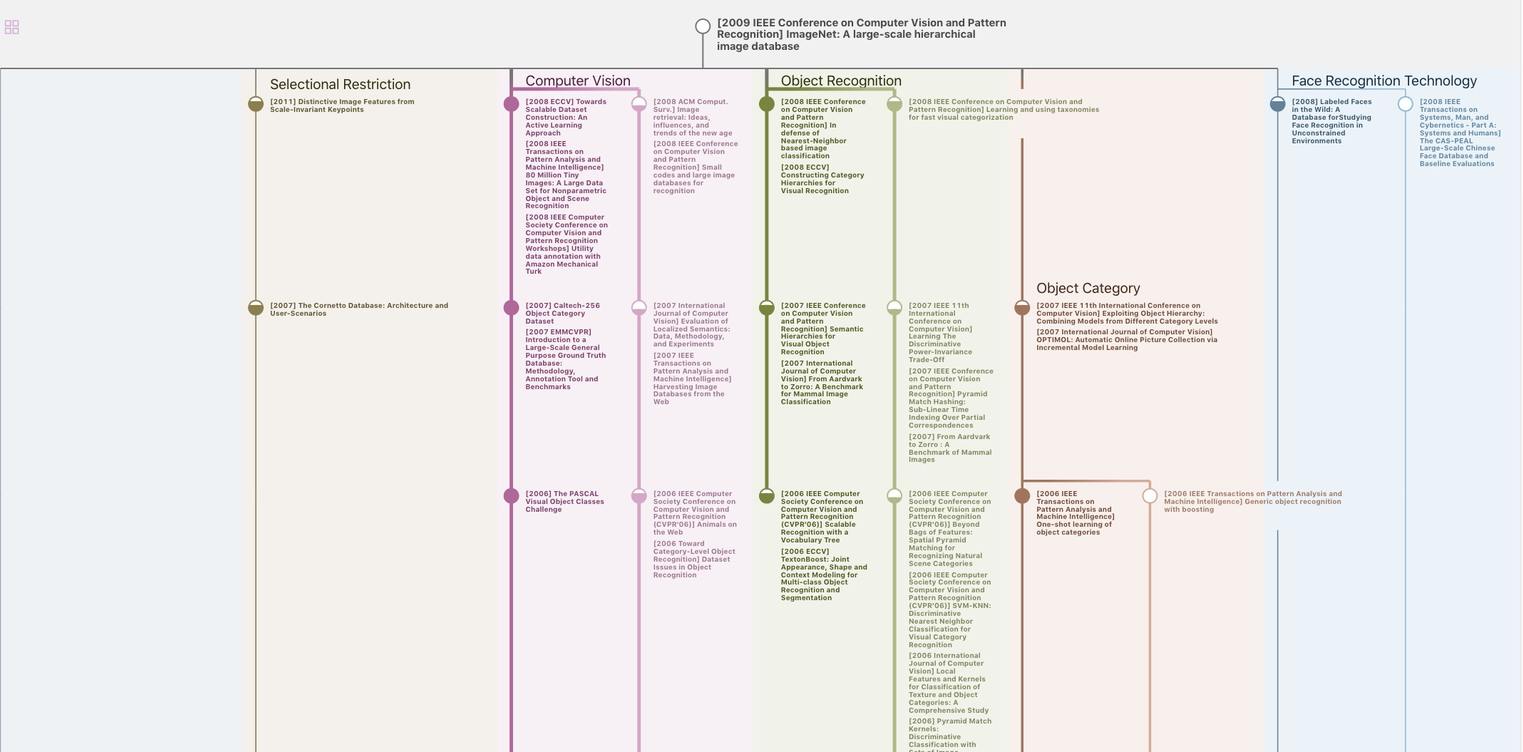
生成溯源树,研究论文发展脉络
Chat Paper
正在生成论文摘要