Enhancing prediction accuracy for LOCA break sizes in nuclear power plants: A hybrid deep learning method with data augmentation and hyperparameter optimization
ANNALS OF NUCLEAR ENERGY(2024)
摘要
In order to accurately predict the break size of loss of coolant accident, this study presents a novel break size prediction method based on a hybrid deep learning model that leverages data augmentation and optimization. This approach utilizes an interpolation method to augment limited sample data. By integrating Convolutional Neural Networks (CNN) and Long Short-Term Memory (LSTM) networks within the hybrid deep learning architecture, accurate extraction of both spatial and temporal information from the data is achieved. The hyperparameters of the hybrid model are optimized using an improved particle swarm optimization (IPSO) algorithm. Furthermore, a comparative analysis between the proposed algorithm and traditional methods is conducted, employing four out-of-sample data derived from novel test cases to evaluate the model's prediction accuracy. The results demonstrate that the proposed method can achieve high-precision deep learning modeling and effectively determine the break size of loss of coolant accident.
更多查看译文
关键词
Loss of Coolant Accident (LOCA),Hybrid Deep Learning,Convolutional Neural Networks (CNN),Long Short-Term Memory (LSTM) Networks,Improved Particle Swarm Optimization (IPSO),Data Augmentation in Nuclear Accidents
AI 理解论文
溯源树
样例
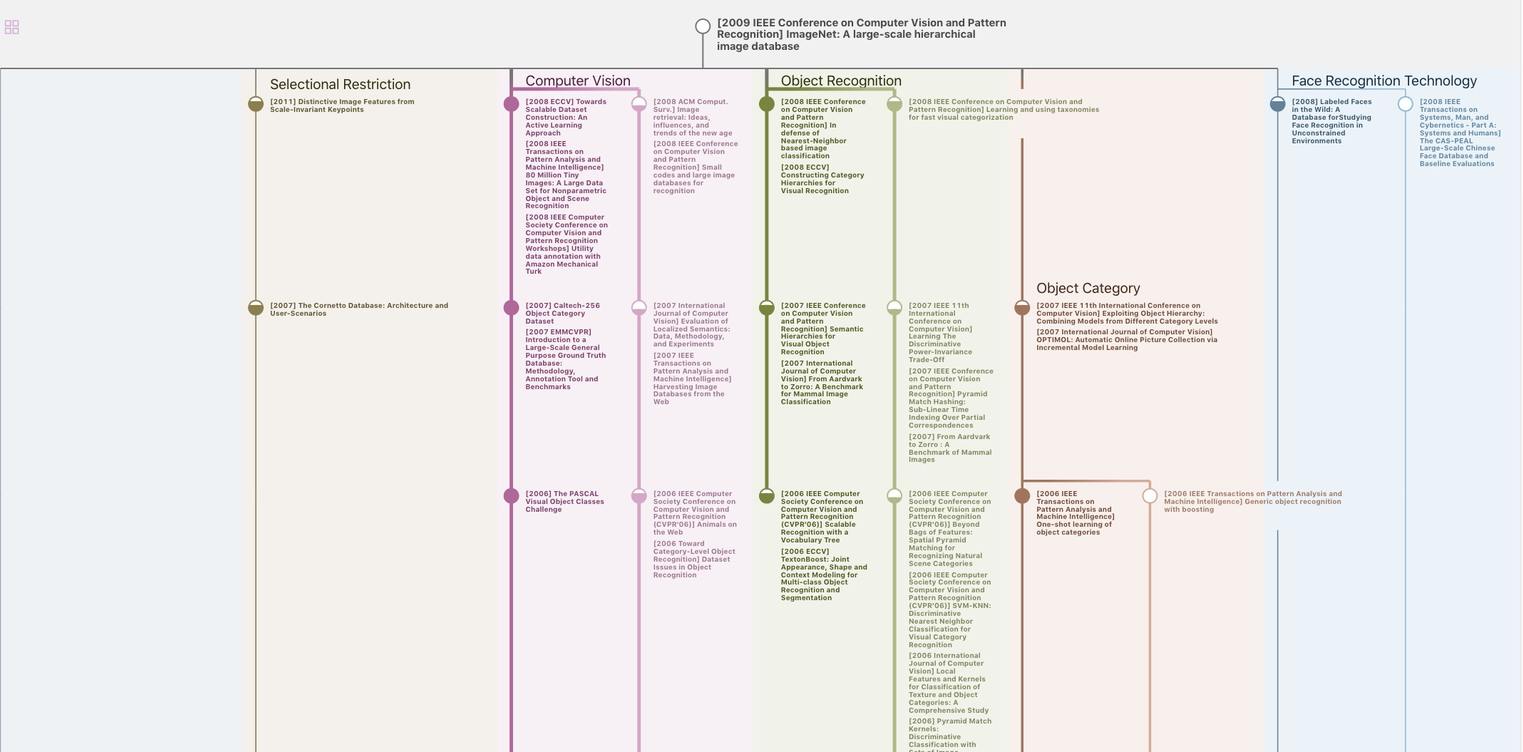
生成溯源树,研究论文发展脉络
Chat Paper
正在生成论文摘要