PESI: Personalized Explanation recommendation with Sentiment Inconsistency between ratings and reviews
KNOWLEDGE-BASED SYSTEMS(2024)
摘要
Explainable recommendations aim to generate personalized explanations for suggested items, which are provided based on the historical interactions (e.g., ratings) between users and items. Review contents are often taken as a proxy of explanations. However, most review-based models presume a sentiment consistency between user ratings and review contents, ignoring their inconsistency in real applications. By analyzing three real datasets, we observe that a user may share a positive (negative) opinion to an item in terms of rating value but a negative (positive) sentiment in terms of review content, and such contradicting scenario takes over 40% of all cases in general. To resolve this issue, in this paper we propose a novel explainable recommendation model called PESI, which can generate accurate Personalized Explanations recommendation with the involvement of Sentiment Inconsistency between ratings and reviews. Specifically, PESI consists of three modules: rating prediction, explanation generation, and a novel rating-review inconsistency extraction. The inconsistency extraction module disentangles ratings and reviews, effectively distinguishing both shared and private features, and ensuring accurate disentanglement through contrastive learning objectives. Then, the extracted inconsistent features are injected into the explanation generation module to provide more personalized and higher-quality explanations. The experimental results on the three datasets show that PESI consistently outperforms other competing methods in terms of explanation quality.
更多查看译文
关键词
Recommendation system,Explanation generation,Sentiment analysis
AI 理解论文
溯源树
样例
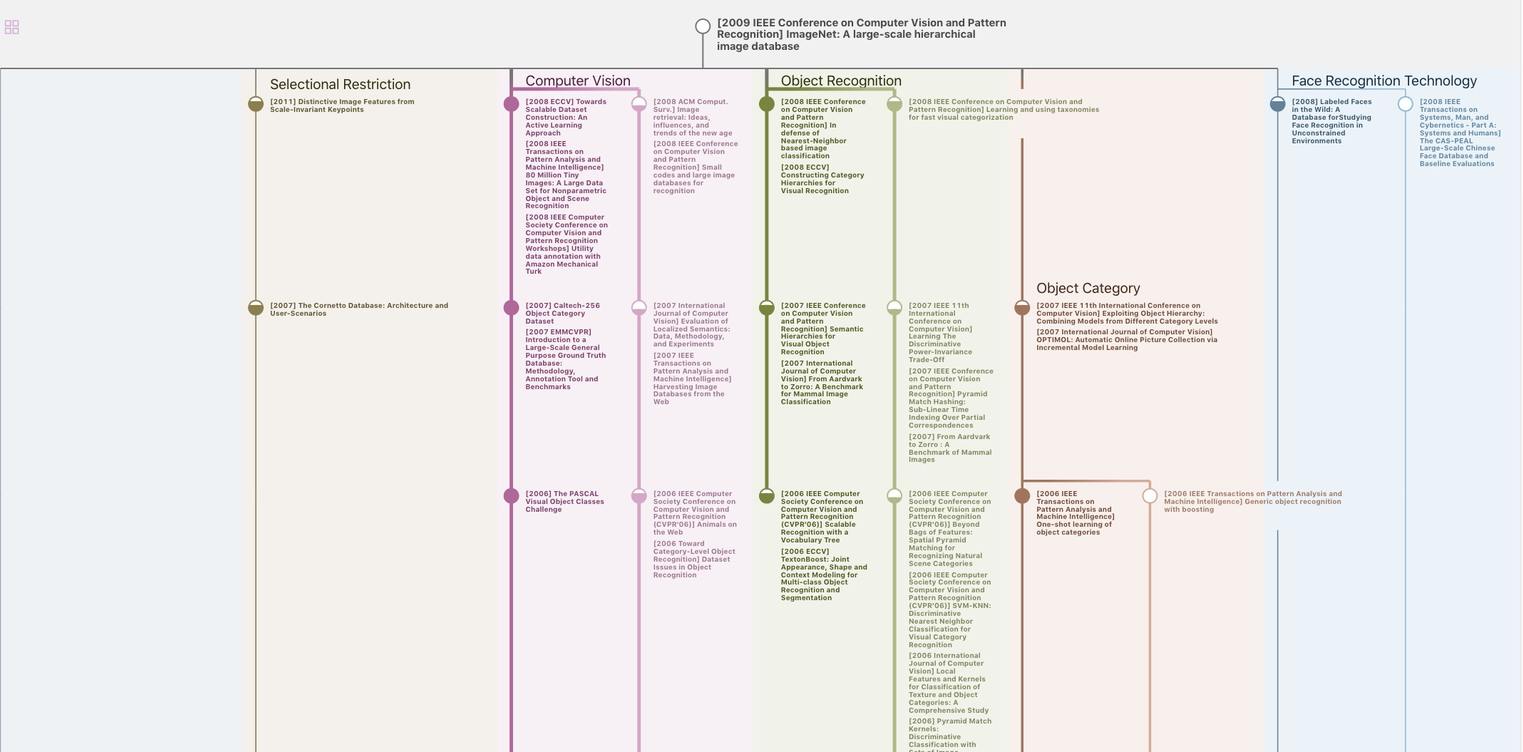
生成溯源树,研究论文发展脉络
Chat Paper
正在生成论文摘要