Regularized Hypothesis-Induced Wasserstein Divergence for unsupervised domain adaptation
KNOWLEDGE-BASED SYSTEMS(2024)
摘要
Unsupervised domain adaptation (UDA) aims to align the data distributions of source and target domains to learn domain -invariant feature representations. The performance of such representations heavily relies on the measurement of the discrepancy between the distributions. Recent UDA methods utilize hypothesis -based measurements, also known as classifier -induced divergences, to quantify the differences between the two domain distributions, for instance, H Delta H-divergence. Nevertheless, classifier -induced divergences face several challenges, including the fake covariate alignment problem and the inconsistency problem, rendering them inadequate for discerning dissimilarities between different categories of output by the classifier. This paper proposes a novel method called Regularized Hypothesis -Induced Wasserstein Divergence -Based Representation Learning for Unsupervised Domain Adaptation (RHWD) to address these issues. Specifically, RHWD introduces a new hypothesis -induced Wasserstein divergence (HWD) to effectively quantify the differences between the two distributions, aiming to overcome the fake covariate alignment problem. Furthermore, RHWD includes a consistency regularization term to mitigate the inconsistency problem. We train RHWD adversarially in three iterative steps. Additionally, we provide empirical evaluations on standard benchmarks, demonstrating the effectiveness of our proposed RHWD.
更多查看译文
关键词
Wasserstein hypothesis,Representation learning,Unsupervised domain adaptation,Generalized sliced Wasserstein distance
AI 理解论文
溯源树
样例
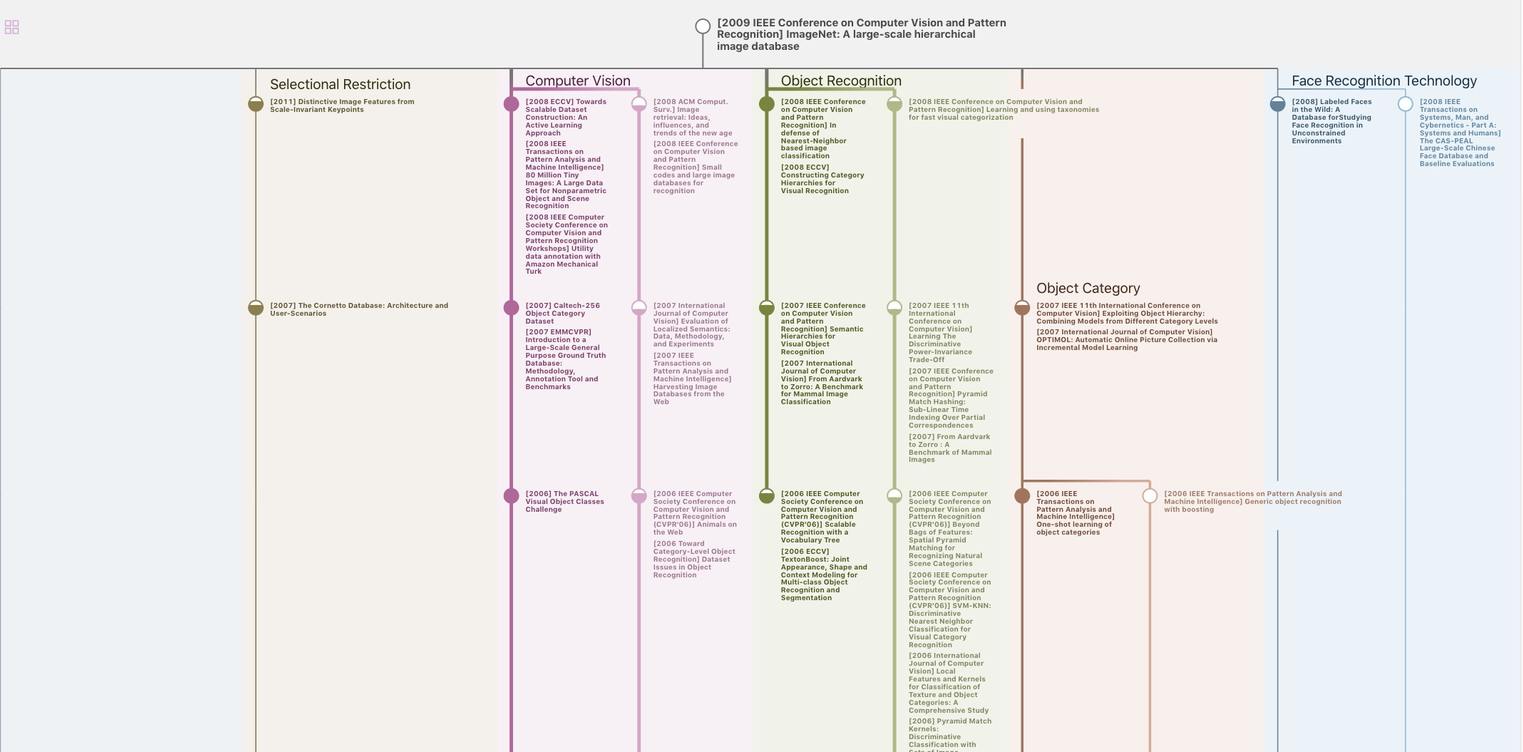
生成溯源树,研究论文发展脉络
Chat Paper
正在生成论文摘要