Mix-supervised multiset learning for cancer prognosis analysis with high-censoring survival data
EXPERT SYSTEMS WITH APPLICATIONS(2024)
摘要
High censoring phenomenon usually occurs in cancer prognosis analysis, which, however, would introduce bias for model construction and limit generalization performance. In this paper, we first explore and identify an appropriate censoring range for cancer prognosis evaluation, upon which we present a mix-supervised multiset learning framework to cope with high-censoring data. Specifically, we construct multiple subsets with the specified censoring proportion, followed by a multiset representation learning method to learn subset-specific representations, which equips with adversary integrality preservation and dependency limitation constraints to ensure the unbiasedness of subsets and eliminate the redundancy among subsets, respectively. Furthermore, a mix-supervised multiset fusion model is proposed to estimate the relative survival risk, in which teacher model can make full use of the survival time of uncensored samples and the prognosis-related attributes of censored ones to generate reliable pseudo-labels and latent-space for student model. We evaluate the proposed method on three public datasets, and extensive experimental results demonstrate its superiority.
更多查看译文
关键词
High-censoring data,Multiset representation learning,Mix-supervised,Prognosis analysis
AI 理解论文
溯源树
样例
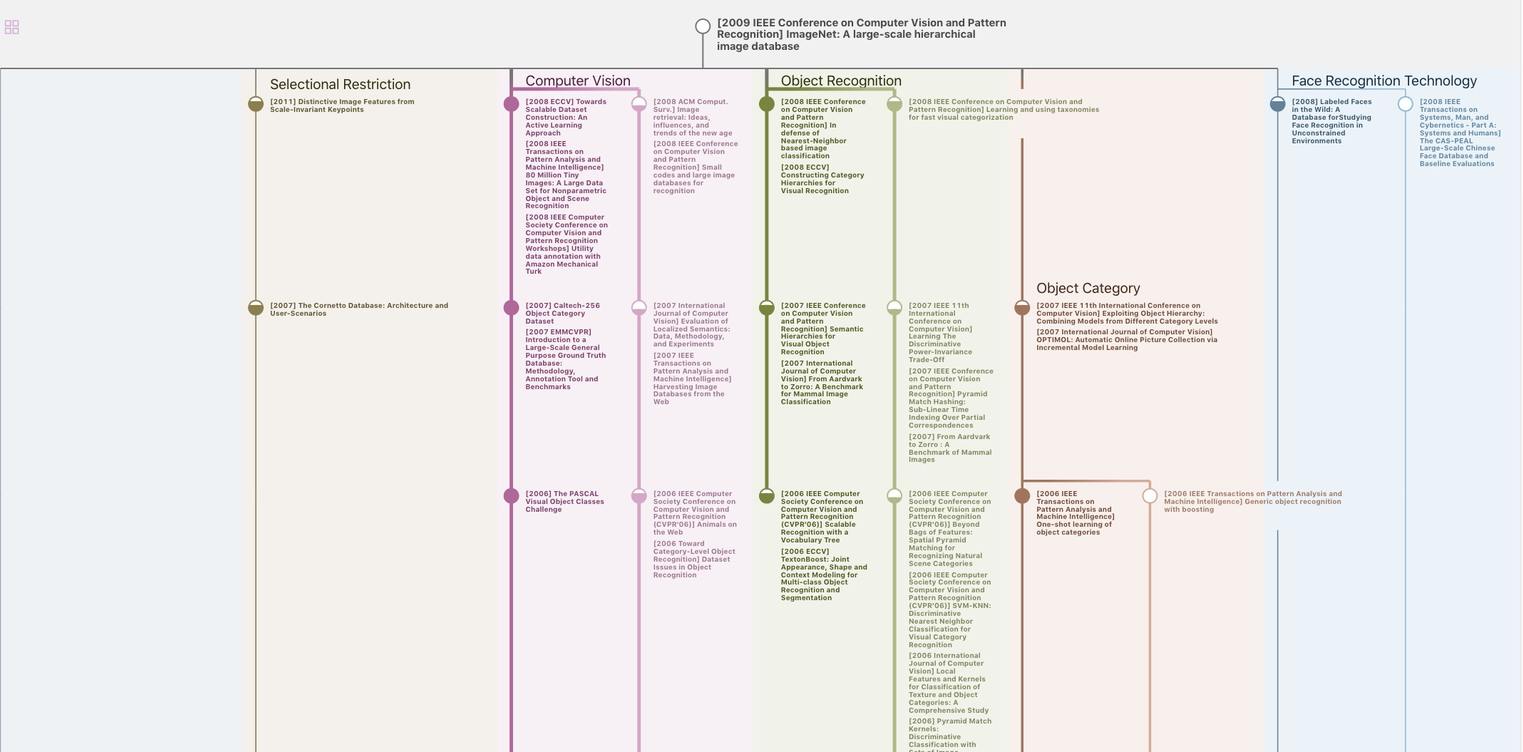
生成溯源树,研究论文发展脉络
Chat Paper
正在生成论文摘要