Cross-patient automatic epileptic seizure detection using patient-adversarial neural networks with spatio-temporal EEG augmentation
BIOMEDICAL SIGNAL PROCESSING AND CONTROL(2024)
摘要
Cross-patient automatic epileptic seizure detection through electroencephalogram (EEG) is significant for clinical application and research. However, most automatic seizure detection methods are patient-specific and have poor generalization ability to unseen patients. In this paper, we consider two aspects for solving the cross-patient generalization problem in deep learning approaches. Firstly, we propose a new data augmentation method to largely improve generalization. We analyze the statistical distribution of seizure EEG signals and propose spatiotemporal EEG augmentation (STEA) to generate synthetic training seizure data with spatiotemporal dependencies in EEG. Secondly, we propose patient-adversarial neural network (PANN) to learn a patient-invariant representation for better generalization. We add a discriminator to distinguish the identity of patients and perform adversarial optimization between the feature extractor and identity discriminator, so that only shared seizure features are maintained. We conduct experiments on both public and clinical EEG datasets with various settings of different window lengths and divisions of training and testing sets. Our model significantly improves the performance of cross-patient detection compared with other methods and achieves state-of-the-art performance. Particularly, our proposed method achieves up to 95% sensitivity on CHB-MIT under 5 s window length segments, and achieves about 85% AUC and sensitivity even on the clinical dataset. Our designed methods can enable detection models extended to unseen patients, with performance achieving the clinical application standards.
更多查看译文
关键词
Automatic epileptic seizure detection,Cross-patient,Deep learning,EEG data augmentation,Patient-adversarial neural networks
AI 理解论文
溯源树
样例
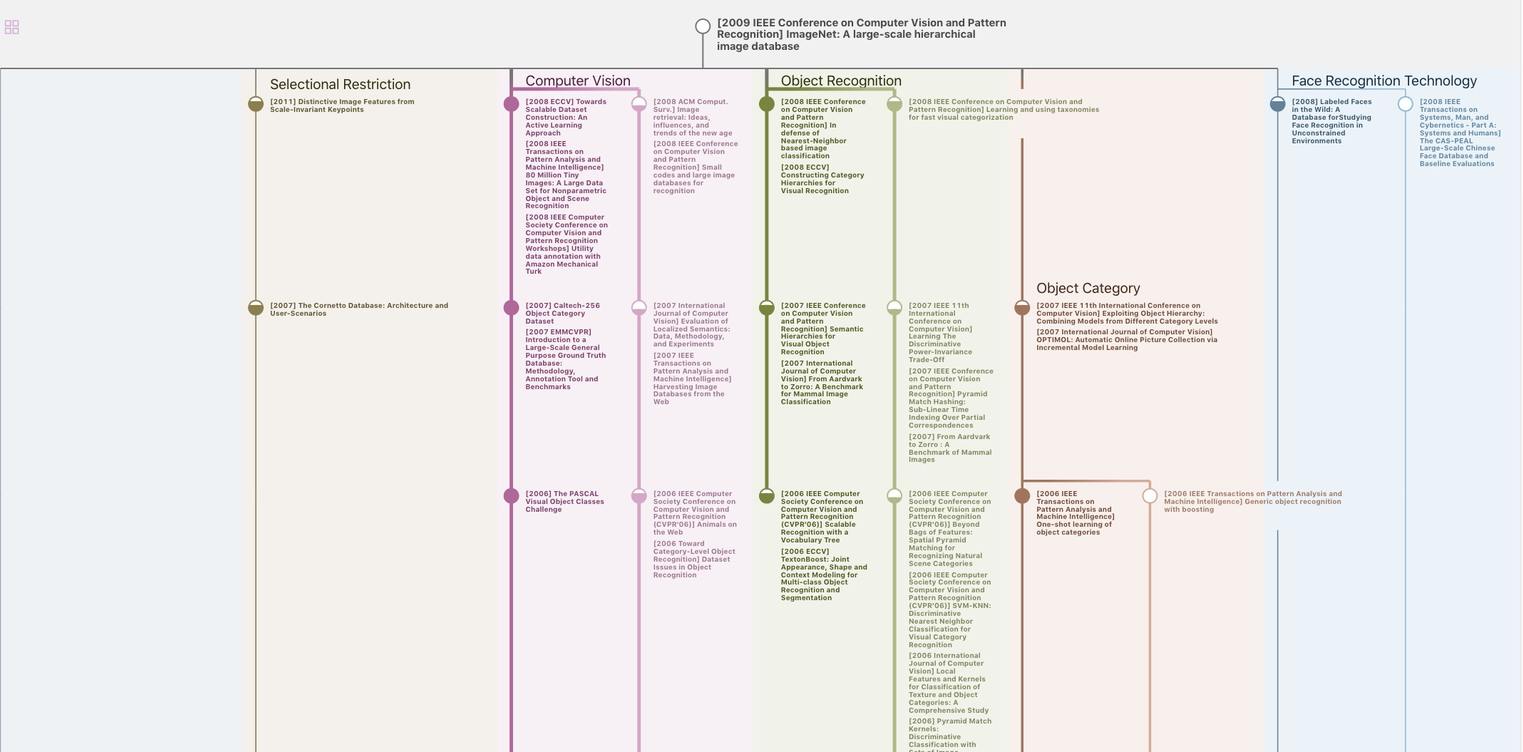
生成溯源树,研究论文发展脉络
Chat Paper
正在生成论文摘要