ARIES: Accelerating Distributed Training in Chiplet-based Systems via Flexible Interconnects
2023 IEEE/ACM INTERNATIONAL CONFERENCE ON COMPUTER AIDED DESIGN, ICCAD(2023)
摘要
Large-scale deep learning models are widely deployed in many application domains with remarkable performance improvements. However, training these models with immense parameters calls for unprecedented computing and communication capabilities. Recently, chiplet-based architectures have shown much promise in scaling Deep Neural Network (DNN) inference, but their applications in the training phase remain unexplored and challenging. In this paper, we posit, beyond scaling computing capability, chiplet-based architectures could also be leveraged to enable new optimization opportunities for existing parallel training algorithms (e.g., Ring and Tree-based all-reduce). Specifically, we aim to explore a variety of topological characteristics, along with the interposer technology, to sustain the performance scaling of parallel training in chiplet-based systems. We propose ARIES, a versatile chiplet-based communication architecture supporting various parallel training algorithms using a flexible interconnect design. The proposed design can adapt to various collective operations such as reduce and gather across a wide diversity of training algorithms. Moreover, such flexibility is also leveraged to further enhance existing all-reduce algorithms depending on the latency and bandwidth requirements of the DNN model and dataset size. Simulation results show that the proposed ARIES can achieve up to 3.92x speedup in execution time and 38.8% reduction in Network-on-Chip (NoC) energy consumption when compared to prior work.
更多查看译文
关键词
DNNs,parallel training,chiplets,collective operations
AI 理解论文
溯源树
样例
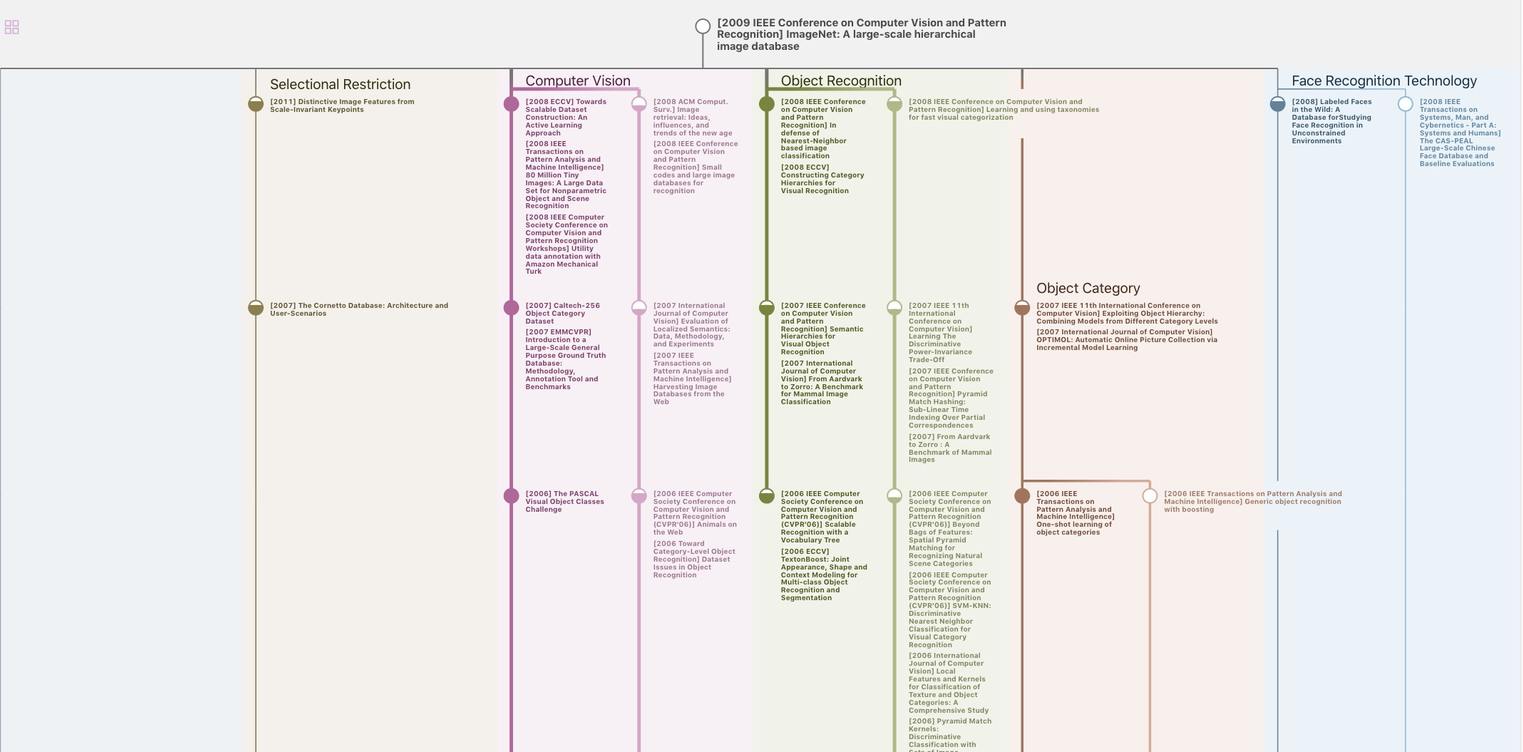
生成溯源树,研究论文发展脉络
Chat Paper
正在生成论文摘要