Unveiling consumer preferences in automotive reviews through aspect-based opinion generation
JOURNAL OF RETAILING AND CONSUMER SERVICES(2024)
摘要
Unveiling consumer preferences in online reviews is receiving increasing attention. While most existing ap-proaches for consumer preferences have achieved significant improvements, fine-grained sentiment is rarely considered. Fine-grained sentiment analysis involves several essential tasks, such as aspect-opinion recognition, and sentiment orientation analysis. However, existing methods cannot effectively generate an opinion pair, especially when dealing with Chinese automotive reviews. In this paper, we propose a joint course-and fine-grained sentiment analysis of preferences, a new framework for opinion pair generation using graph neural networks (GCN), which optimizes model performance based on aspect-wise sentiment information, as well as our experiments on the course-and fine-grained tasks. Our graph-based multi-grained convolution (CMGC) model outperforms all baselines by at least 1% accuracy in coarse-grained tasks. The results in the fine-grained task are significantly better than the baseline, surpassing the previous state-of-the-art by 1.33% and 3.88% in R and R@1, respectively. Our results can effectively reveal consumer preferences from automotive reviews, which provides business managers with specific marketing strategies.
更多查看译文
关键词
Consumer preference,Fine-grained sentiment analysis,Deep learning,Chinese automotive reviews
AI 理解论文
溯源树
样例
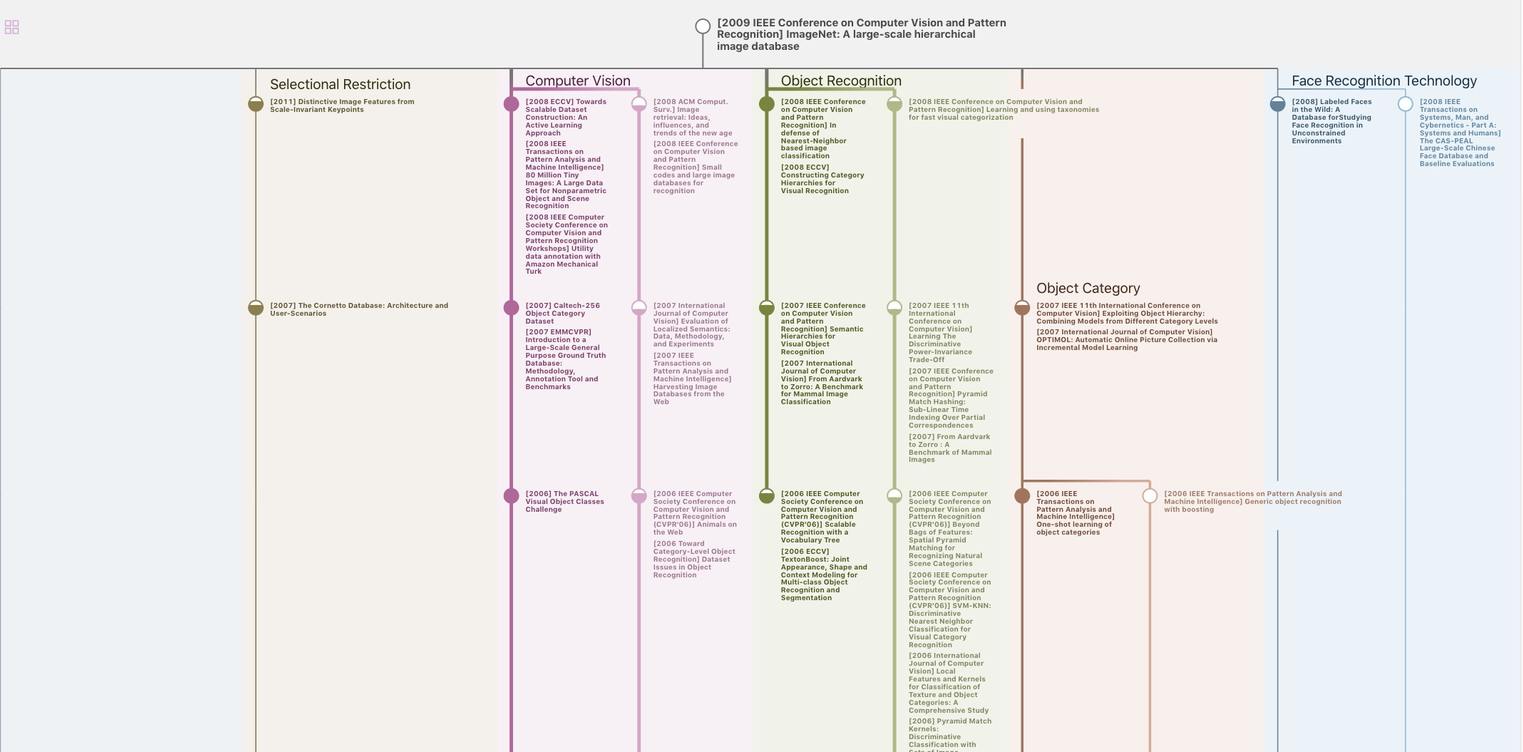
生成溯源树,研究论文发展脉络
Chat Paper
正在生成论文摘要