Mapping cropland rice residue cover using a radiative transfer model and deep learning
COMPUTERS AND ELECTRONICS IN AGRICULTURE(2023)
摘要
Accurate determination of rice residue cover (RRC) can improve the monitoring of tillage information. Currently, the accurate determination of RRC using optical remote sensing is hindered by variations in cropland moisture and cover of following crops. The fractional cover (FC) of the soil (fS), crop (fC), and crop residue (fCR) changes (fS + fC + fCR = 1) after the following crop is planted, which increases the difficulty of remote-sensing RRC estimation. Cropland soil moisture and crop residue moisture affect the values of cropland and crop residue spectral indices (CRSIs), thereby reducing the accuracy of remote-sensing RRC estimation. Deep learning techniques (e.g., convolutional neural networks [CNN] and transfer learning [TL]) have been proven to extract the deep features of input images with distortion invariance, such as displacement and scaling, which are similar to moisture and the following crop effects on remote-sensing CRSIs. This study aimed to evaluate the combined use of deep features of cropland spectra extracted by deep learning techniques to estimate the cropland RRC under the effects of variations in cropland moisture and cover of the following crops. This study proposes an RRCNet CNN that fuses deep and shallow features to improve RRC estimation. A PROSAIL radiative transfer model was employed to simulate a cropland "soil-crop-crop residue" mixed spectra dataset (n = 103,068), considering the variations in cropland moisture and the cover of the following crop. The RRCNet was first pre-trained using the simulated dataset, and then the knowledge from the pre-trained RRCNet was updated based on field experimental FCs, RRCs, and Sentinel-2 image spectra using the TL technique. Our study indicates that RRCNet can incorporate shallow and deep spectral features of cropland "soil-crop-crop residue" mixed spectra, providing high-performance FCs and RRC estimation. The FCs and RRC estimates from RRCNet + TL (FCs: R2 = 0.939, root mean squared error (RMSE) = 0.071; RRC: R2 = 0.891, RMSE = 0.083) were more accurate than those from CRSI + multiple linear regression, CRSI + random forest, and CRSI + support vector machine (FCs: R2 = 0.877-907, RMSE = 0.086-0.101; RRC: R2 = 0.378-0.714, RMSE = 0.133-0.229). We mapped the multistage RRC based on Sentinel-2 multispectral instrument (MSI) images and RRCNet. Tillage information can be inferred from RRC and RRC difference maps changes.
更多查看译文
关键词
Convolutional neural network,RRC,RTM,Machine learning
AI 理解论文
溯源树
样例
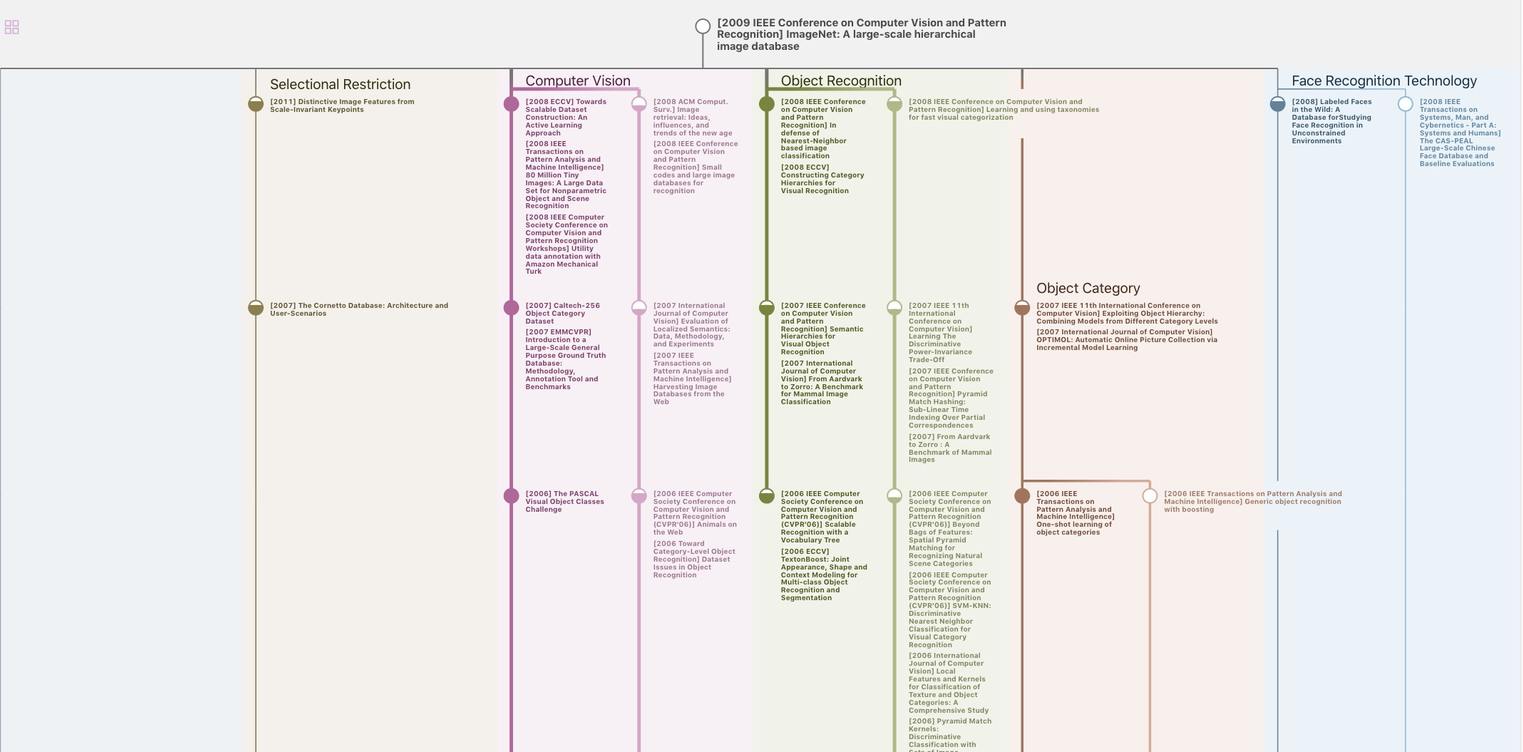
生成溯源树,研究论文发展脉络
Chat Paper
正在生成论文摘要