Neural network-based energy signatures for non-intrusive energy audit of buildings: Methodological approach and a real-world application
SUSTAINABLE ENERGY GRIDS & NETWORKS(2023)
摘要
Energy Signatures (ES) are highly informative gray-box regression models. Thanks to their simplicity and interpretability, to their data-driven approach, and to their effectiveness in describing a building response to external weather variables, they are employed for (i) the determination of the Balance Point (BP) temperature of a building, (ii) the ranking of the efficiency of heating or cooling systems, (iii) the provision of building diagnostic information, and (iv) the development of strategies for more energy-efficient buildings and the estimation of potential savings. In this work, we propose an innovative energy audit tool, based on a Feed Forward Neural Network (NN) to determine ES from aggregated (meter-level) electric load profiles of buildings. Multiple NN-based regression models are defined for each building and compared to provide the most accurate and informative one, by considering proper fit and significance indexes. This allows the eventual existence of multiple cooling regimes to be detected. Moreover, the energy audit methodology defines and applies an innovative Key Performance Indicator (KPI), called Temperature Unstandardized Beta Weight (beta(Temp)*), to account not only for the thermal behavior of buildings but also for the efficiency of the conditioning system and the internal heat generation. This ES approach has been applied to a dataset of electric consumption patterns from about eighty industrial buildings from a telecommunication (TLC) service provider in Italy. The useful outputs from the proposed methodology, together with its simplicity, effectiveness and applicability, are intended to support the diffused understanding of the thermal behavior of buildings and the analysis of their inefficiencies, in order to enhance energy retrofit actions and reduce consumption.
更多查看译文
关键词
Building energy efficiency,Energy audit,Energy signature,Neural network
AI 理解论文
溯源树
样例
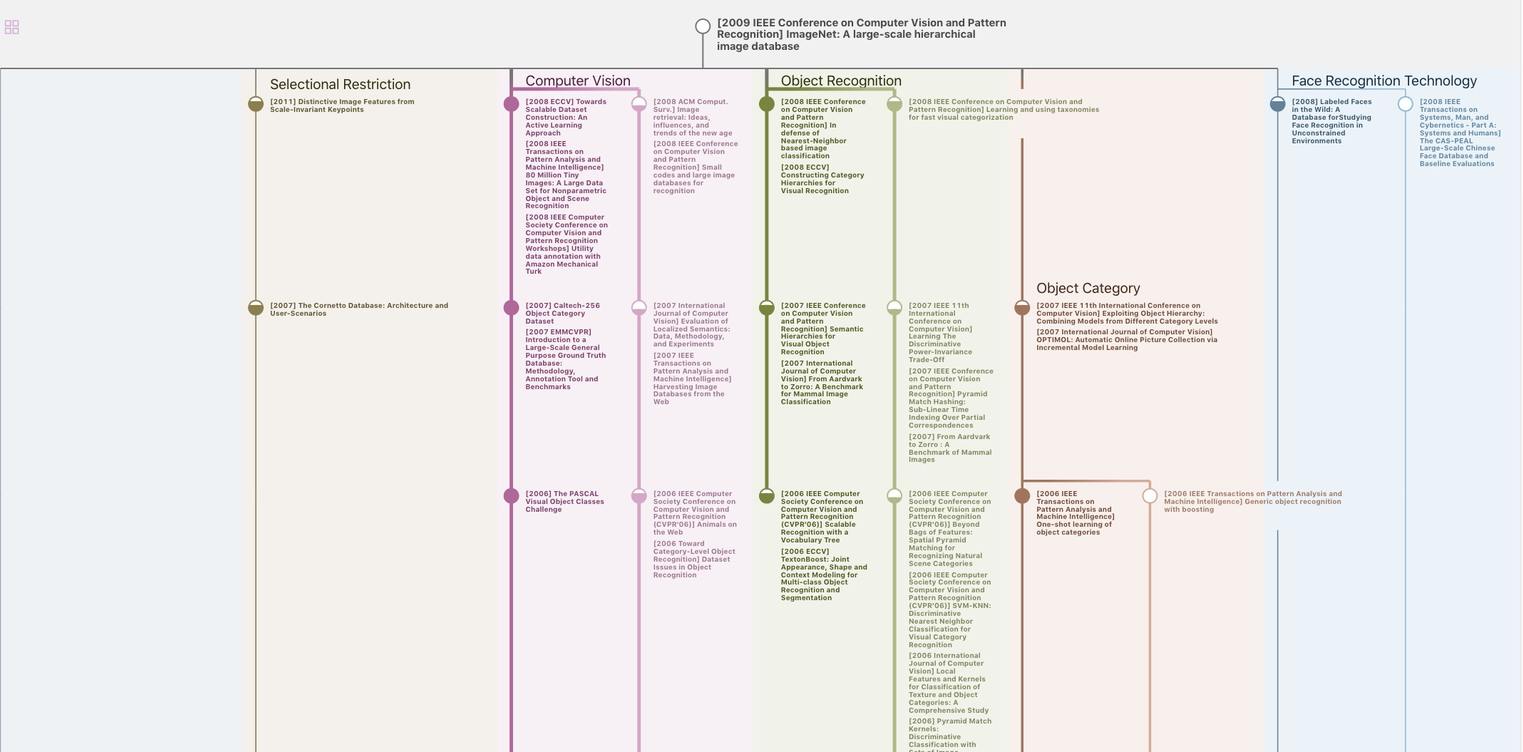
生成溯源树,研究论文发展脉络
Chat Paper
正在生成论文摘要